Crowd-Sensing Enhanced Parking Patrol Using Sharing Bikes’ Trajectories
IEEE Transactions on Knowledge and Data Engineering(2023)
摘要
Illegal vehicle parking is a common urban problem faced by major cities in the world, as it incurs traffic jams, which lead to air pollution and traffic accidents. The government highly relies on active human efforts to detect illegal parking events. However, such an approach is extremely ineffective to cover a large city since the police have to patrol over the entire city roads. The massive and high-quality sharing bike trajectories from Mobike offer us a unique opportunity to design a ubiquitous illegal parking detection approach, as most of the illegal parking events happen at curbsides and have significant impact on the bike users. The detection result can guide the patrol schedule, i.e., send the patrol policemen to the region with higher illegal parking risks, and further improve the patrol efficiency. Inspired by this idea, three main components are employed in the proposed framework: 1)
trajectory pre-processing
, which filters outlier GPS points, performs map-matching, and builds trajectory indexes; 2)
illegal parking detection
, which models the normal trajectories, extracts features from the evaluation trajectories, and utilizes a distribution test-based method to discover the illegal parking events; and 3)
patrol scheduling
, which leverages the detection result as reference context, and models the scheduling task as a multi-agent reinforcement learning problem to guide the patrol police. Finally, extensive experiments are presented to validate the effectiveness of illegal parking detection, as well as the improvement of patrol efficiency.
更多查看译文
关键词
Trajectory data mining,urban computing,crowd-sensing,reinforcement learning
AI 理解论文
溯源树
样例
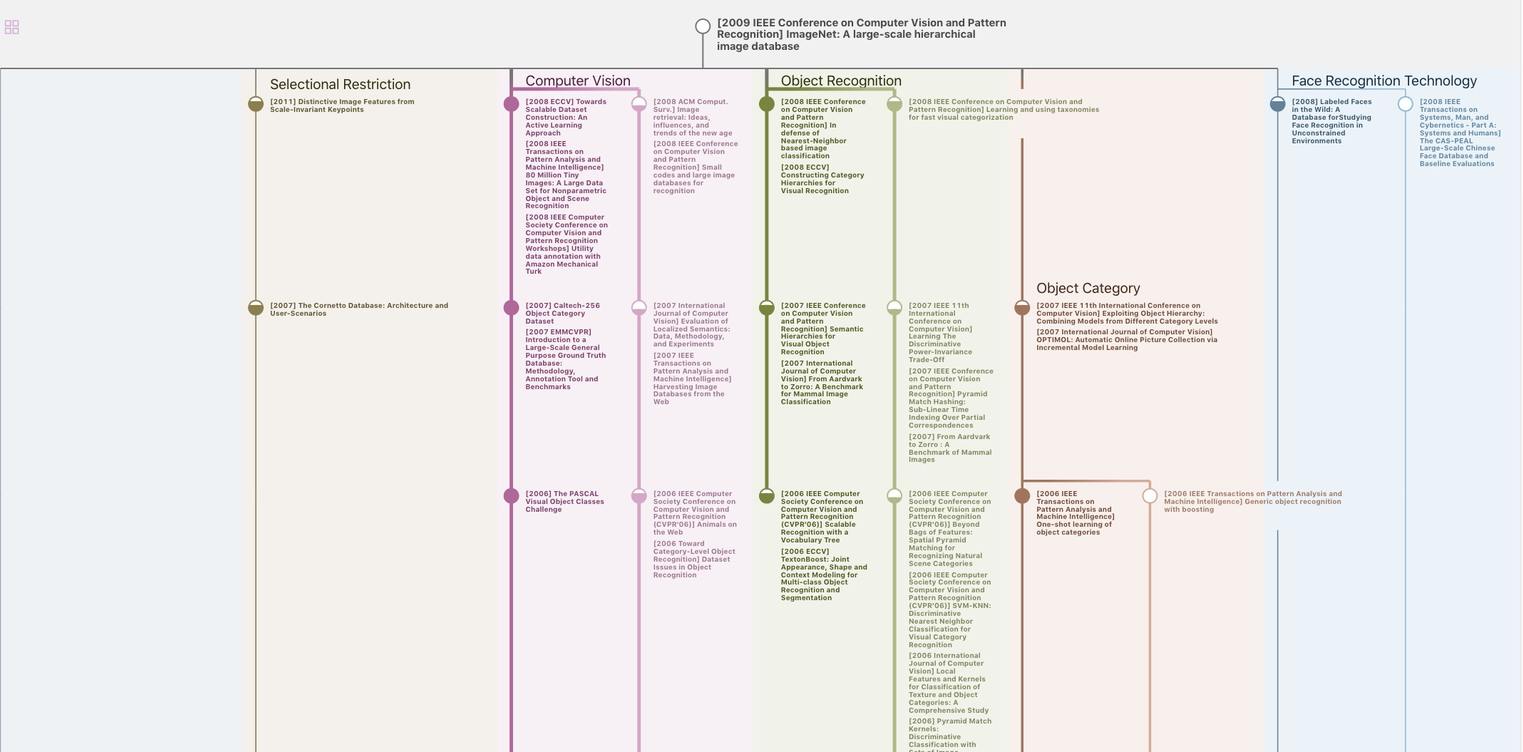
生成溯源树,研究论文发展脉络
Chat Paper
正在生成论文摘要