Numerical Optimization of a Nanophotonic Cavity by Machine Learning for Near-Unity Photon Indistinguishability at Room Temperature
arxiv(2021)
摘要
Room-temperature (RT), on-chip deterministic generation of indistinguishable photons coupled to photonic integrated circuits is key for quantum photonic applications. Nevertheless, high indistinguishability (I) at RT is difficult to obtain due to the intrinsic dephasing of most deterministic single-photon sources (SPS). Here, we present a numerical demon-stration of the design and optimization of a hybrid slot-Bragg nanophotonic cavity that achieves a theoretical near-unity I and a high coupling efficiency (beta) at RT for a variety of single-photon emitters. Our numerical simulations predict modal volumes in the order of 10(-3)(lambda/2n)(3), allowing for strong coupling of quantum photonic emitters that can be heterogeneously integrated. We show that high I and beta should be possible by fine-tuning the quality factor (Q) depending on the intrinsic properties of the single-photon emitter. Furthermore, we perform a machine learning optimization based on the combination of a deep neural network and a genetic algorithm (GA) to further decrease the modal volume by almost 3 times while relaxing the tight dimensions of the slot width required for strong coupling. The optimized device has a slot width of 20 nm. The design requires fabrication resolution in the limit of the current state-of-the-art technology. Also, the condition for high I and beta requires a positioning accuracy of the quantum emitter at the nanometer level. Although the proposal is not a scalable technology, it can be suitable for experimental demonstration of single-photon operation.
更多查看译文
关键词
single-photon,neural network,genetic algorithm,nanophotonics,nanocavity
AI 理解论文
溯源树
样例
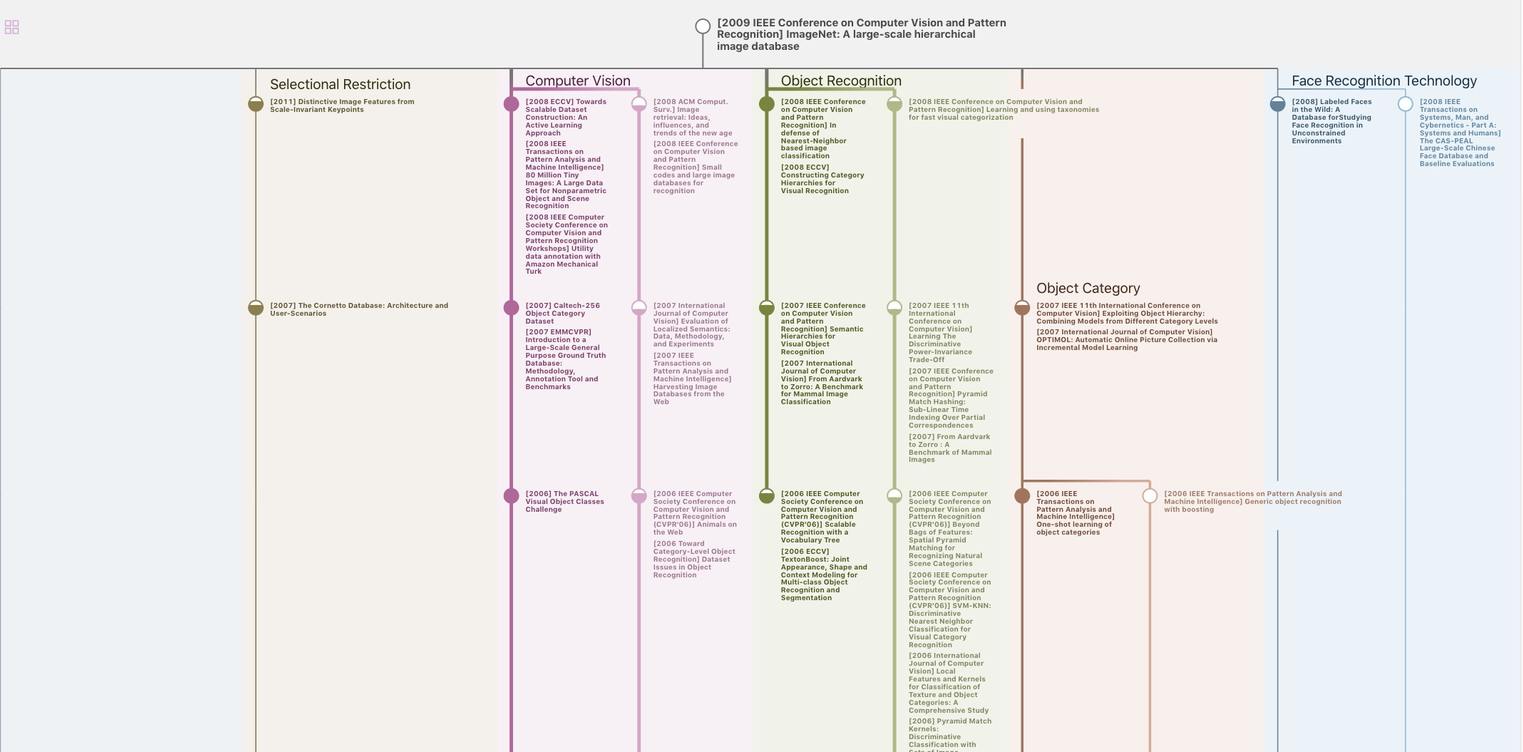
生成溯源树,研究论文发展脉络
Chat Paper
正在生成论文摘要