A Survey of Self-Supervised and Few-Shot Object Detection
IEEE Transactions on Pattern Analysis and Machine Intelligence(2023)
摘要
Labeling data is often expensive and time-consuming, especially for tasks such as object detection and instance segmentation, which require dense labeling of the image. While few-shot object detection is about training a model on
novel
(unseen) object classes with little data, it still requires prior training on many labeled examples of
base
(seen) classes. On the other hand, self-supervised methods aim at learning representations from unlabeled data which transfer well to downstream tasks such as object detection. Combining few-shot and self-supervised object detection is a promising research direction. In this survey, we review and characterize the most recent approaches on few-shot and self-supervised object detection. Then, we give our main takeaways and discuss future research directions. Project page:
https://gabrielhuang.github.io/fsod-survey/
.
更多查看译文
关键词
Self-supervised,few-shot,low-data,object detection,instance segmentation
AI 理解论文
溯源树
样例
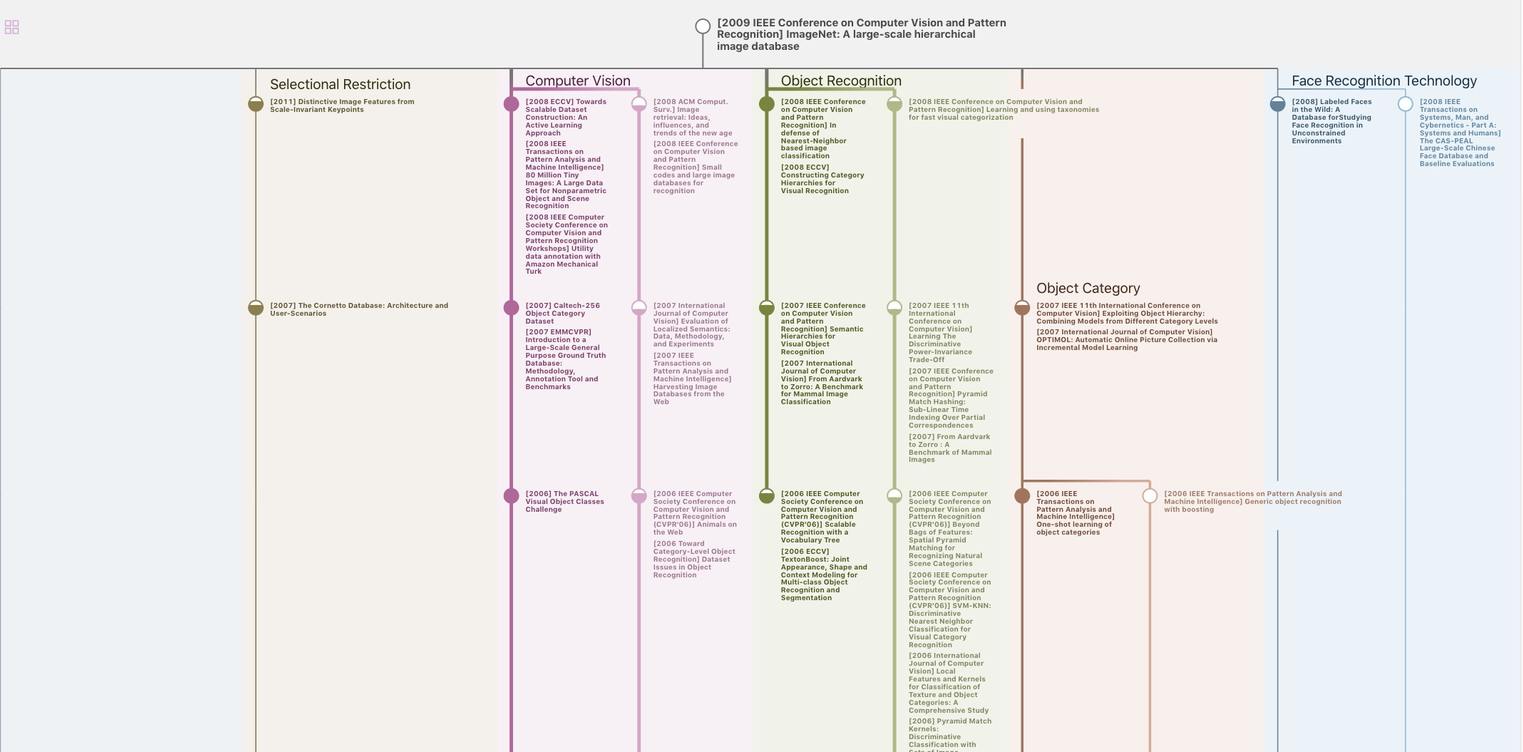
生成溯源树,研究论文发展脉络
Chat Paper
正在生成论文摘要