Online Discriminative Semantic-Preserving Hashing for Large-Scale Cross-Modal Retrieval.
PRICAI(2021)
摘要
Cross-modal hashing has drawn increasing attentions for efficient retrieval across different modalities, and existing methods primarily learn the hash functions in a batch based mode, i.e., offline methods. Nevertheless, the multimedia data often comes in a streaming fashion, which makes the batch based learning methods uncompetitive for large-scale streaming data due to the large memory consumption and calculation. To address this problem, we present an Online Discriminative Semantic-Preserving Hashing (ODSPH) method for large-scale cross-modal retrieval, featuring on fast training speed, low memory consumption and high retrieval accuracy. Within the proposed ODSPH framework, we utilize the newly coming data points to learn the hash codes and update hash functions in a stream manner. When new data comes, the corresponding hash codes are obtained by regressing the class label of the training examples. For hash function, we update it with the accumulated information from each round. Besides, we design a novel momentum updating method to adaptively update the hash function and reduce quantization loss, which can produce discriminative hash codes for high retrieval precision. Extensive experiments on three benchmark datasets show that the proposed ODSPH method improves the retrieval performance over the state-of-the-arts.
更多查看译文
关键词
semantic-preserving,large-scale,cross-modal
AI 理解论文
溯源树
样例
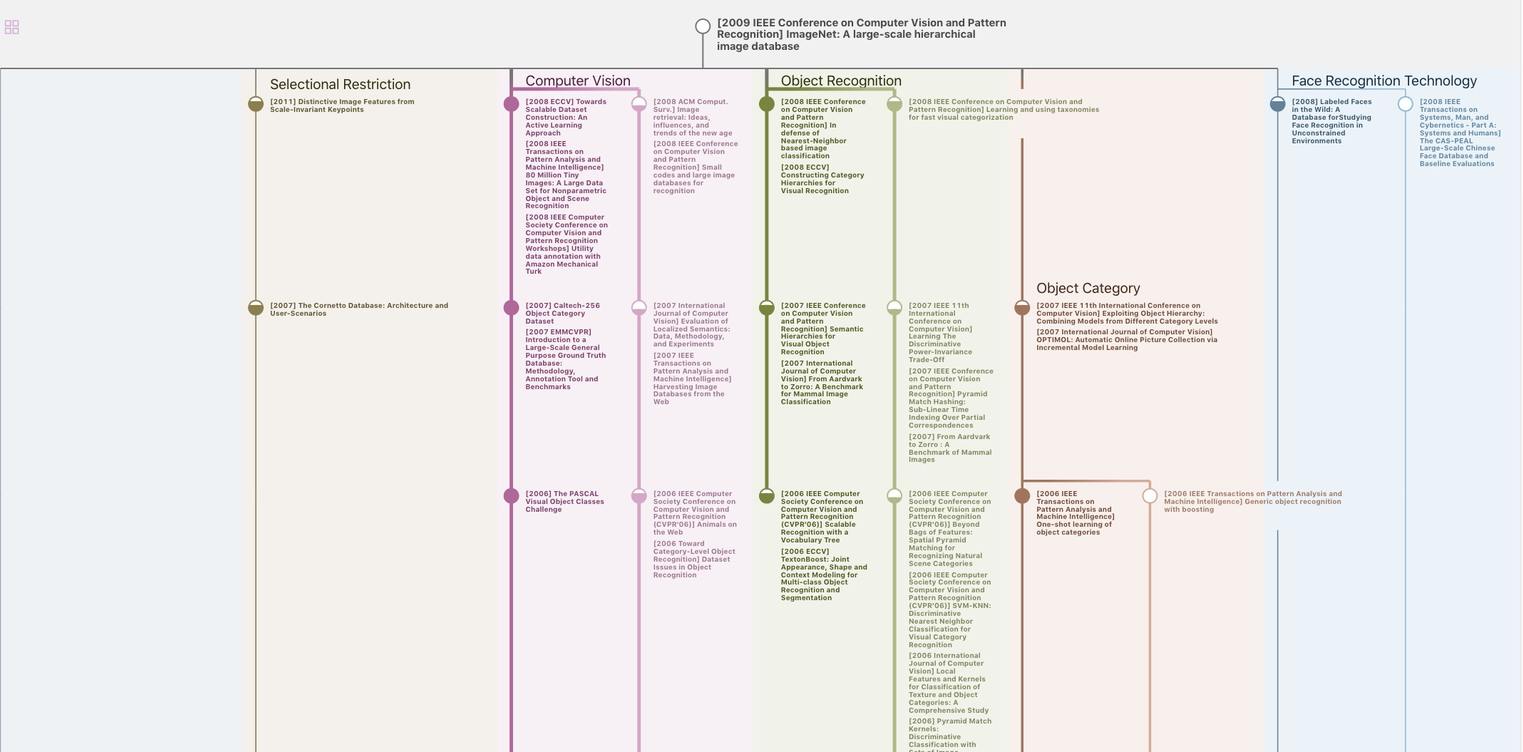
生成溯源树,研究论文发展脉络
Chat Paper
正在生成论文摘要