CNOS: A Strong Baseline for CAD-based Novel Object Segmentation
2023 IEEE/CVF INTERNATIONAL CONFERENCE ON COMPUTER VISION WORKSHOPS, ICCVW(2023)
摘要
We propose a simple yet powerful method to segment novel objects in RGB images from their CAD models. Leveraging recent foundation models, Segment Anything and DINOv2, we generate segmentation proposals in the input image and match them against object templates that are pre-rendered using the CAD models. The matching is realized by comparing DINOv2 cls tokens of the proposed regions and the templates. The output of the method is a set of segmentation masks associated with per-object confidences defined by the matching scores. We experimentally demonstrate that the proposed method achieves state-of-the-art results in CAD-based novel object segmentation on the seven core datasets of the BOP challenge, surpassing the recent method of Chen et al. by absolute 19.8% AP.
更多查看译文
AI 理解论文
溯源树
样例
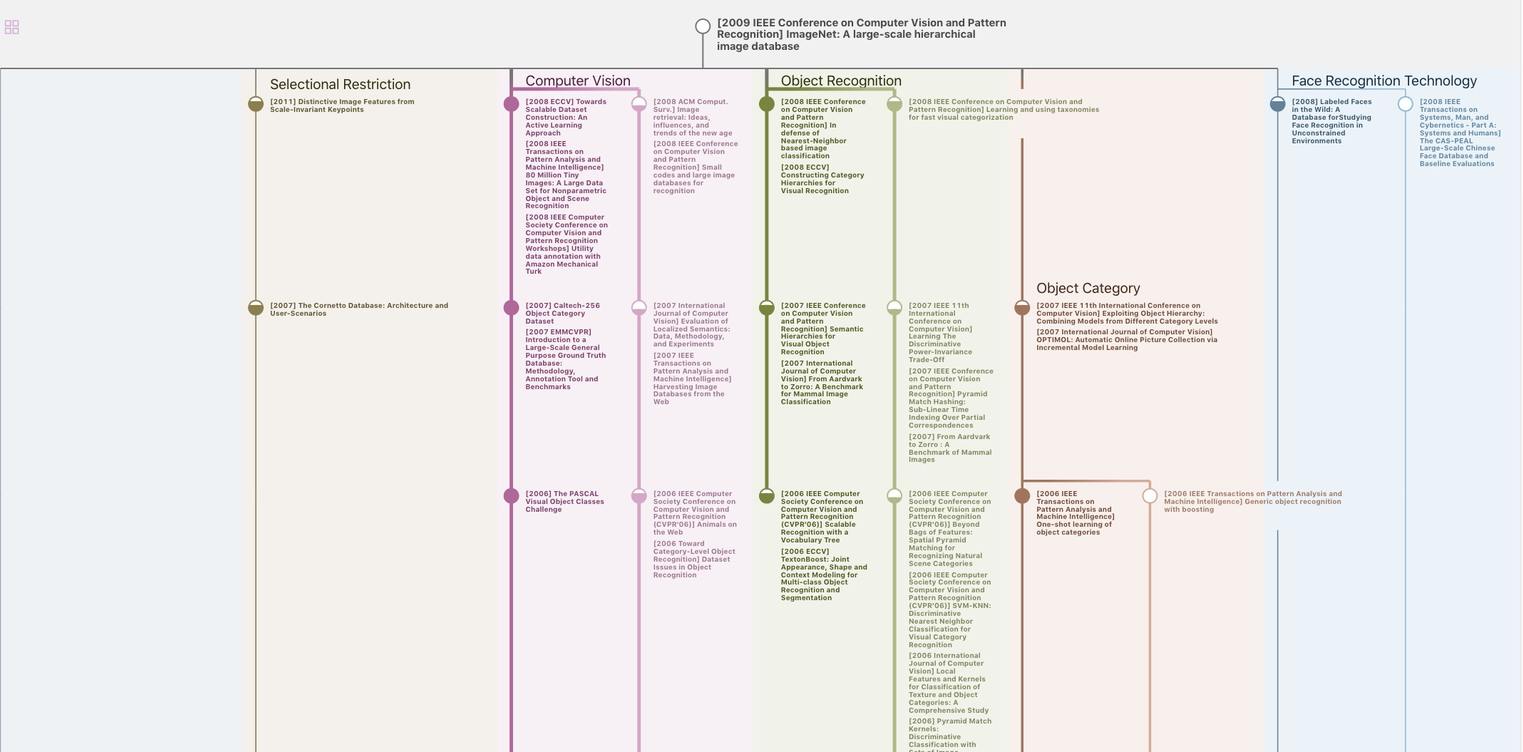
生成溯源树,研究论文发展脉络
Chat Paper
正在生成论文摘要