Improving GI Toxicity Models Through Deep Learning-Based Segmentation and Biomechanical Model-Based Dose Accumulation
INTERNATIONAL JOURNAL OF RADIATION ONCOLOGY BIOLOGY PHYSICS(2021)
摘要
PURPOSE/OBJECTIVE(S) Current GI toxicity models are limited by the uncertainty in the estimated delivered dose used to develop the models. The purpose of this study is to assess the benefit of harnessing deep learning and biomechanical models to improve the understanding of the dose-toxicity relationship. MATERIALS/METHODS Retrospective dose accumulation was conducted on 75 patients with primary and metastatic liver cancer treated with external beam radiotherapy guided by daily CT-on-rails (CTOR), based on a novel deformable image registration (DIR) approach that applies biomechanical model-based and intensity-based DIR inside and outside the liver, respectively. The liver was auto-segmented on all images using a clinically validated 2D deep learning model. On a sub-cohort, the combination of stomach and duodenum region of interest (GI ROI) was auto-contoured using a 3D UNet model trained independently on 102 patients. These contours were compared to physician-drawn contours on CTOR, using Dice similarity coefficient (DSC), mean distance to agreement (DTA), Hausdorff distance (HD), and planned dose metrics. Intra-observer variability was evaluated. Doses were converted to equivalent dose in 2Gy fractions (EQD2) for analysis (α/β = 3.5). Differences between planned and delivered dose were calculated, as well as GI ROI daily dose variation. Toxicity probability was calculated based on previously developed accumulated dose normal tissue complication probability (NTCP) models for duodenum and stomach. RESULTS Average (SD) DSC, DTA, and HD between the deep learning model and physician-drawn GI ROI were 0.9 (0.1), 0.2 (0.1) cm, and 2.4 (2.2) cm; intra-observer values were 0.9 (0.0), 0.1 (0.0) cm, and 2.3 (0.8) cm, P > 0.1. Average differences in volume (SD), D50 (SD), and D1 (SD) between the AI model and physician-drawn contours were 25.0 (36.7) cc, 0.6 (1.1) Gy, and 0.5 (0.2) Gy; intra-observer values were 7.1 (4.5) cc, 0.2 (0.3) Gy and 0.3 (0.3) Gy, P > 0.1. Average planned and accumulated D1 (EQD2) to the GI ROI were 28.1 Gy and 26.6 Gy, respectively. These distributions were significantly different (P < 0.01), with differences ranging from 0.2 Gy to 2.9 Gy. Daily D1 to the GI ROI varied by up to 47% (average 25%). For 38% of patients, relative differences in NTCP based on planned and accumulated dose were greater than 50%. Compared to planned dose, 63% of the patients had lower NTCP with accumulated dose, and 13% had higher NTCP. CONCLUSION Variability between AI-based and physician-drawn stomach and duodenum segmentation was equivalent to intra-observer variability. Accumulated dose showed statistically significant differences from planned dose for the stomach and duodenum leading to meaningful differences in NTCP. The daily variability in the maximum dose varied by an average of +/- 25%, demonstrating the potential to optimize daily fractionation based on anatomical configuration of the day.
更多查看译文
AI 理解论文
溯源树
样例
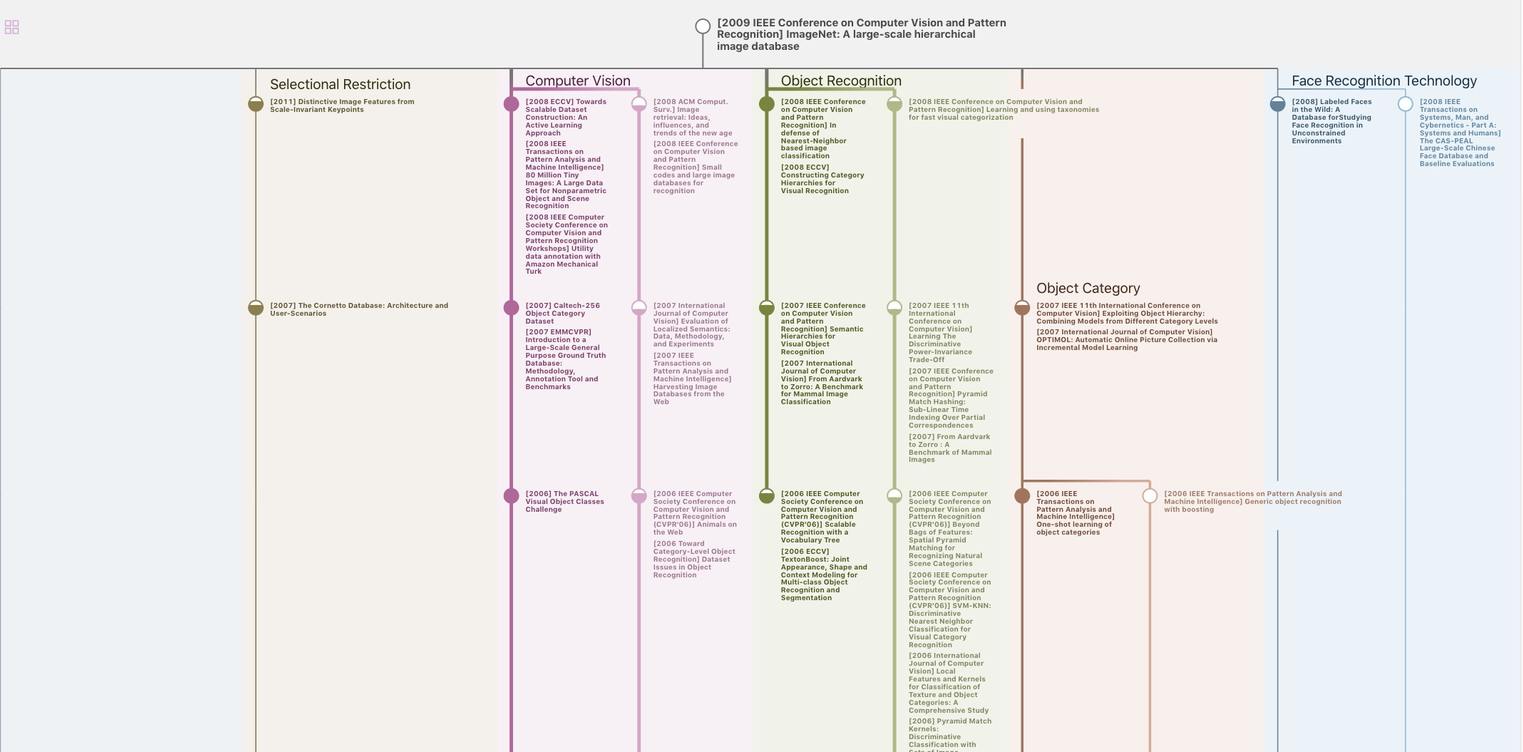
生成溯源树,研究论文发展脉络
Chat Paper
正在生成论文摘要