International Workshop on Continual Semi-Supervised Learning: Introduction, Benchmarks and Baselines
CONTINUAL SEMI-SUPERVISED LEARNING, CSSL 2021(2022)
摘要
The aim of this paper is to formalise a new continual semi-supervised learning (CSSL) paradigm, proposed to the attention of the machine learning community via the IJCAI 2021 International Workshop on Continual Semi-Supervised Learning (CSSL@IJCAI) (https://sites.google.com/view/sscl-workshop-ijcai-2021/), with the aim of raising the field's awareness about this problem and mobilising its effort in this direction. After a formal definition of continual semi-supervised learning and the appropriate training and testing protocols, the paper introduces two new benchmarks specifically designed to assess CSSL on two important computer vision tasks: activity recognition and crowd counting. We describe the Continual Activity Recognition (CAR) and Continual Crowd Counting (CCC) challenges built upon those benchmarks, the baseline models proposed for the challenges, and describe a simple CSSL baseline which consists in applying batch self-training in temporal sessions, for a limited number of rounds. The results show that learning from unlabelled data streams is extremely challenging, and stimulate the search for methods that can encode the dynamics of the data stream.
更多查看译文
关键词
Continual learning, Semi-supervised learning, Artificial intelligence
AI 理解论文
溯源树
样例
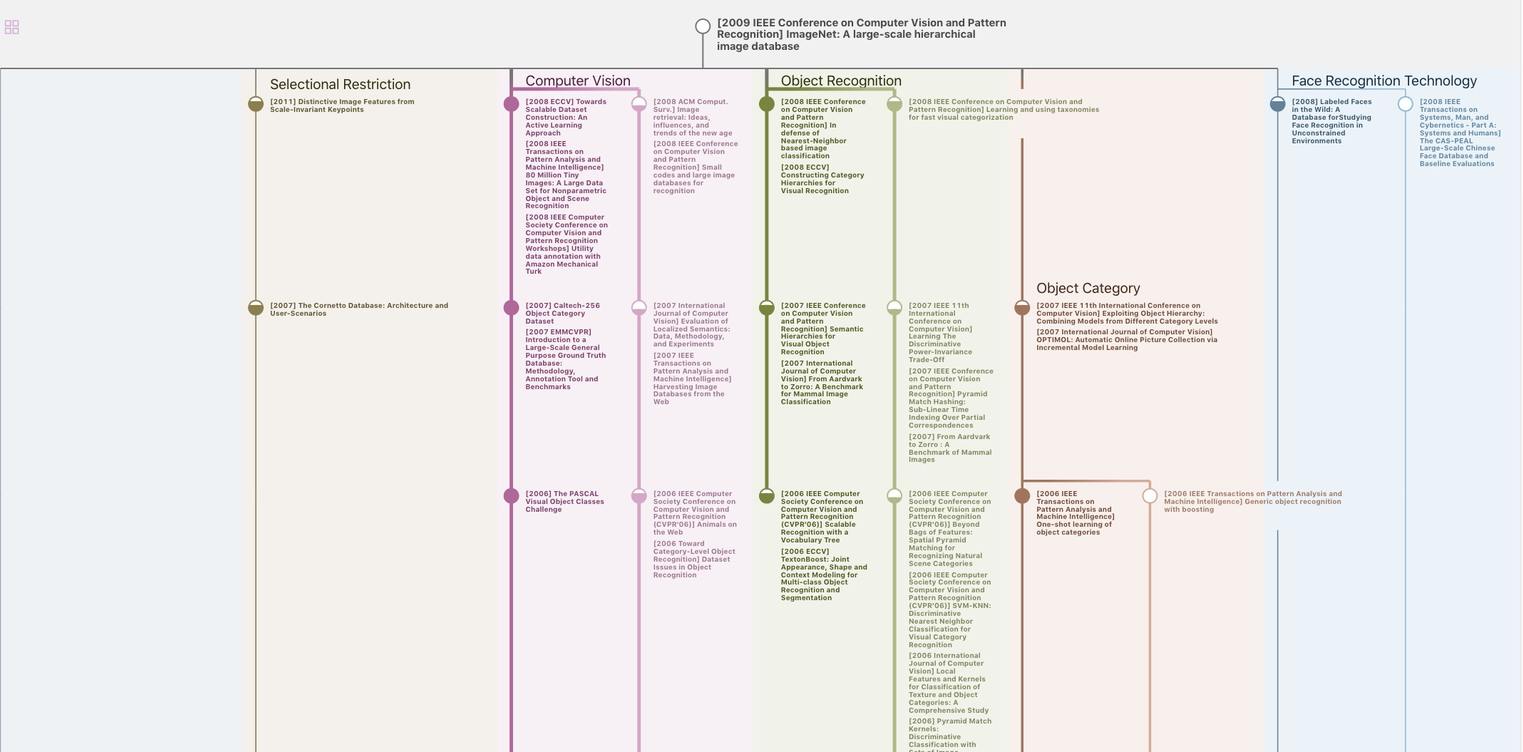
生成溯源树,研究论文发展脉络
Chat Paper
正在生成论文摘要