How Important is Importance Sampling for Deep Budgeted Training?
British Machine Vision Conference(2021)
摘要
Long iterative training processes for Deep Neural Networks (DNNs) are commonly required to achieve state-of-the-art performance in many computer vision tasks. Importance sampling approaches might play a key role in budgeted training regimes, i.e. when limiting the number of training iterations. These approaches aim at dynamically estimating the importance of each sample to focus on the most relevant and speed up convergence. This work explores this paradigm and how a budget constraint interacts with importance sampling approaches and data augmentation techniques. We show that under budget restrictions, importance sampling approaches do not provide a consistent improvement over uniform sampling. We suggest that, given a specific budget, the best course of action is to disregard the importance and introduce adequate data augmentation; e.g. when reducing the budget to a 30% in CIFAR-10/100, RICAP data augmentation maintains accuracy, while importance sampling does not. We conclude from our work that DNNs under budget restrictions benefit greatly from variety in the training set and that finding the right samples to train on is not the most effective strategy when balancing high performance with low computational requirements. Source code available at https://git.io/JKHa3 .
更多查看译文
关键词
importance sampling,deep budgeted training
AI 理解论文
溯源树
样例
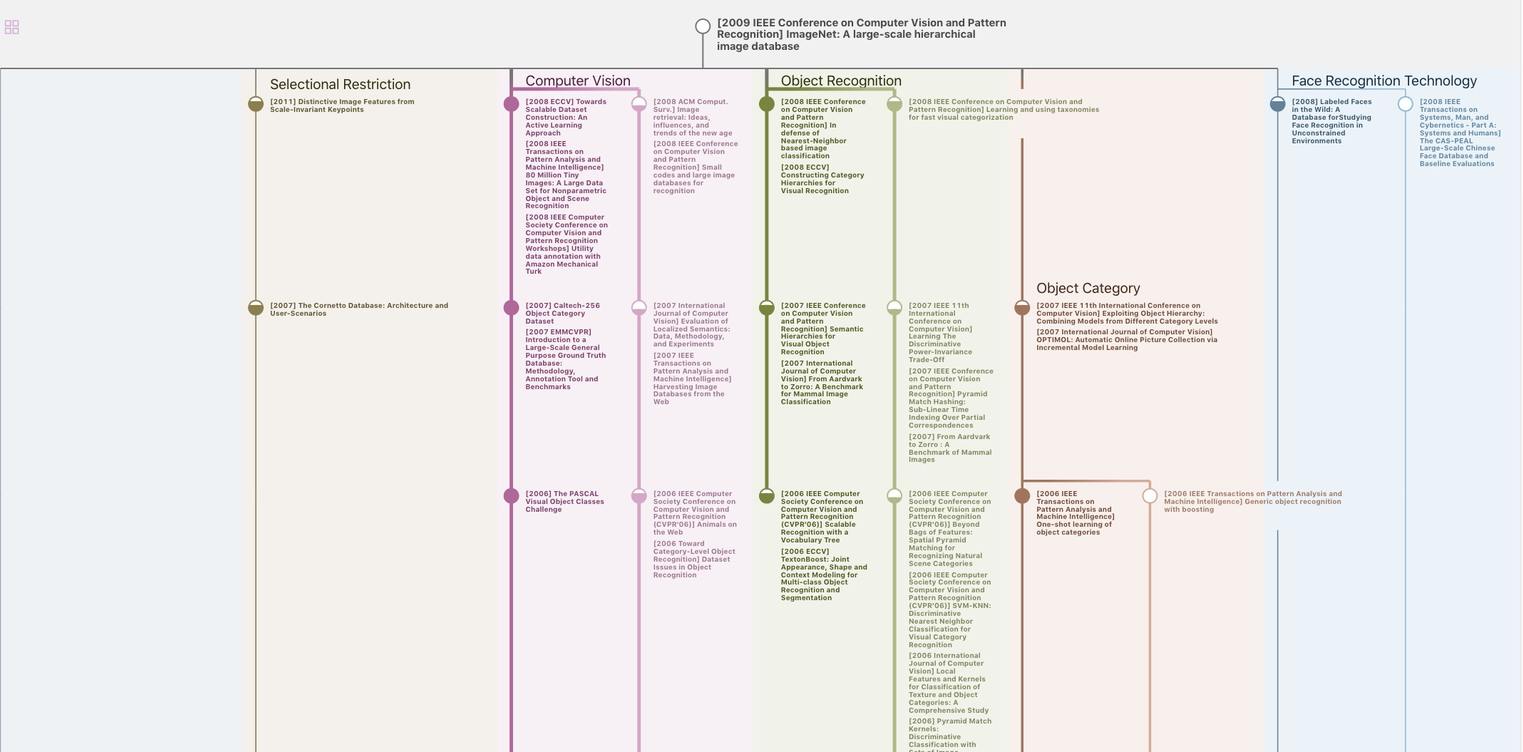
生成溯源树,研究论文发展脉络
Chat Paper
正在生成论文摘要