Don't read, just look: Main content extraction from web pages using visually apparent features
arxiv(2021)
摘要
The extraction of main content provides only primary informative blocks by removing a web page's minor areas like navigation menu, ads, and site templates. It has various applications: information retrieval, search engine optimization, and browser reader mode. We tested the existing four main content extraction methods (Firefox Readability.js, Chrome DOM Distiller, Web2Text, and Boilernet) in web pages datasets of two English datasets from the global websites and seven non-English datasets from seven local regions each. It shows that the performance decreases by up to 40% in non-English datasets over English datasets. This paper proposes a multilingual main content extraction method that uses visually apparent features such as the elements' positions, size, and distances from the centers of the browser window and the web document. These are based on the authors' intention: the elements' placement and appearance in web pages have constraints because of humans' narrow central vision. Hence, our method, Grid-Center-Expand (GCE), finds the closest leaf node to the centroid of the web page from which minor areas have been removed. For the main content, the leaf node repeatedly ascends to the parent node of the DOM tree until this node fits one of the following conditions: tag, containing specific attributes, or sudden width change. In the non-English datasets, our method performs better than up to 13% over Boilernet, especially 56% in the Japan dataset and 7% in the English dataset. Therefore, our method performs well regardless of the regional and linguistic characteristics of the web page. In addition, we create DNN models using Google's TabNet with GCE's features. The best of our models has similar performance to Boilernet and Web2text in all datasets. Accordingly, we show that these features can be useful to machine learning models for extracting main content.
更多查看译文
AI 理解论文
溯源树
样例
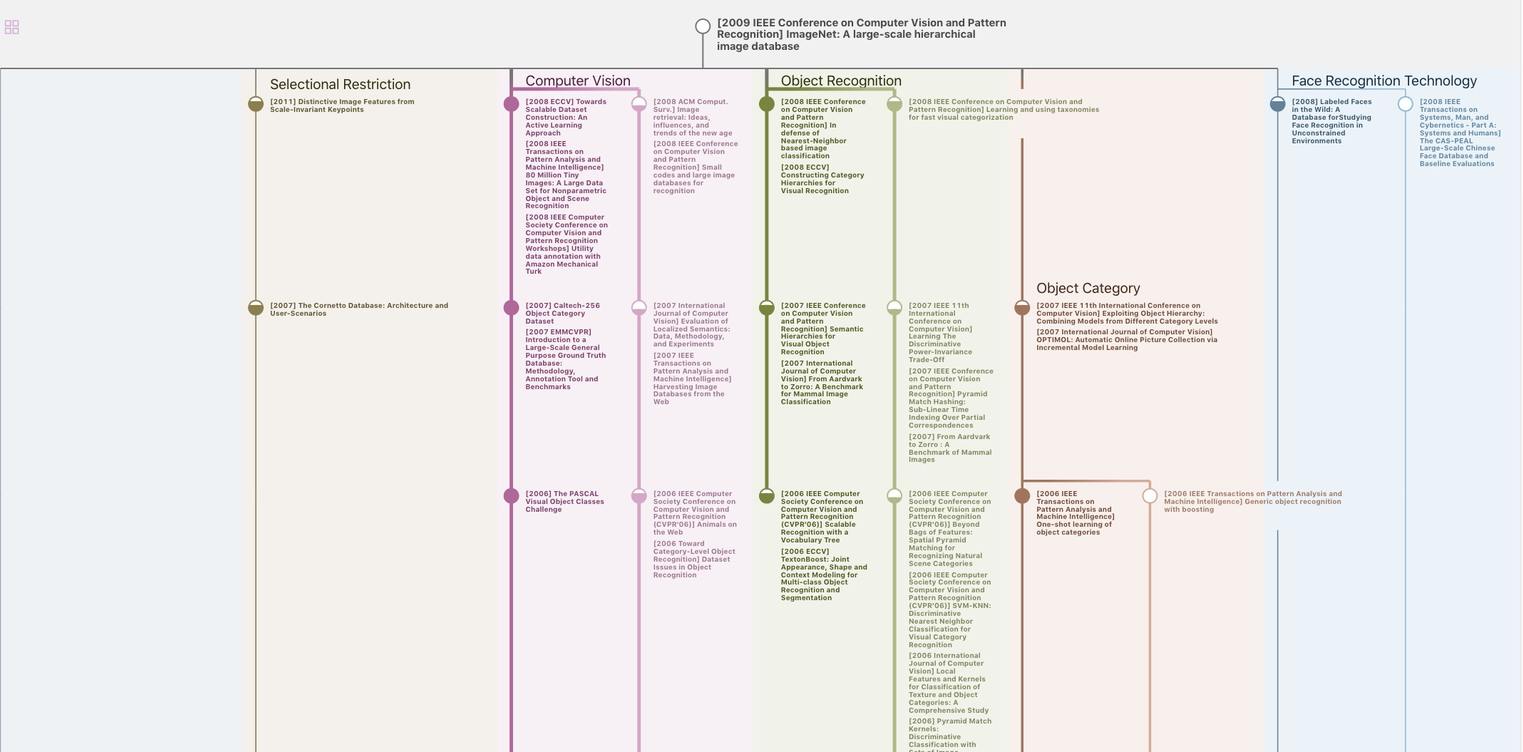
生成溯源树,研究论文发展脉络
Chat Paper
正在生成论文摘要