End-to-end Multi-Instance Robotic Reaching from Monocular Vision
2021 IEEE INTERNATIONAL CONFERENCE ON ROBOTICS AND AUTOMATION (ICRA 2021)(2024)
摘要
Multi-instance scenes are especially challenging for end-to-end visuomotor
(image-to-control) learning algorithms. "Pipeline" visual servo control
algorithms use separate detection, selection and servo stages, allowing
algorithms to focus on a single object instance during servo control.
End-to-end systems do not have separate detection and selection stages and need
to address the visual ambiguities introduced by the presence of arbitrary
number of visually identical or similar objects during servo control. However,
end-to-end schemes avoid embedding errors from detection and selection stages
in the servo control behaviour, are more dynamically robust to changing scenes,
and are algorithmically simpler. In this paper, we present a real-time
end-to-end visuomotor learning algorithm for multi-instance reaching. The
proposed algorithm uses a monocular RGB image and the manipulator's joint
angles as the input to a light-weight fully-convolutional network (FCN) to
generate control candidates. A key innovation of the proposed method is
identifying the optimal control candidate by regressing a control-Lyapunov
function (cLf) value. The multi-instance capability emerges naturally from the
stability analysis associated with the cLf formulation. We demonstrate the
proposed algorithm effectively reaching and grasping objects from different
categories on a table-top amid other instances and distractors from an
over-the-shoulder monocular RGB camera.
The network is able to run up to approximately 160 fps during inference on
one GTX 1080 Ti GPU.
更多查看译文
关键词
multiinstance robotic reaching,pipeline visual servo control algorithms,cLf formulation,end-to-end visuomotor learning algorithm,visually identical objects,image-to-control,monocular vision,control-Lyapunov function value,optimal control candidate,fully-convolutional network,monocular RGB image
AI 理解论文
溯源树
样例
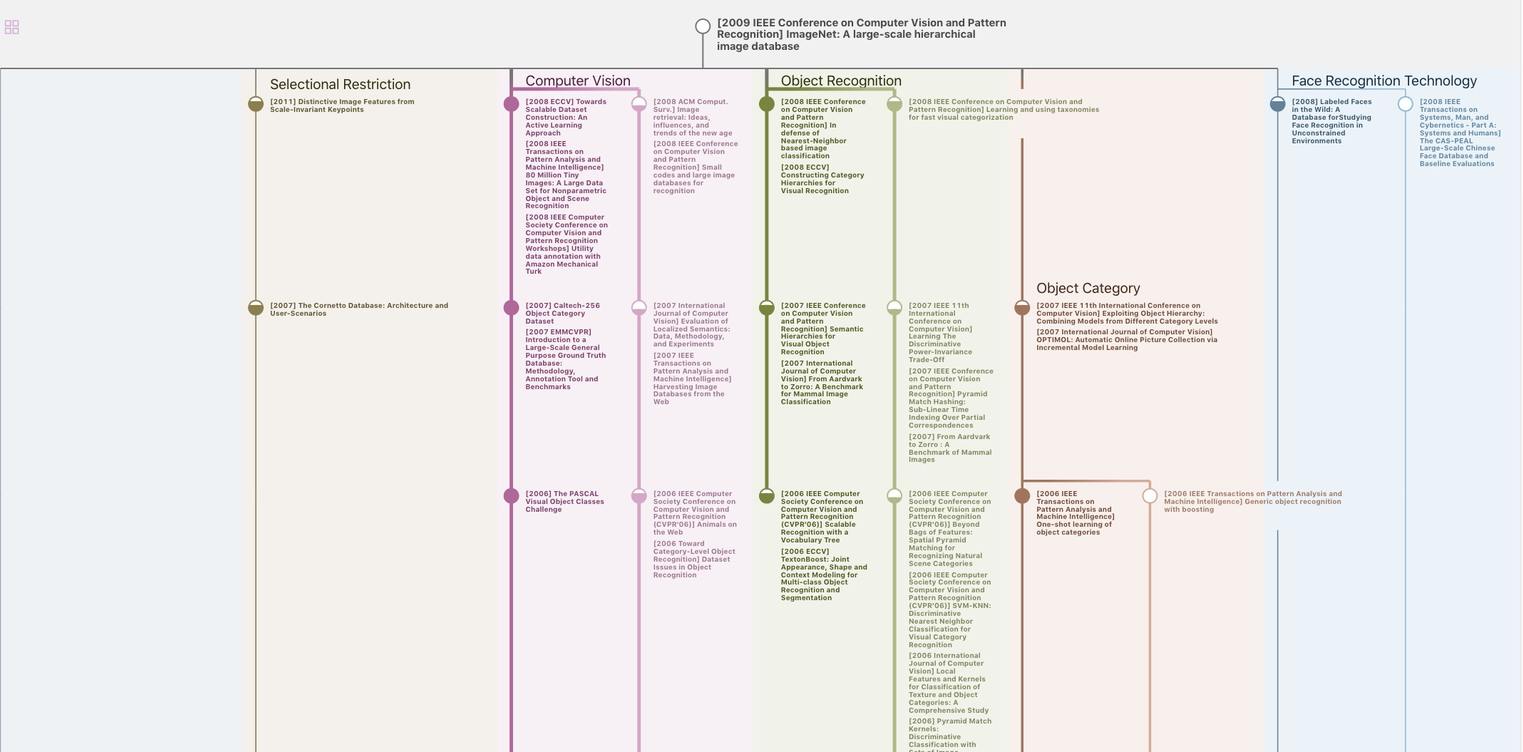
生成溯源树,研究论文发展脉络
Chat Paper
正在生成论文摘要