Real-time Optimal Navigation Planning Using Learned Motion Costs
2021 IEEE INTERNATIONAL CONFERENCE ON ROBOTICS AND AUTOMATION (ICRA 2021)(2021)
摘要
Navigation on challenging terrain topographies requires the understanding of robots' locomotion capabilities to produce optimal solutions. We present an integrated framework for real-time autonomous navigation of mobile robots based on elevation maps. The framework performs rapid global path planning and optimization that is aware of the locomotion capabilities of the robot. A GPU-aided, sampling-based path planner combined with a gradient-based path optimizer provides optimal paths by using a neural network-based locomotion cost predictor which is trained in simulation. We show that our approach is capable of planning and optimizing paths three orders of magnitude faster than RRT* on GPU-enabled hardware, enabling real-time deployment on mobile platforms. We successfully evaluate the framework on the ANYmal C quadrupedal robot in both simulations and real-world environments for path planning tasks on multiple complex terrains.
更多查看译文
关键词
time optimal navigation,learned motion costs,terrain topographies,optimal solutions,integrated framework,real-time autonomous navigation,mobile robots,elevation maps,rapid global path planning,optimization,locomotion capabilities,GPU-aided,sampling-based path planner,gradient-based path optimizer,optimal paths,neural network-based locomotion cost predictor,GPU-enabled hardware,real-time deployment,mobile platforms,ANYmal C quadrupedal robot,multiple complex terrains
AI 理解论文
溯源树
样例
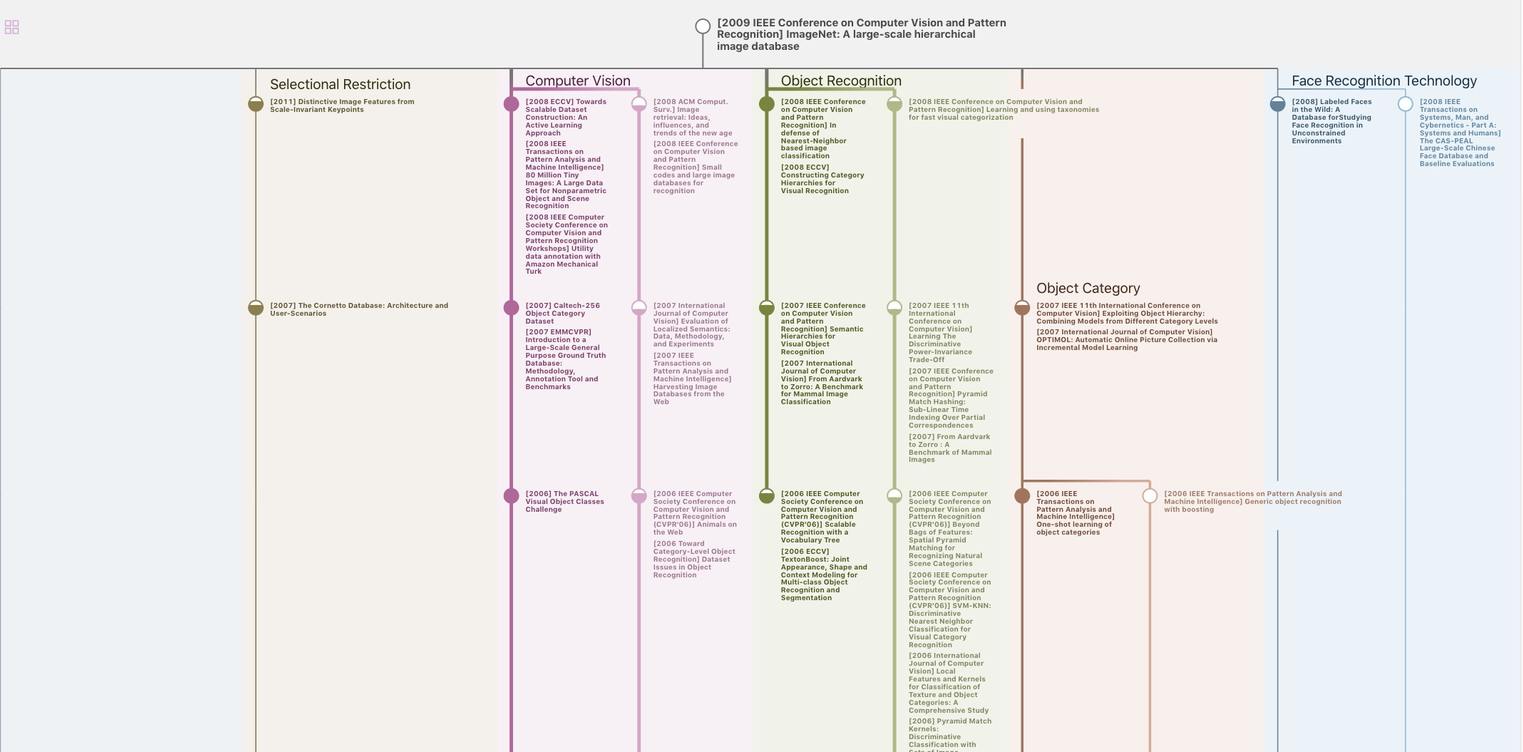
生成溯源树,研究论文发展脉络
Chat Paper
正在生成论文摘要