Hierarchies of Planning and Reinforcement Learning for Robot Navigation
2021 IEEE INTERNATIONAL CONFERENCE ON ROBOTICS AND AUTOMATION (ICRA 2021)(2021)
摘要
Solving robotic navigation tasks via reinforcement learning (RL) is challenging due to their sparse reward and long decision horizon nature. However, in many navigation tasks, high-level (HL) task representations, like a rough floor plan, are available. Previous work has demonstrated efficient learning by hierarchial approaches consisting of path planning in the HL representation and using sub-goals derived from the plan to guide the RL policy in the source task. However, these approaches usually neglect the complex dynamics and sub-optimal sub-goal-reaching capabilities of the robot during planning. This work overcomes these limitations by proposing a novel hierarchical framework that utilizes a trainable planning policy for the IIL representation. Thereby robot capabilities and environment conditions can be learned utilizing collected rollout data. We specifically introduce a planning policy based on value iteration with a learned transition model (VI-RL). In simulated robotic navigation tasks, VI-RL results in consistent strong improvement over vanilla RL, is on par with vanilla hierarchal RL on single layouts but more broadly applicable to multiple layouts, and is on par with trainable HL path planning baselines except for a parking task with difficult non-holonomic dynamics where it shows marked improvements.
更多查看译文
关键词
trainable HL path planning,parking task,reinforcement learning,robot navigation,sparse reward,long decision horizon nature,high-level task representations,rough floor plan,hierarchal approaches,HL representation,using sub-goals,RL policy,source task,complex dynamics,sub-optimal sub-goal-reaching,novel hierarchical framework,trainable planning policy,robot capabilities,collected rollout data,learned transition model,simulated robotic navigation tasks,VI-RL results,consistent strong improvement,vanilla RL,vanilla hierarchal RL
AI 理解论文
溯源树
样例
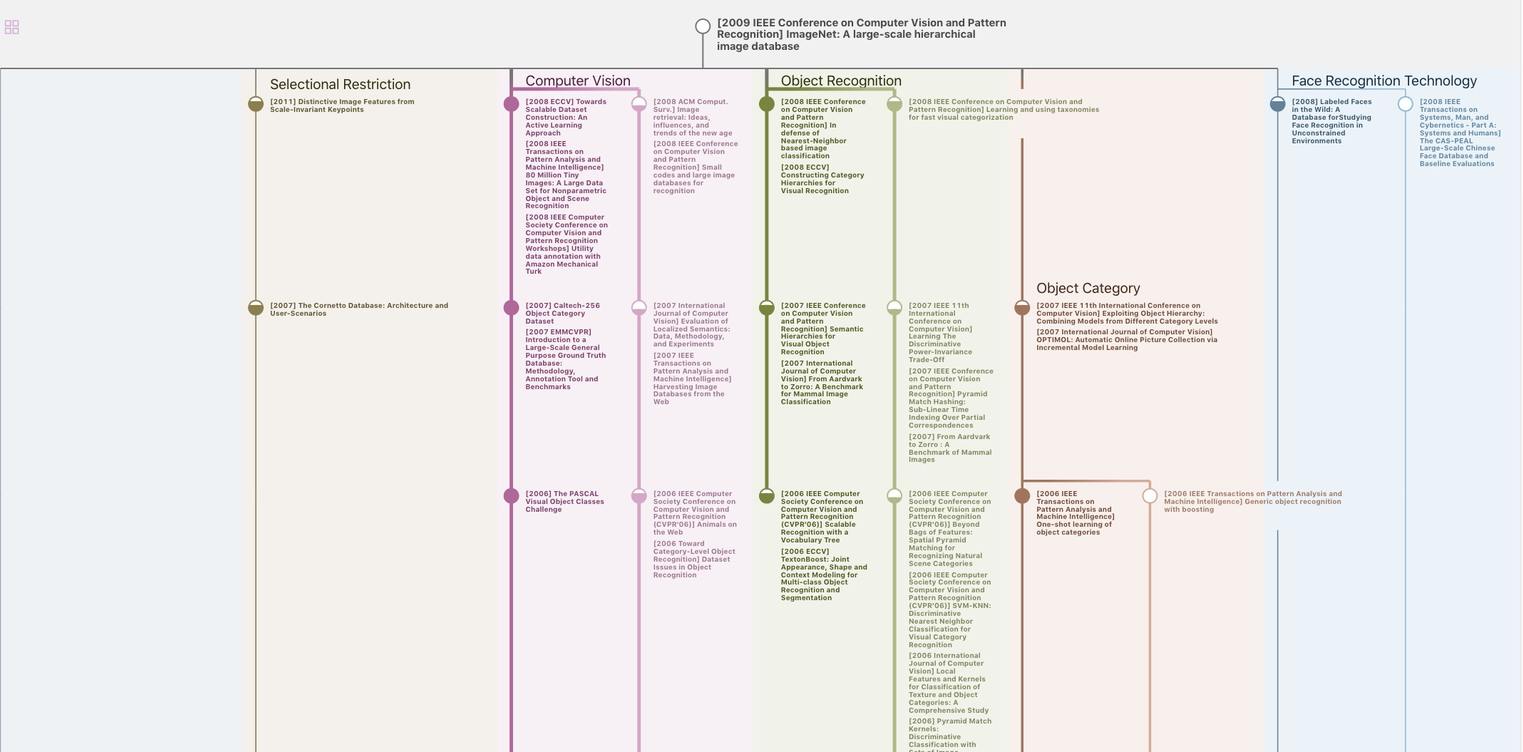
生成溯源树,研究论文发展脉络
Chat Paper
正在生成论文摘要