DeepWalk: Omnidirectional Bipedal Gait by Deep Reinforcement Learning
2021 IEEE INTERNATIONAL CONFERENCE ON ROBOTICS AND AUTOMATION (ICRA 2021)(2021)
摘要
Bipedal walking is one of the most difficult but exciting challenges in robotics. The difficulties arise from the complexity of high-dimensional dynamics, sensing and actuation limitations combined with real-time and computational constraints. Deep Reinforcement Learning (DRL) holds the promise to address these issues by fully exploiting the robot dynamics with minimal craftsmanship. In this paper, we propose a novel DRL approach that enables an agent to learn omnidirectional locomotion for humanoid (bipedal) robots. Notably, the locomotion behaviors are accomplished by a single control policy (a single neural network). We achieve this by introducing a new curriculum learning method that gradually increases the task difficulty by scheduling target velocities. In addition, our method does not require reference motions which facilities its application to robots with different kinematics, and reduces the overall complexity. Finally, different strategies for sim-to-real transfer are presented which allow us to transfer the learned policy to a real humanoid robot.
更多查看译文
关键词
omnidirectional bipedal gait,deepwalk reinforcement learning
AI 理解论文
溯源树
样例
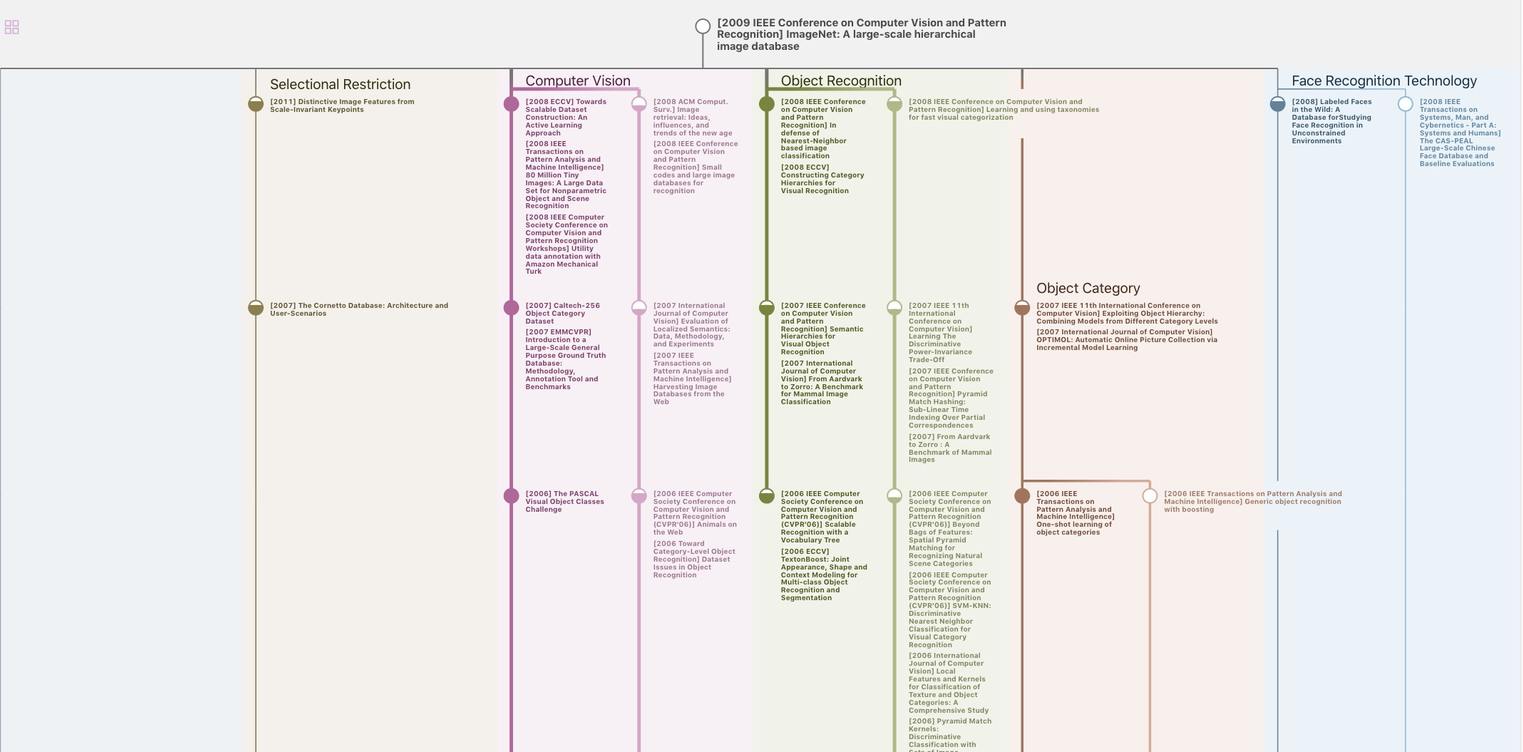
生成溯源树,研究论文发展脉络
Chat Paper
正在生成论文摘要