3D Multi-Object Tracking using Random Finite Set-based Multiple Measurement Models Filtering (RFS-M-3) for Autonomous Vehicles
2021 IEEE INTERNATIONAL CONFERENCE ON ROBOTICS AND AUTOMATION (ICRA 2021)(2021)
摘要
Multiple object tracking (MOT) is a critical module for enabling autonomous vehicles to achieve safe planing and navigation in cluttered environments. In tracking-by-detection systems, there are inevitably many false positives and misses among learning-based input detections. The challenge for MOT is to combine these detections into tracks, and filter them based on their uncertainties, states, and temporal consistency to achieve accurate and persistent tracks. In this paper, we propose to solve the 3D MOT problem for autonomous driving applications using a random finite set-based (RFS) Multiple Measurement Models filter (RFS-M-3). In partiuclar, we propose multiple measurement models for a Poisson multi-Bernoulli mixture (PMBM) filter in support of different application scenarios. Our RFS-M-3 filter can naturally model these uncertainties accurately and elegantly. We combine the learning-based detections with our RFS-M-3 tracker through incorporating the detection confidence score into the PMBM prediction and update step. The superior experimental results of our RFS-M-3 tracker on Waymo, Argoverse and nuSceness datasets illustrate that our RFS-M-3 tracker outperforms state-of-the-art deep learning-based and traditional filter-based approaches. To the best of our knowledge, this represents a first successful attempt for employing an RFS-based approach in conjunction with 3D learning-based amodal detections for 3D MOT applications with comprehensive validation using challenging datasets made available by industry leaders.
更多查看译文
AI 理解论文
溯源树
样例
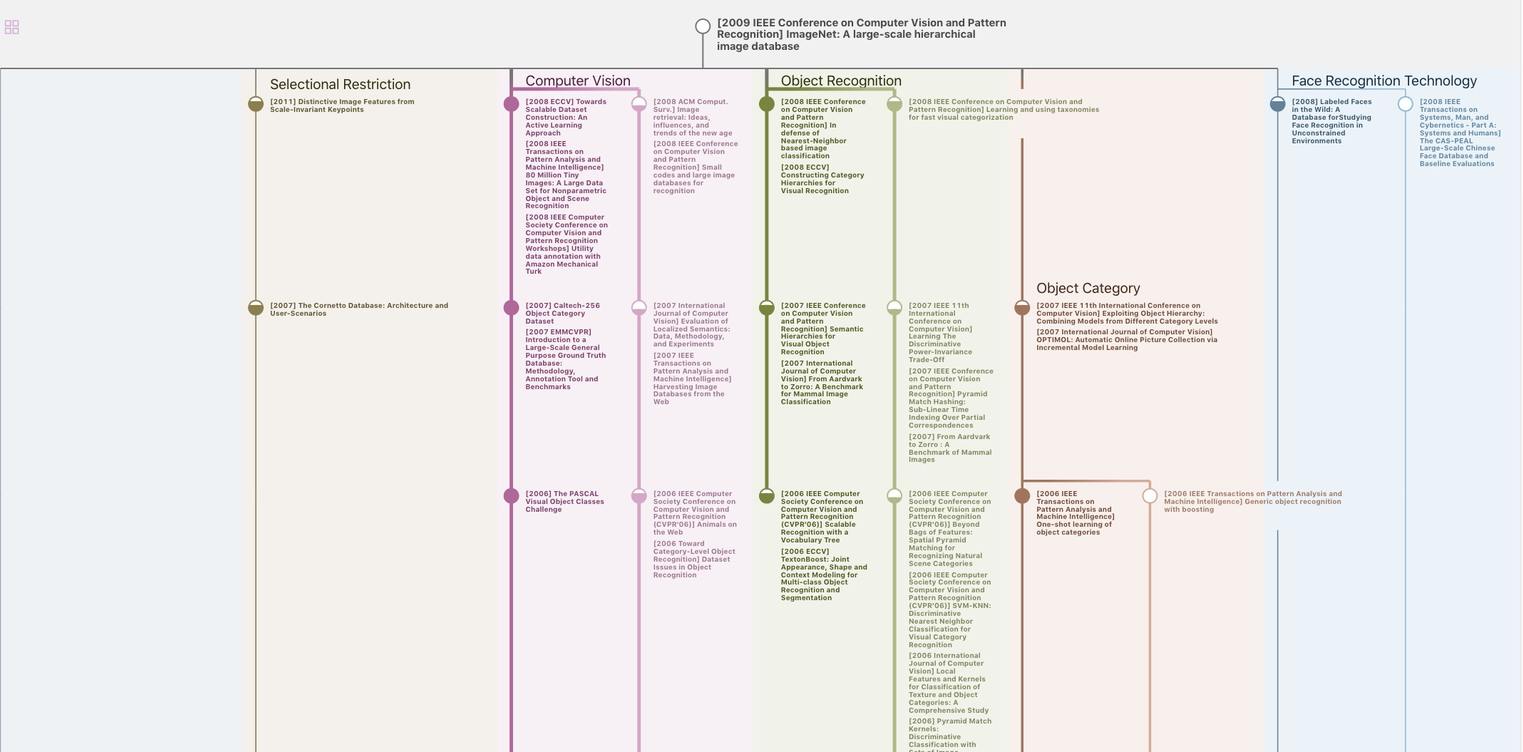
生成溯源树,研究论文发展脉络
Chat Paper
正在生成论文摘要