Efficient Real-Time Inference in Temporal Convolution Networks
2021 IEEE INTERNATIONAL CONFERENCE ON ROBOTICS AND AUTOMATION (ICRA 2021)(2021)
摘要
It has been recently demonstrated that Temporal Convolution Networks (TCNs) provide state-of-the-art results in many problem domains where the input data is a time-series. TCNs typically incorporate information from a long history of inputs (the receptive field) into a single output using many convolution layers. Real-time inference using a trained TCN can be challenging on devices with limited compute and memory, especially if the receptive field is large. This paper introduces the RT-TCN algorithm that reuses the output of prior convolution operations to minimize the computational requirements and persistent memory footprint of a TCN during real-time inference. We also show that when a TCN is trained using time slices of the input time-series, it can be executed in real-time continually using RT-TCN. In addition, we provide TCN architecture guidelines that ensure that real-time inference can be performed within memory and computational constraints.
更多查看译文
关键词
persistent memory footprint,time slices,TCN architecture guidelines,computational constraints,time series data,real time inference,temporal convolution networks,trained TCN,RT-TCN algorithm
AI 理解论文
溯源树
样例
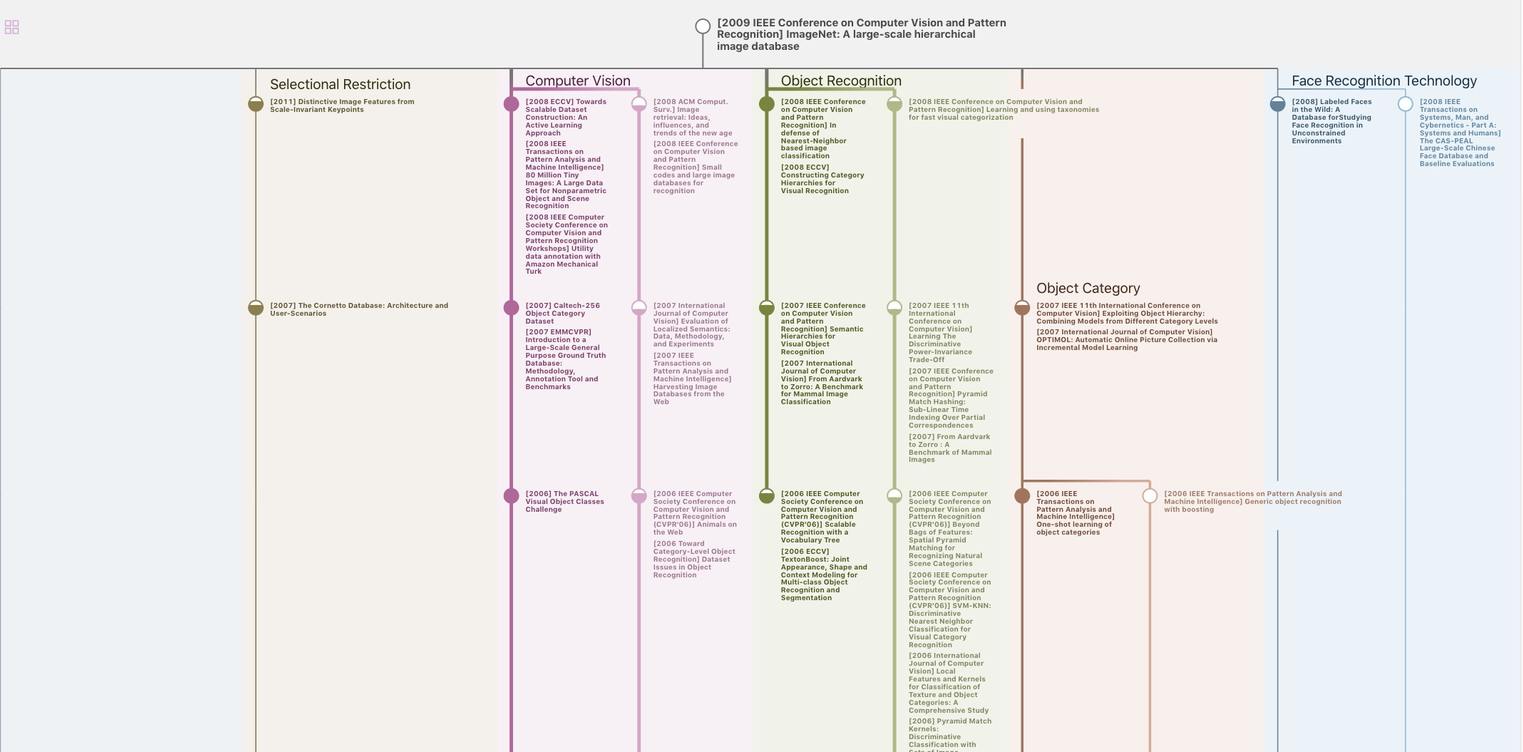
生成溯源树,研究论文发展脉络
Chat Paper
正在生成论文摘要