MonoSOD: Monocular Salient Object Detection based on Predicted Depth
2021 IEEE INTERNATIONAL CONFERENCE ON ROBOTICS AND AUTOMATION (ICRA 2021)(2021)
摘要
Salient object detection (SOD) can directly improve the performance of tasks like obstacle detection, semantic segmentation and object recognition. Such tasks are important for robotic and other autonomous navigation systems. State-of-the-art SOD methodologies, provide improved performance by incorporating depth information, usually acquired using additional specialized sensors, e.g., RGB-D cameras. This introduces an overhead to the overall cost and flexibility of such systems. Nevertheless, the recent advances of machine learning, have provided models, capable of generating depth map approximations, given a single RGB image. In this work, we propose a novel monocular SOD (MonoSOD) methodology, based on a two-branch CNN autoencoder architecture capable of predicting depth maps and estimating saliency through a trainable refinement scheme. Its application on benchmark datasets, indicates that its performance is comparable to that of state-of-the-art SOD methods relying on RGB-D data. Therefore, it could be considered as a lower-cost alternative of such methods for future applications.
更多查看译文
关键词
predicted depth,obstacle detection,semantic segmentation,robotic navigation systems,other autonomous navigation systems,state-of-the-art SOD methodologies,depth information,RGB-D cameras,flexibility,machine learning,depth map approximations,single RGB image,novel monocular SOD methodology,MonoSOD,two-branch CNN autoencoder architecture,depth maps,estimating saliency,state-of-the-art SOD methods,RGB-D data,monocular salient object detection
AI 理解论文
溯源树
样例
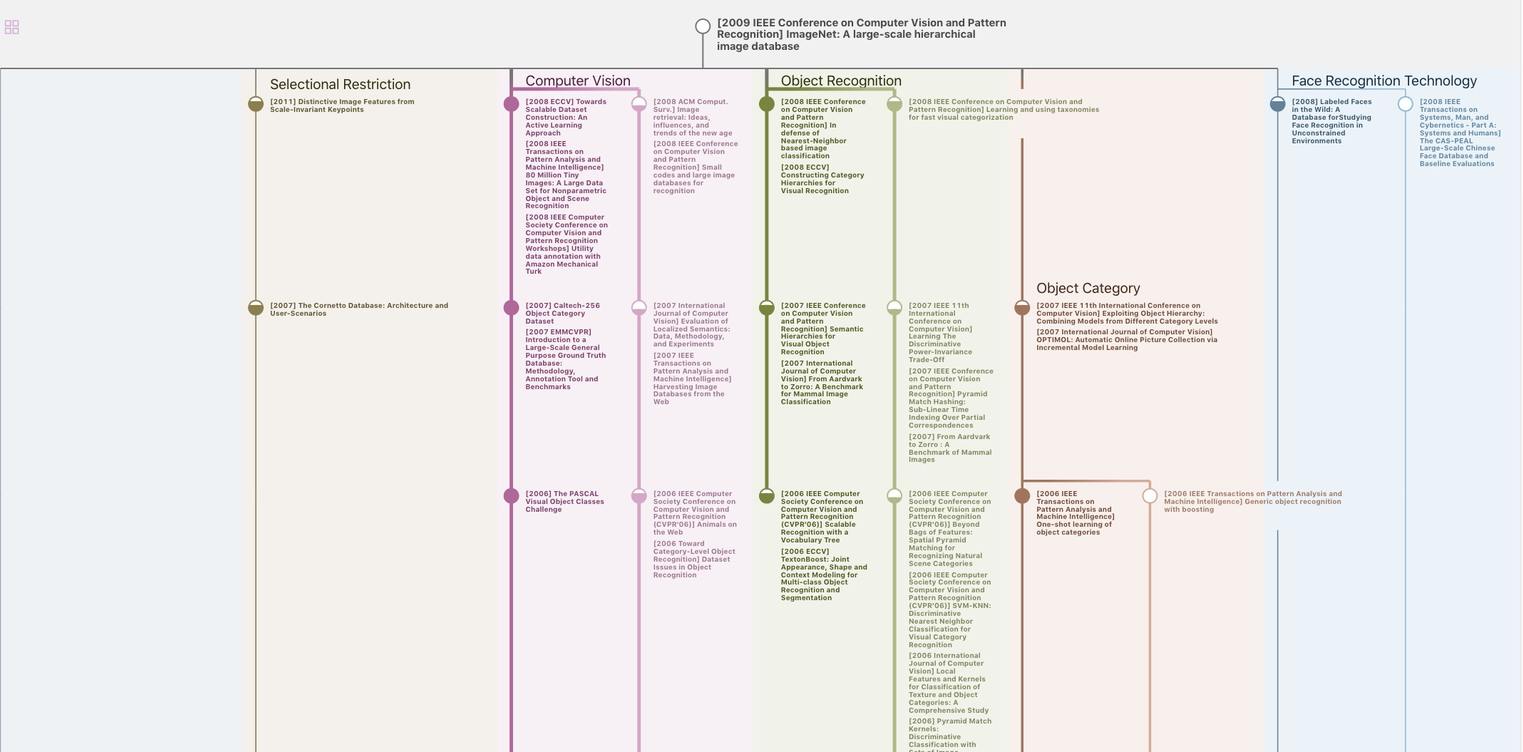
生成溯源树,研究论文发展脉络
Chat Paper
正在生成论文摘要