Event-Triggered Neural-Network Adaptive Control for Strict-Feedback Nonlinear Systems: Selections on Valid Compact Sets
IEEE transactions on neural networks and learning systems(2023)
摘要
This article studies neural-network (NN) adaptive control for strict-feedback nonlinear systems with matched uncertainties and event-triggered communication. Radial basis function NNs (RBFNNs) are used in the backstepping design approach to compensate for nonlinear uncertain functions. The concept of valid compact sets for RBFNN adaptive controllers is proposed, where a local RBFNN approximator is defined and the closed-loop state can remain. To guarantee the existence of such valid compact sets, a new property on RBFNNs is presented, which shows that, in some properly designed RBFNNs, the norm of their ideal weight vectors can always become arbitrarily small. By utilizing this property, the selections on valid compact sets are investigated, resulting in rigorous proof on RBFNN adaptive controllers to solve a local tracking problem with a given smooth enough reference signal. Subsequently, to save limited communication resources, a Zeno-free event-triggering mechanism in controller-to-actuator channels is proposed. Under this event-triggered adaptive controller, the corresponding tradeoff among the tracking performance, computational burden, and communication consumption is analyzed. Furthermore, two extensions are made to the general local function approximator, which is in the form of a weight vector multiplying a group of basis functions, and to the communication in sensor-to-controller channels. Finally, several simulation results are provided to illustrate the efficiency and feasibility of the obtained results.
更多查看译文
关键词
Artificial neural networks,Adaptive control,Backstepping,Uncertainty,Nonlinear dynamical systems,Systematics,Stability analysis,Backstepping,event-triggered control,neural-network (NN) adaptive control,strict-feedback nonlinear systems
AI 理解论文
溯源树
样例
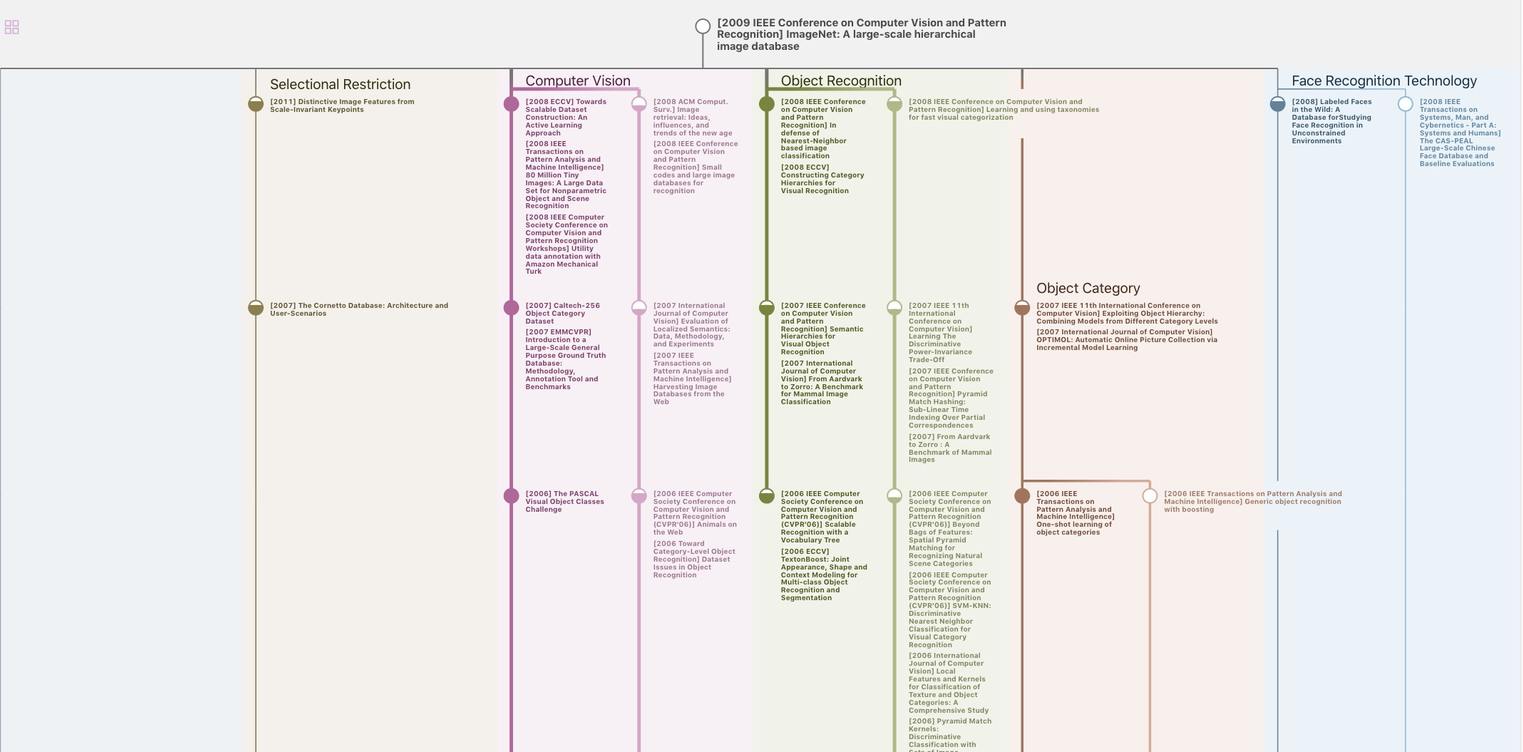
生成溯源树,研究论文发展脉络
Chat Paper
正在生成论文摘要