Learning rich features for gait recognition by integrating skeletons and silhouettes
Multimedia Tools and Applications(2024)
摘要
Gait recognition captures gait patterns from the walking sequence of an individual for identification. Most existing gait recognition methods learn features from silhouettes or skeletons for the robustness to clothing, carrying, and other exterior factors. The combination of the two data modalities, however, is not fully exploited. Previous multimodal gait recognition methods mainly employ the skeleton to assist the local feature extraction where the intrinsic discrimination of the skeleton data is ignored. To fill this gap and make full use of the two complementary data modalities, this paper proposes a simple yet effective Bimodal Fusion (BiFusion) network which mines discriminative gait patterns in skeletons and integrates with silhouette representations to learn rich features for better identification. Particularly, the inherent hierarchical semantics of body joints in a skeleton is leveraged to design a novel Multi-Scale Gait Graph (MSGG) network for the feature extraction of skeletons. Extensive experiments on CASIA-B and OUMVLP demonstrate both the superiority of the proposed MSGG network in modeling skeletons and the effectiveness of the bimodal fusion for gait recognition. Under the most challenging condition of cross-clothing gait recognition on CASIA-B, our method achieves the rank-1 accuracy of 94.0%, which outperforms previous state-of-the-art methods by a large margin. The code is released at https://github.com/YunjiePeng/BimodalFusion .
更多查看译文
AI 理解论文
溯源树
样例
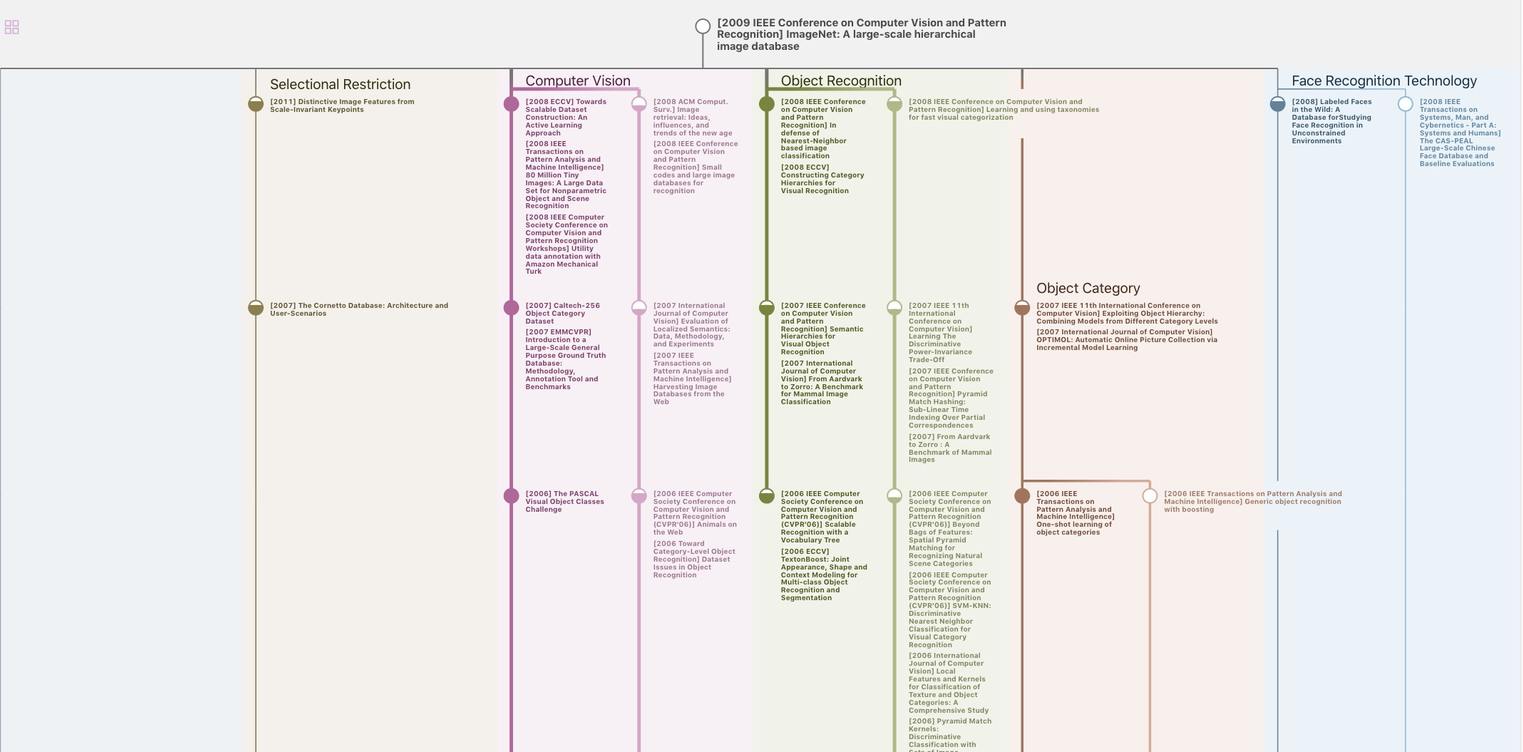
生成溯源树,研究论文发展脉络
Chat Paper
正在生成论文摘要