Grounding deep neural network predictions of human categorization behavior in understandable functional features: The case of face identity
PATTERNS(2021)
摘要
Deep neural networks (DNNs) can resolve real-world categorization tasks with apparent human-level performance. However, true equivalence of behavioral performance between humans and their DNN models requires that their internal mechanisms process equivalent features of the stimulus. To develop such feature equivalence, our methodology leveraged an interpretable and experimentally controlled generative model of the stimuli (realistic three-dimensional textured faces). Humans rated the similarity of randomly generated faces to four familiar identities. We predicted these similarity ratings from the activations of five DNNs trained with different optimization objectives. Using information theoretic redundancy, reverse correlation, and the testing of generalization gradients, we show that DNN predictions of human behavior improve because their shape and texture features overlap with those that subsume human behavior. Thus, we must equate the functional features that subsume the behavioral performances of the brain and its models before comparing where, when, and how these features are processed.
更多查看译文
关键词
autoencoder,categorization,deep neural networks,face,generalization,information theory,reverse correlation,shape,texture,visual cognition
AI 理解论文
溯源树
样例
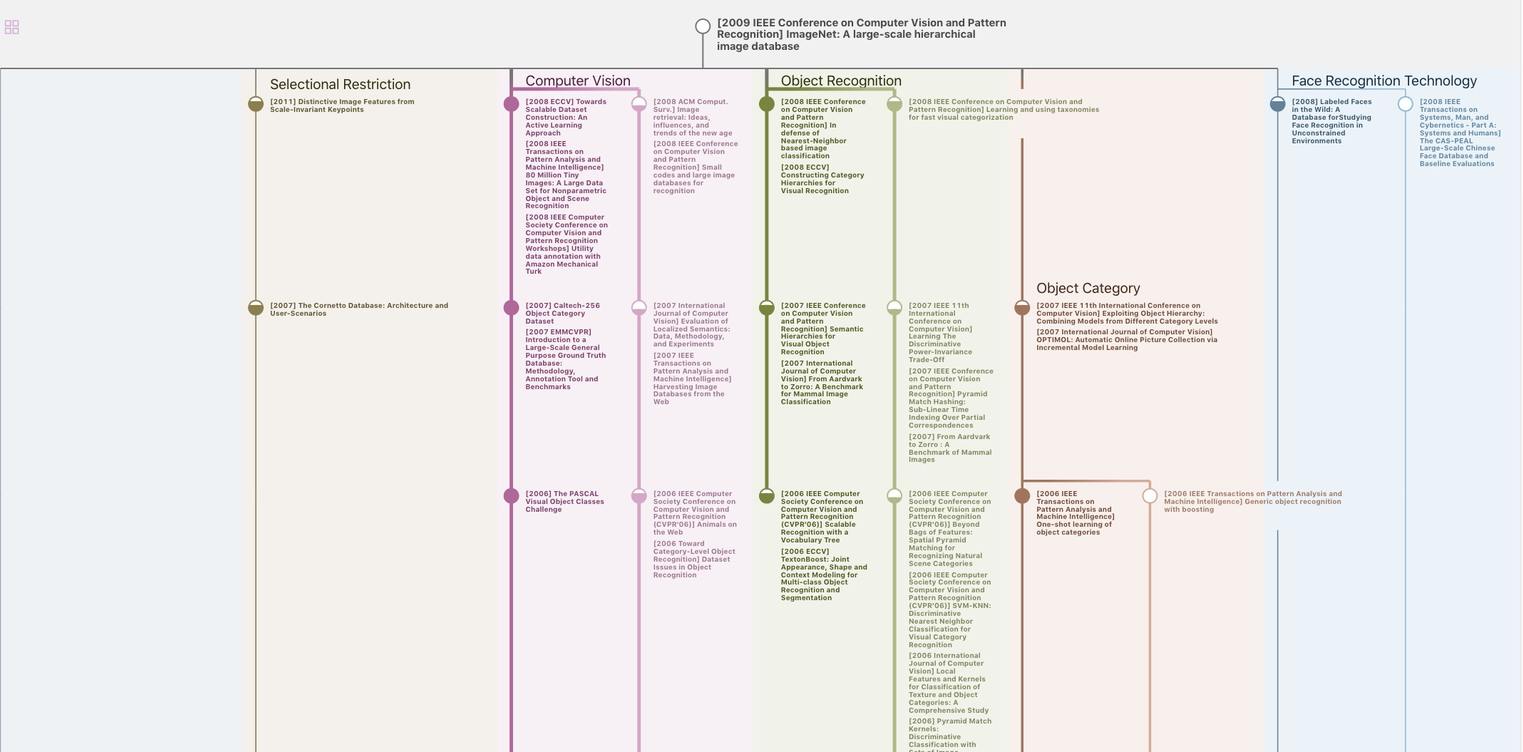
生成溯源树,研究论文发展脉络
Chat Paper
正在生成论文摘要