Mapping geographic clusters of new HIV diagnoses to inform granular-level interventions for HIV epidemic control in western Kenya
BMC PUBLIC HEALTH(2021)
摘要
Background As countries make progress towards HIV epidemic control, there is increasing need to identify finer geographic areas to target HIV interventions. We mapped geographic clusters of new HIV diagnoses, and described factors associated with HIV-positive diagnosis, in order to inform targeting of HIV interventions to finer geographic areas and sub-populations. Methods We analyzed data for clients aged > 15 years who received home-based HIV testing as part of a routine public health program between May 2016 and July 2017 in Siaya County, western Kenya. Geospatial analysis using Kulldorff’s spatial scan statistic was used to detect geographic clusters (radius < 5 kilometers) of new HIV diagnoses. Factors associated with new HIV diagnosis were assessed in a spatially-integrated Bayesian hierarchical model. Results Of 268,153 clients with HIV test results, 2906 (1.1%) were diagnosed HIV-positive. We found spatial variation in the distribution of new HIV diagnoses, and identified nine clusters in which the number of new HIV diagnoses was significantly (1.56 to 2.64 times) higher than expected. Sub-populations with significantly higher HIV-positive yield identified in the multivariable spatially-integrated Bayesian model included: clients aged 20–24 years [adjusted relative risk (aRR) 3.45, 95% Bayesian Credible Intervals (CI) 2.85–4.20], 25–35 years (aRR 4.76, 95% CI 3.92–5.81) and > 35 years (aRR 2.44, 95% CI 1.99–3.00); those in polygamous marriage (aRR 1.84, 95% CI 1.55–2.16), or separated/divorced (aRR 3.36, 95% CI 2.72–4.08); and clients who reported having never been tested for HIV (aRR 2.35, 95% CI 2.02–2.72), or having been tested > 12 months ago (aRR 1.53, 95% CI 1.41–1.66). Conclusion Our study used routine public health program data to identify granular geographic clusters of higher new HIV diagnoses, and sub-populations with higher HIV-positive yield in the setting of a generalized HIV epidemic. In order to target HIV testing and prevention interventions to finer granular geographic areas for maximal epidemiologic impact, integrating geospatial analysis into routine public health programs can be useful.
更多查看译文
关键词
Geospatial analysis, Mapping geographic clusters, New HIV diagnoses, Kenya
AI 理解论文
溯源树
样例
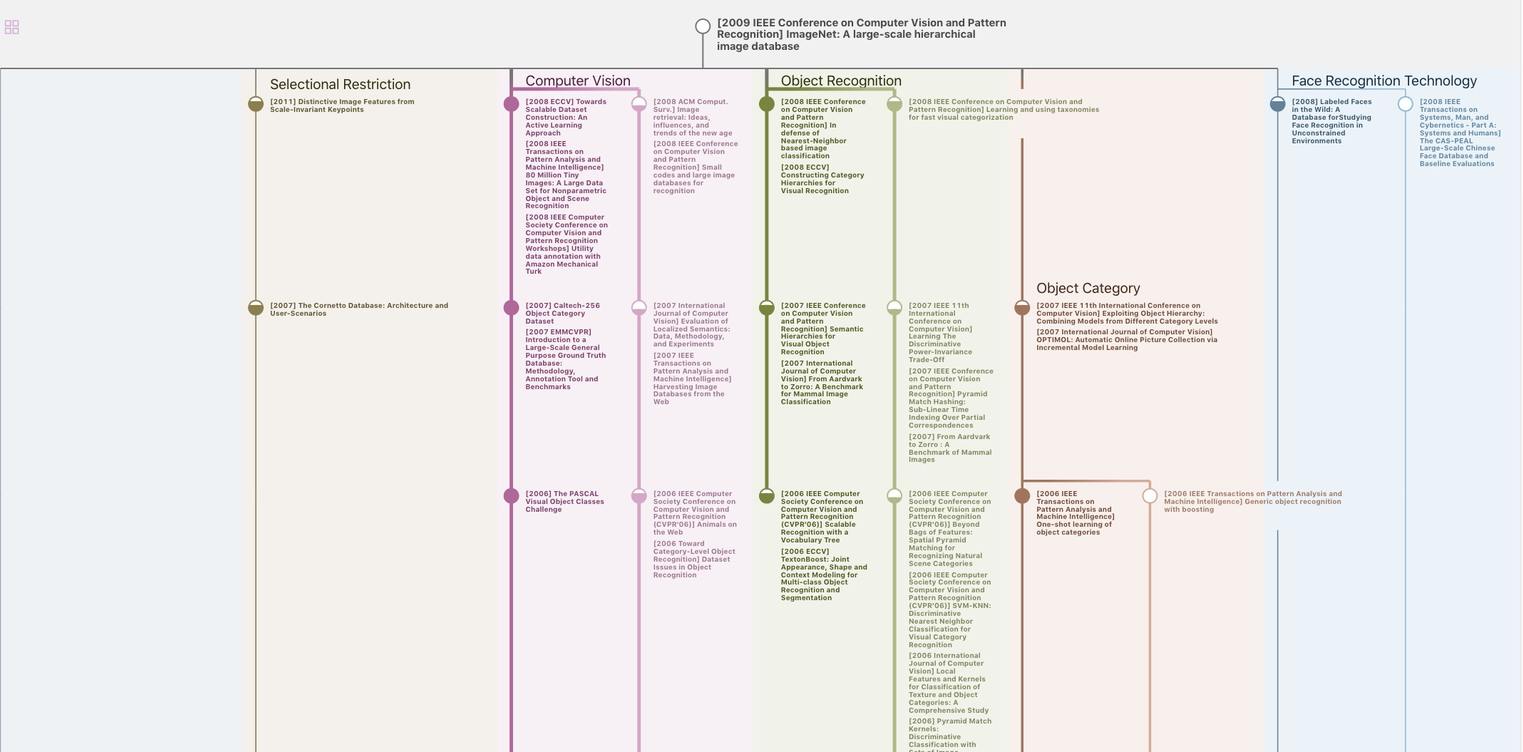
生成溯源树,研究论文发展脉络
Chat Paper
正在生成论文摘要