A robust fuzzy approach for gene expression data clustering
Soft Computing(2021)
摘要
In the big data era, clustering is one of the most popular data mining method. Most clustering algorithms have complications like automatic cluster number determination, poor clustering precision, inconsistent clustering of various datasets and parameter-dependent, etc. A new fuzzy autonomous solution for clustering named Meskat-Mahmudul (MM) clustering algorithm was proposed to overcome the complexity of parameter-free automatic cluster number determination and clustering accuracy. The Meskat-Mahmudul clustering algorithm finds out the exact number of clusters based on the average silhouette method in multivariate mixed attribute dataset, including real-time gene expression dataset and missing values, noise, and outliers. Meskat-Mahmudul Extended K-Means (MMK) clustering algorithm enhances the K-Means algorithm, which serves the purpose of automatic cluster discovery and runtime cluster placement. Several validation methods are used to evaluate clusters and certify optimum cluster partitioning and perfection. Some datasets are used to assess the performance of the proposed algorithms to other algorithms in terms of time complexity and clustering efficiency. Finally, Meskat-Mahmudul clustering and Meskat-Mahmudul Extended K-Means clustering algorithms were found superior over conventional algorithms.
更多查看译文
关键词
FCM, K-Means, Fuzzy clustering, Clustering algorithm, Data mining
AI 理解论文
溯源树
样例
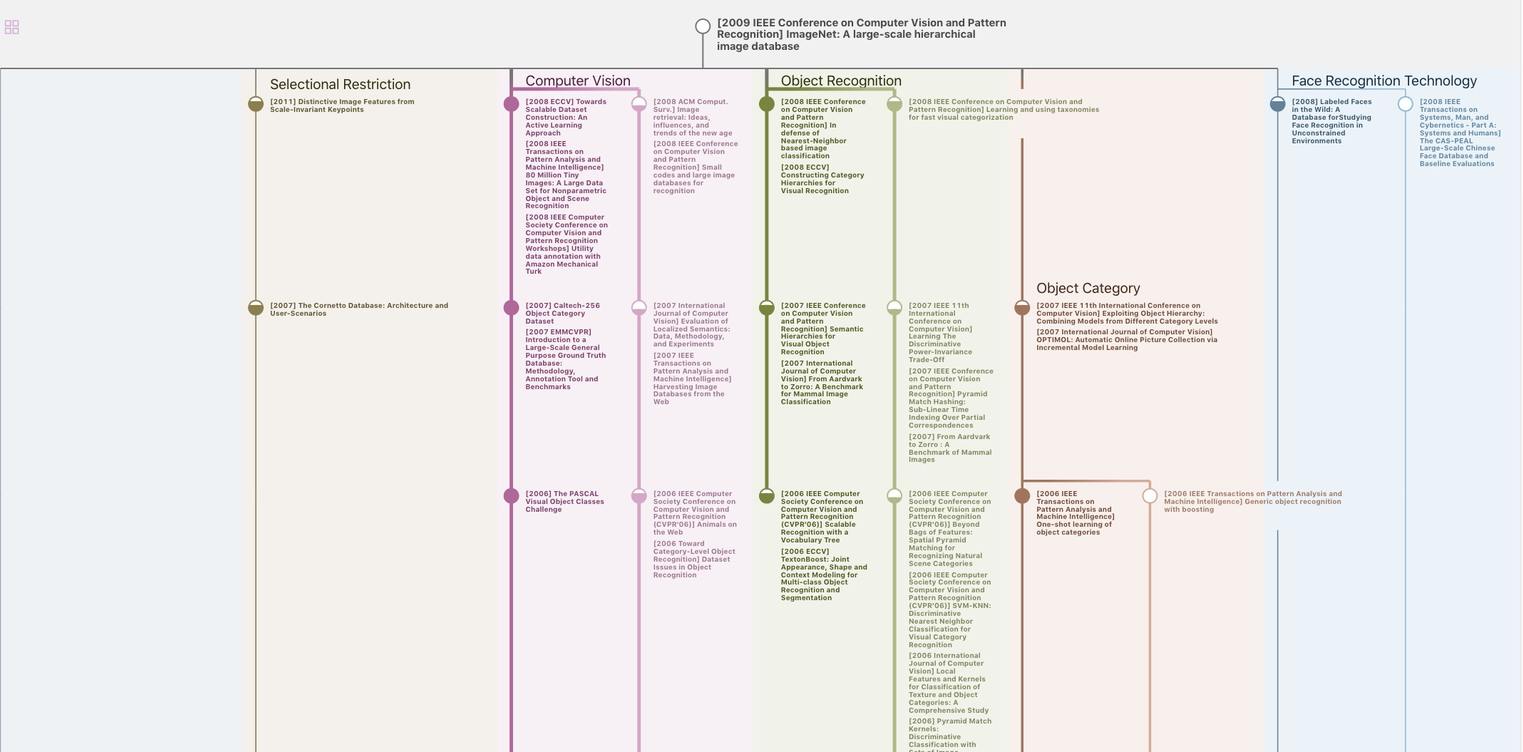
生成溯源树,研究论文发展脉络
Chat Paper
正在生成论文摘要