Generative Residual Attention Network for Disease Detection
arxiv(2021)
摘要
Accurate identification and localization of abnormalities from radiology images serve as a critical role in computer-aided diagnosis (CAD) systems. Building a highly generalizable system usually requires a large amount of data with high-quality annotations, including disease-specific global and localization information. However, in medical images, only a limited number of high-quality images and annotations are available due to annotation expenses. In this paper, we explore this problem by presenting a novel approach for disease generation in X-rays using a conditional generative adversarial learning. Specifically, given a chest X-ray image from a source domain, we generate a corresponding radiology image in a target domain while preserving the identity of the patient. We then use the generated X-ray image in the target domain to augment our training to improve the detection performance. We also present a unified framework that simultaneously performs disease generation and localization.We evaluate the proposed approach on the X-ray image dataset provided by the Radiological Society of North America (RSNA), surpassing the state-of-the-art baseline detection algorithms.
更多查看译文
关键词
generative residual attention network,disease detection
AI 理解论文
溯源树
样例
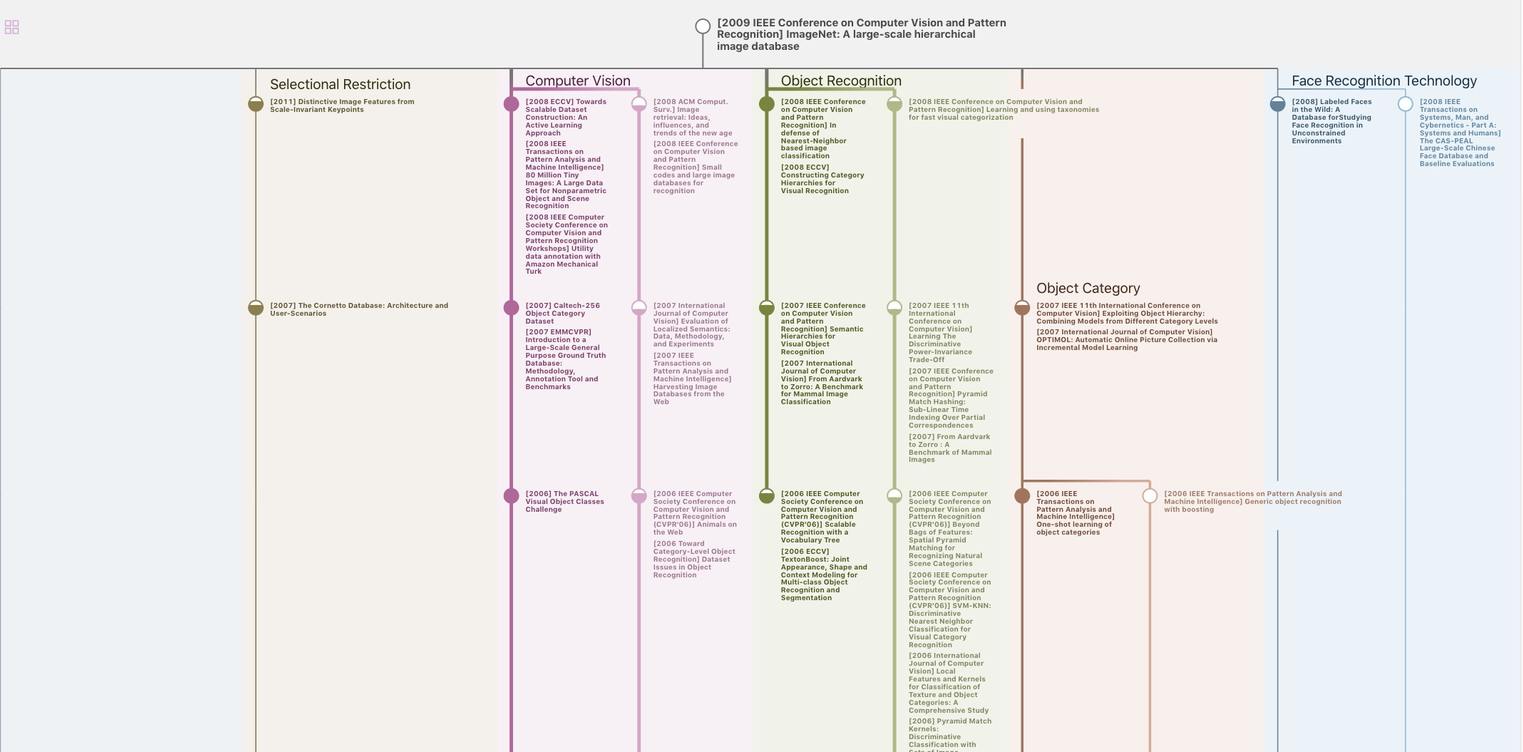
生成溯源树,研究论文发展脉络
Chat Paper
正在生成论文摘要