Probabilistic deep learning approach for targeted hybrid organic-inorganic perovskites
PHYSICAL REVIEW MATERIALS(2021)
摘要
We develop a probabilistic machine learning model and use it to screen for new hybrid organic-inorganic perovskites (HOIPs) with targeted electronic band gap. The data set used for this work is highly diverse, containing multiple atomic structures for each of 192 chemically distinct HOIP formulas. Therefore, any property prediction on a given formula must be associated with an irreducible "uncertainty" that comes from its unknown atomic details. As a result, dozens of new HOIP formulas with band gap falling between 1.25 and 1.50 eV were identified and validated against suitable first-principles computations. Through this demonstration we show that the probabilistic deep learning approach is robust, versatile, and can be used to properly quantify this uncertainty. In conclusion, the probabilistic standpoint and approach described herein could be widely useful for the very common and inevitable data uncertainty which is rooted at the incompleteness of information during experiments and/or computations.
更多查看译文
AI 理解论文
溯源树
样例
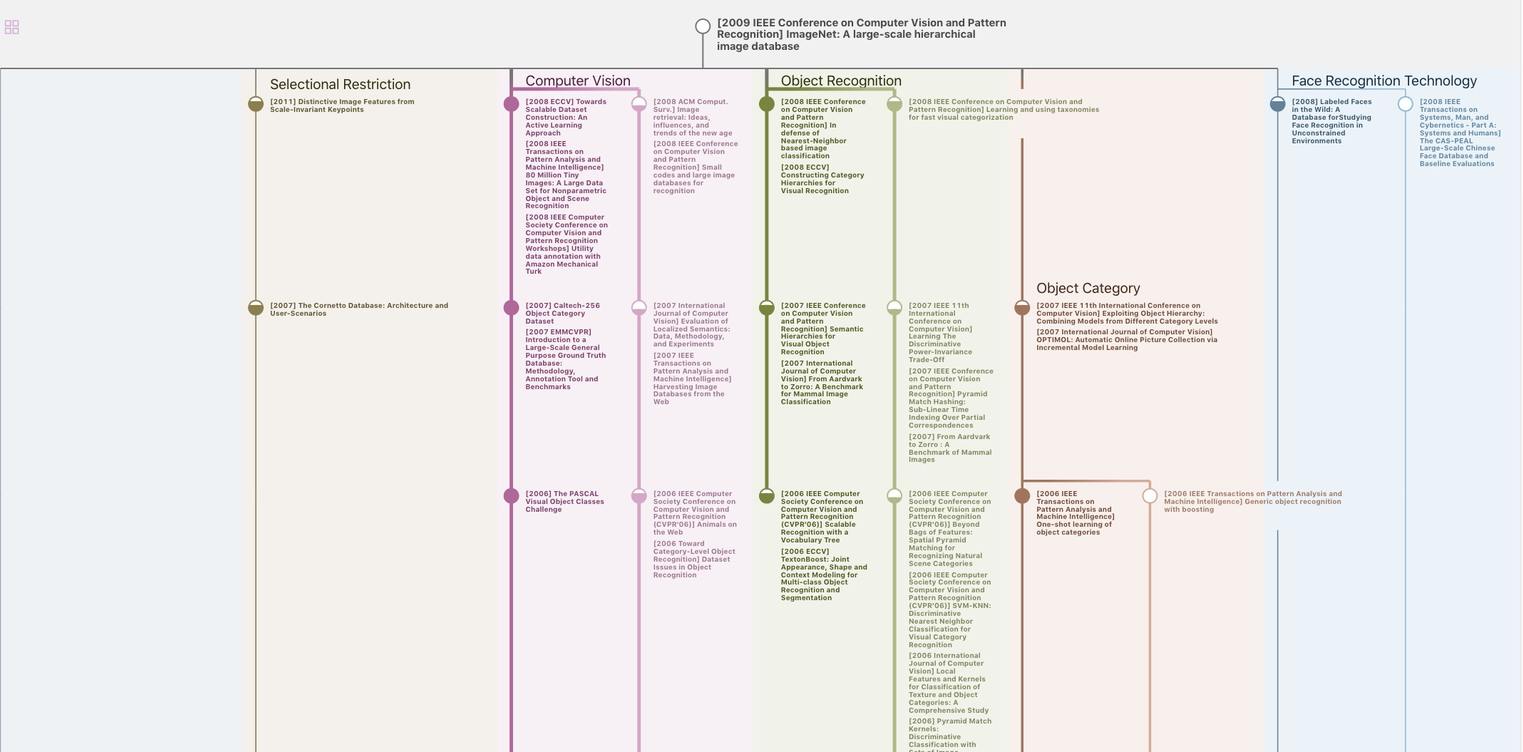
生成溯源树,研究论文发展脉络
Chat Paper
正在生成论文摘要