How to Quantify Polarization in Models of Opinion Dynamics
arxiv(2021)
摘要
It is widely believed that society is becoming increasingly polarized around important issues, a dynamic that does not align with common mathematical models of opinion formation in social networks. In particular, measures of polarization based on opinion variance are known to decrease over time in frameworks such as the popular DeGroot model. Complementing recent work that seeks to resolve this apparent inconsistency by modifying opinion models, we instead resolve the inconsistency by proposing changes to how polarization is quantified. We present a natural class of group-based polarization measures that capture the extent to which opinions are clustered into distinct groups. Using theoretical arguments and empirical evidence, we show that these group-based measures display interesting, non-monotonic dynamics, even in the simple DeGroot model. In particular, for many natural social networks, group-based metrics can increase over time, and thereby correctly capture perceptions of increasing polarization. Our results build on work by DeMarzo et al., who introduced a group-based polarization metric based on ideological alignment. We show that a central tool from that work, a limit analysis of individual opinions under the DeGroot model, can be extended to the dynamics of other group-based polarization measures, including established statistical measures like bimodality. We also consider local measures of polarization that operationalize how polarization is perceived in a network setting. In conjunction with evidence from prior work that group-based measures better align with real-world perceptions of polarization, our work provides formal support for the use of these measures in place of variance-based polarization in future studies of opinion dynamics.
更多查看译文
关键词
quantify polarization,models
AI 理解论文
溯源树
样例
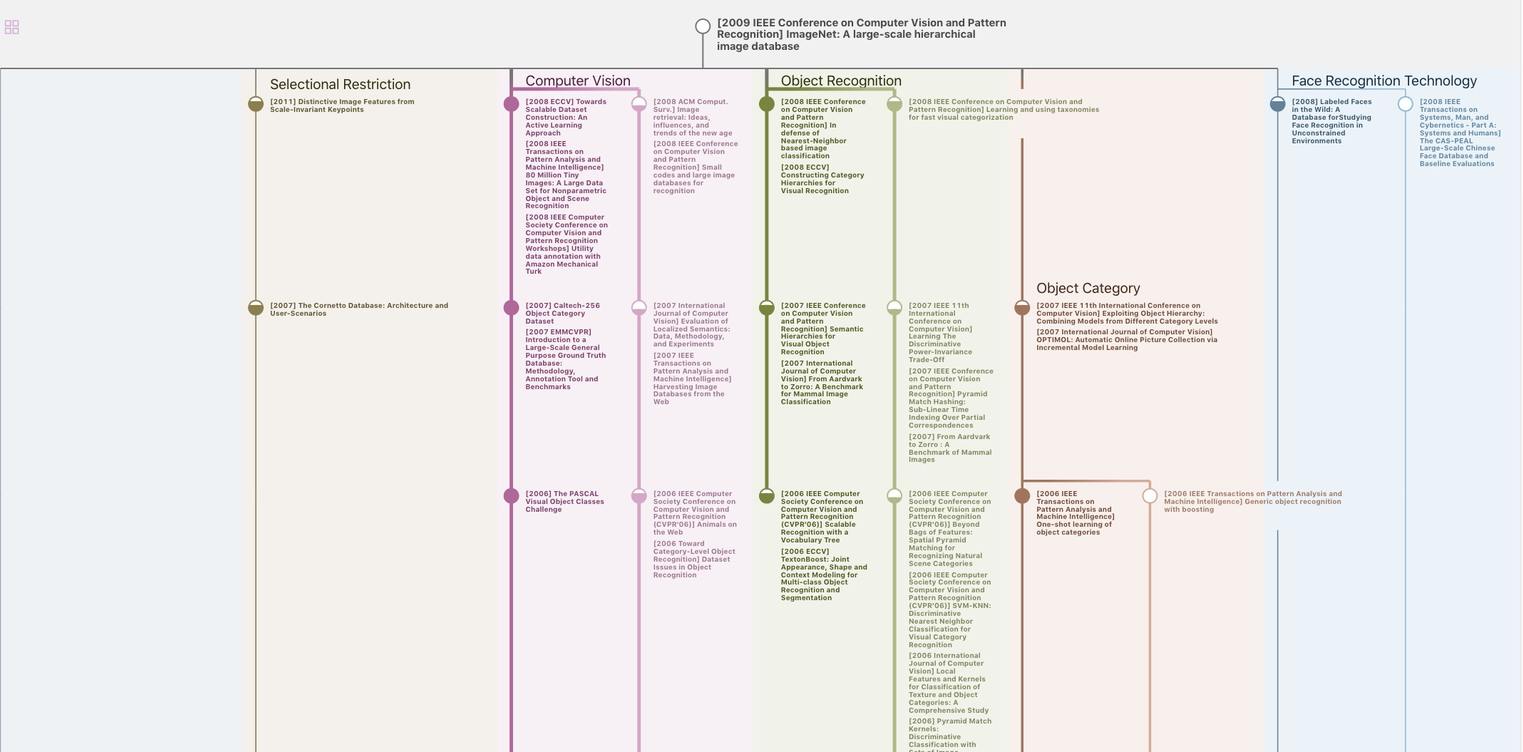
生成溯源树,研究论文发展脉络
Chat Paper
正在生成论文摘要