Adaptive Fusion Affinity Graph with Noise-free Online Low-rank Representation for Natural Image Segmentation
arxiv(2021)
摘要
Affinity graph-based segmentation methods have become a major trend in computer vision. The performance of these methods relies on the constructed affinity graph, with particular emphasis on the neighborhood topology and pairwise affinities among superpixels. Due to the advantages of assimilating different graphs, a multi-scale fusion graph has a better performance than a single graph with single-scale. However, these methods ignore the noise from images which influences the accuracy of pairwise similarities. Multi-scale combinatorial grouping and graph fusion also generate a higher computational complexity. In this paper, we propose an adaptive fusion affinity graph (AFA-graph) with noise-free low-rank representation in an online manner for natural image segmentation. An input image is first over-segmented into superpixels at different scales and then filtered by the proposed improved kernel density estimation method. Moreover, we select global nodes of these superpixels on the basis of their subspace-preserving presentation, which reveals the feature distribution of superpixels exactly. To reduce time complexity while improving performance, a sparse representation of global nodes based on noise-free online low-rank representation is used to obtain a global graph at each scale. The global graph is finally used to update a local graph which is built upon all superpixels at each scale. Experimental results on the BSD300, BSD500, MSRC, SBD, and PASCAL VOC show the effectiveness of AFA-graph in comparison with state-of-the-art approaches.
更多查看译文
关键词
adaptive fusion affinity graph,segmentation,noise-free,low-rank
AI 理解论文
溯源树
样例
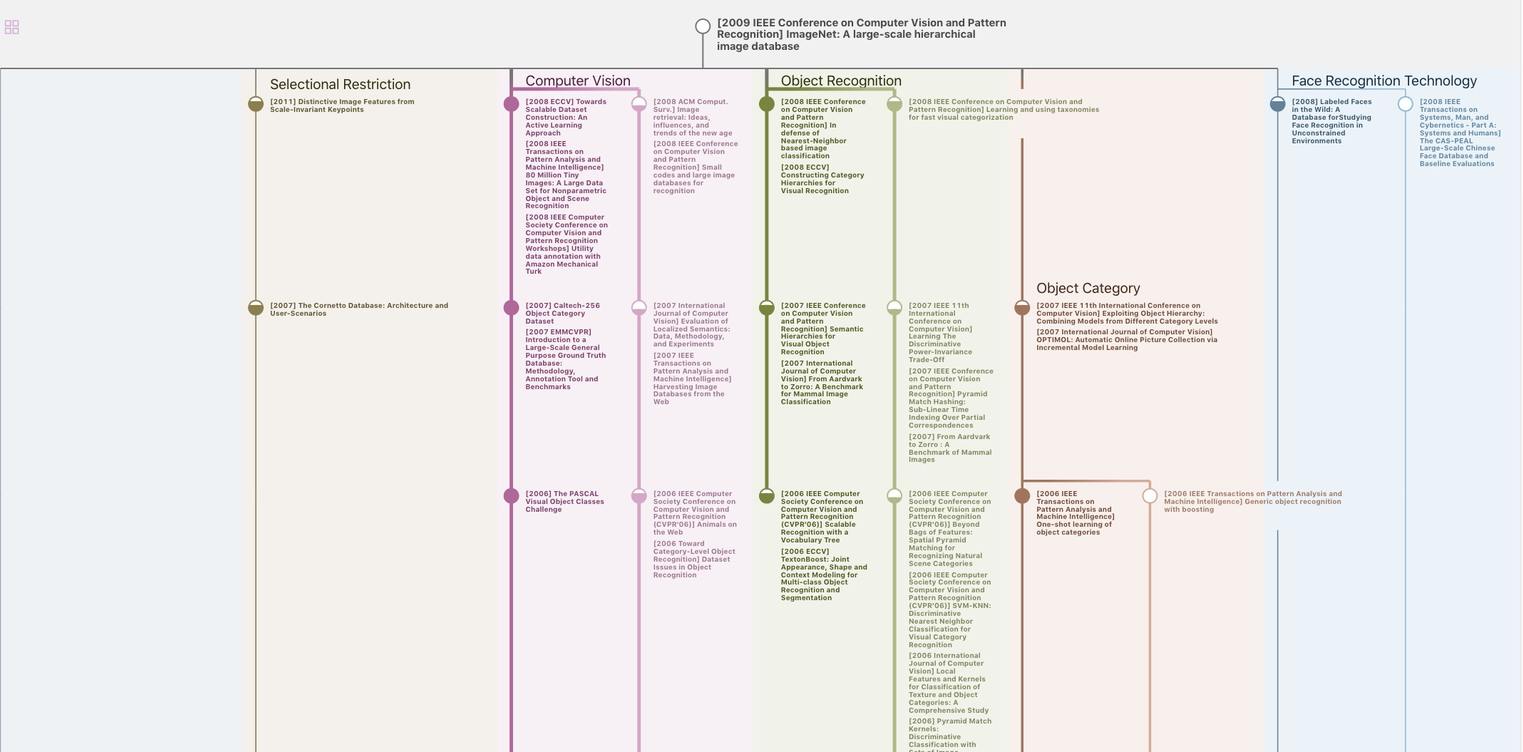
生成溯源树,研究论文发展脉络
Chat Paper
正在生成论文摘要