Exploring Contextual-Aware Representation and Linguistic-Diverse Expression for Visual Dialog
International Multimedia Conference(2021)
摘要
ABSTRACTVisual dialog is a fundamental vision-language task where an AI agent holds a meaningful dialogue about visual content with humans in nature. However, this task remains challenging, since there is still no consensus way to capture rich visual contextual information contained in the environment rather than only focusing on visual objects. Furthermore, conventional methods suffer from the single-answer learning strategy, where it only accepts one correct answer without considering the diverse expressions of the language (i.e., one identical meaning but multiple expressions via rephrasing or adopting synonyms etc). In this paper, we introduce Contextual-Aware Representation and linguistic-diverse Expression (CARE), a novel plug-and-play framework with contextual-based graph embedding and curriculum contrastive learning to solve the above two issues. Specifically, the contextual-based graph embedding (CGE) module aims to integrate the environmental context information with visual objects to improve the answer quality. In addition, we propose a curriculum contrastive learning (CCL) paradigm to imitate the learning habits of humans when facing a question with multiple correct answers sharing the same meaning but with diverse expressions. To support CCL, a CCL loss is designed to progressively strengthen the model's ability in identifying the answers with correct semantics. Extensive experiments are conducted on two benchmark datasets, and our proposed method outperforms the state-of-the-arts by a considerable margin on VisDial V1.0 (4.63% NDCG) and VisDial V0.9 (1.27% MRR, 1.74% [email protected], 0.87% [email protected], 1.28% [email protected], 0.26 Mean.
更多查看译文
AI 理解论文
溯源树
样例
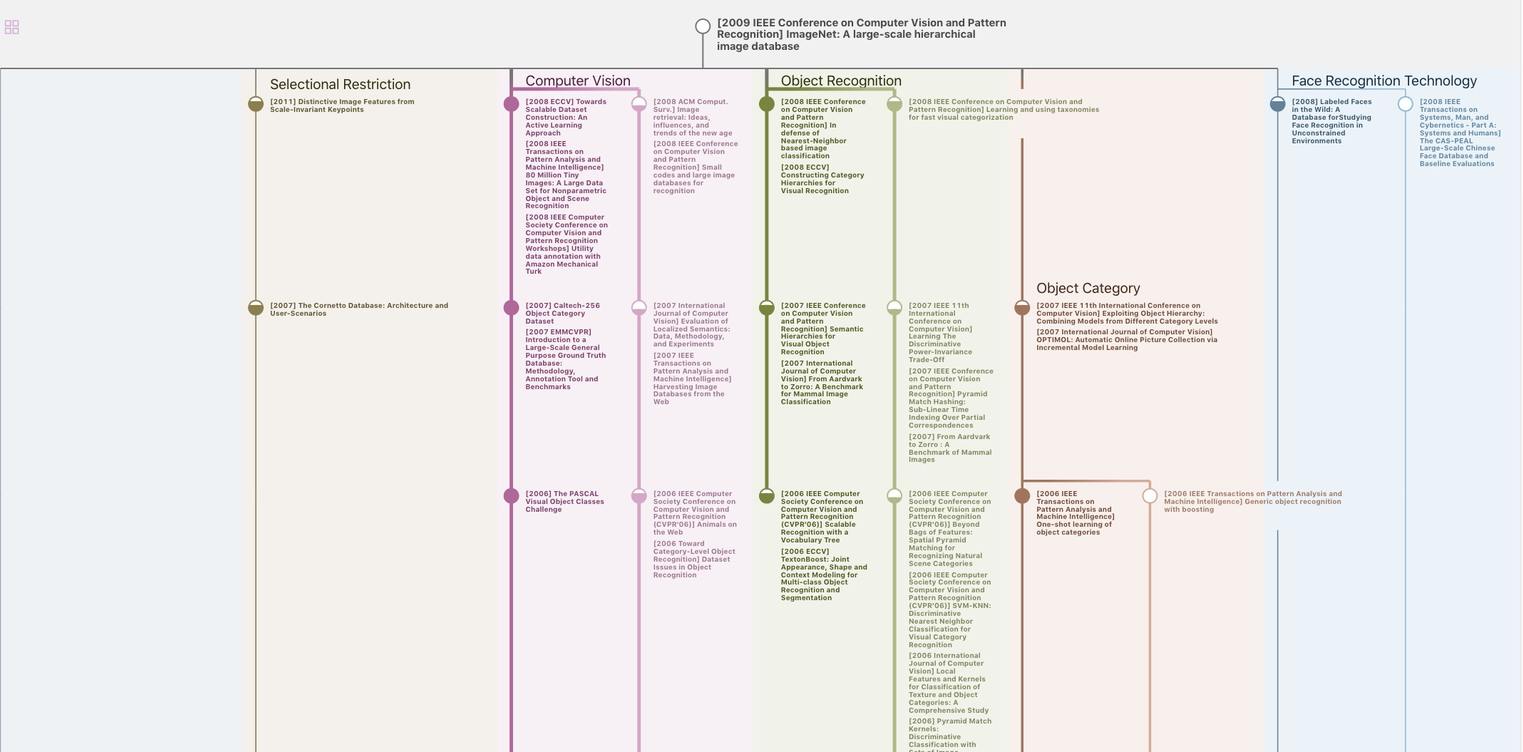
生成溯源树,研究论文发展脉络
Chat Paper
正在生成论文摘要