Self-learning Visual Servoing for Robot Manipulation in Unstructured Environments
INTELLIGENT ROBOTICS AND APPLICATIONS, ICIRA 2021, PT II(2021)
摘要
Current visual servoing methods used in robot manipulation require system modeling and parameters, only working in structured environments. This paper presents a self-learning visual servoing for a robot manipulator operated in unstructured environments. A Gaussian-mapping likelihood process is used in Bayesian stochastic state estimation (SSE) for Robotic coordination control, in which the Monte Carlo sequential importance sampling (MCSIS) algorithm is created for robotic visual-motor mapping estimation. The Bayesian learning strategy described takes advantage of restraining the particles deterioration to maintain the robot robust performance. Additionally, the servoing controller is deduced for robotic coordination directly by visual observation. The proposed visual servoing framework is applied to a manipulator with eye-in-hand configuration no system parameters. Finally, the simulation and experimental results demonstrate consistently that the proposed algorithm outperforms traditional visual servoing approaches.
更多查看译文
关键词
Bayesian filtering, Gaussian-mapping estimation, Visual servoing
AI 理解论文
溯源树
样例
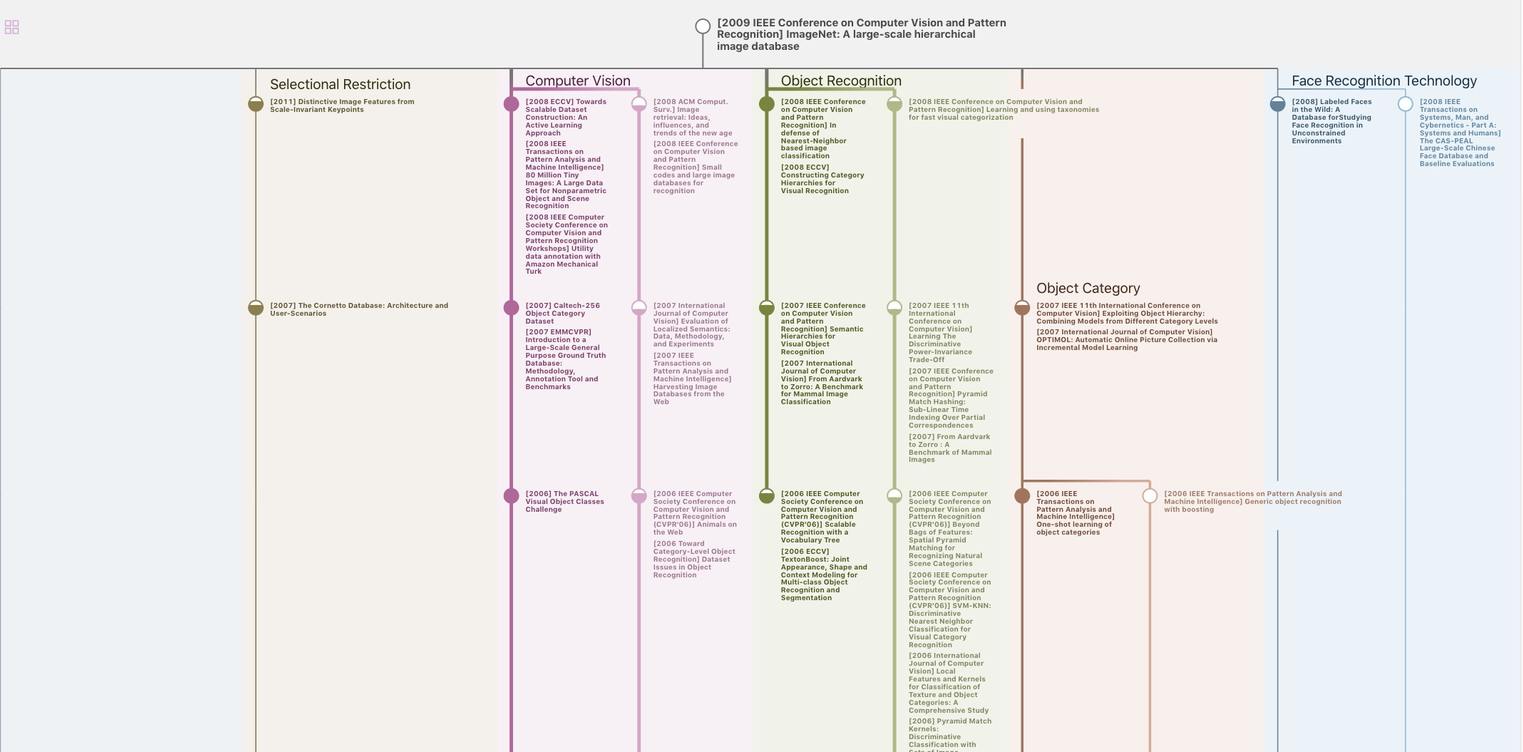
生成溯源树,研究论文发展脉络
Chat Paper
正在生成论文摘要