DLOW: Domain Flow and Applications
INTERNATIONAL JOURNAL OF COMPUTER VISION(2021)
摘要
In this work, we present a domain flow generation (DLOW) model to bridge two different domains by generating a continuous sequence of intermediate domains flowing from one domain to the other. The benefits of our DLOW model are twofold. First, it is able to transfer source images into a domain flow, which consists of images with smoothly changing distributions from the source to the target domain. The domain flow bridges the gap between source and target domains, thus easing the domain adaptation task. Second, when multiple target domains are provided for training, our DLOW model is also able to generate new styles of images that are unseen in the training data. The new images are shown to be able to mimic different artists to produce a natural blend of multiple art styles. Furthermore, for the semantic segmentation in the adverse weather condition, we take advantage of our DLOW model to generate images with gradually changing fog density, which can be readily used for boosting the segmentation performance when combined with a curriculum learning strategy. We demonstrate the effectiveness of our model on benchmark datasets for different applications, including cross-domain semantic segmentation, style generalization, and foggy scene understanding. Our implementation is available at https://github.com/ETHRuiGong/DLOW .
更多查看译文
关键词
Domain flow,Domain adaptation,Domain generalization,Image translation,Semantic segmentation,Fog generation
AI 理解论文
溯源树
样例
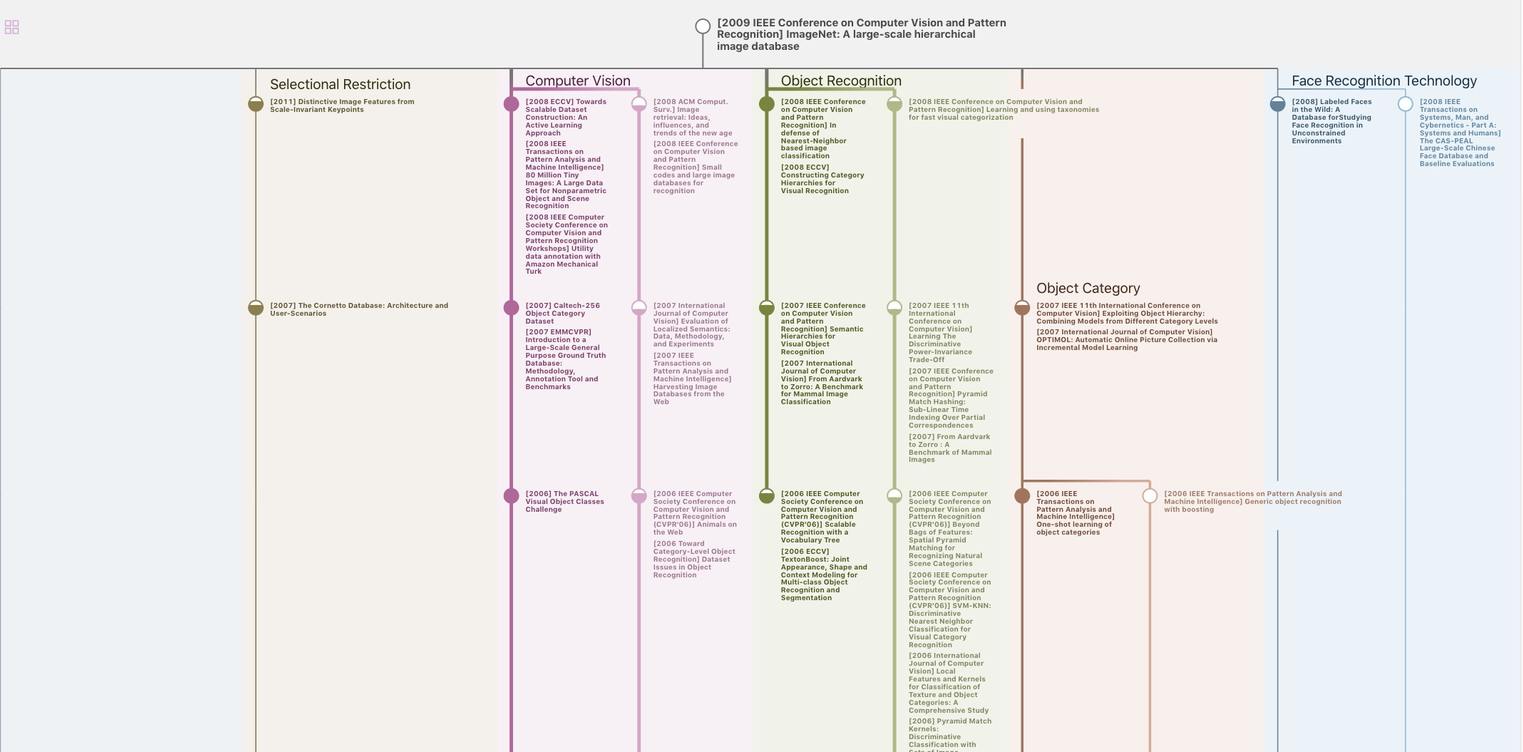
生成溯源树,研究论文发展脉络
Chat Paper
正在生成论文摘要