RL4RS: A Real-World Dataset for Reinforcement Learning based Recommender System
PROCEEDINGS OF THE 46TH INTERNATIONAL ACM SIGIR CONFERENCE ON RESEARCH AND DEVELOPMENT IN INFORMATION RETRIEVAL, SIGIR 2023(2023)
摘要
Reinforcement learning based recommender systems (RL-based RS) aim at learning a good policy from a batch of collected data, by casting recommendations to multi-step decision-making tasks. However, current RL-based RS research commonly has a large reality gap. In this paper, we introduce the first open-source real-world dataset, RL4RS, hoping to replace the artificial datasets and semi-simulated RS datasets previous studies used due to the resource limitation of the RL-based RS domain. Unlike academic RL research, RL-based RS suffers from the difficulties of being well-validated before deployment. We attempt to propose a new systematic evaluation framework, including evaluation of environment simulation, evaluation on environments, and counterfactual policy evaluation. In summary, the RL4RS (Reinforcement Learning for Recommender Systems), a new resource with special concerns on the reality gaps, contains two real-world datasets, data understanding tools, tuned simulation environments, related advanced RL baselines, batch RL baselines, and counterfactual policy evaluation algorithms. The RL4RS suite can be found at https:// github.com/ fuxiAIlab/
更多查看译文
关键词
datasets,recommender systems,applied reinforcement learning
AI 理解论文
溯源树
样例
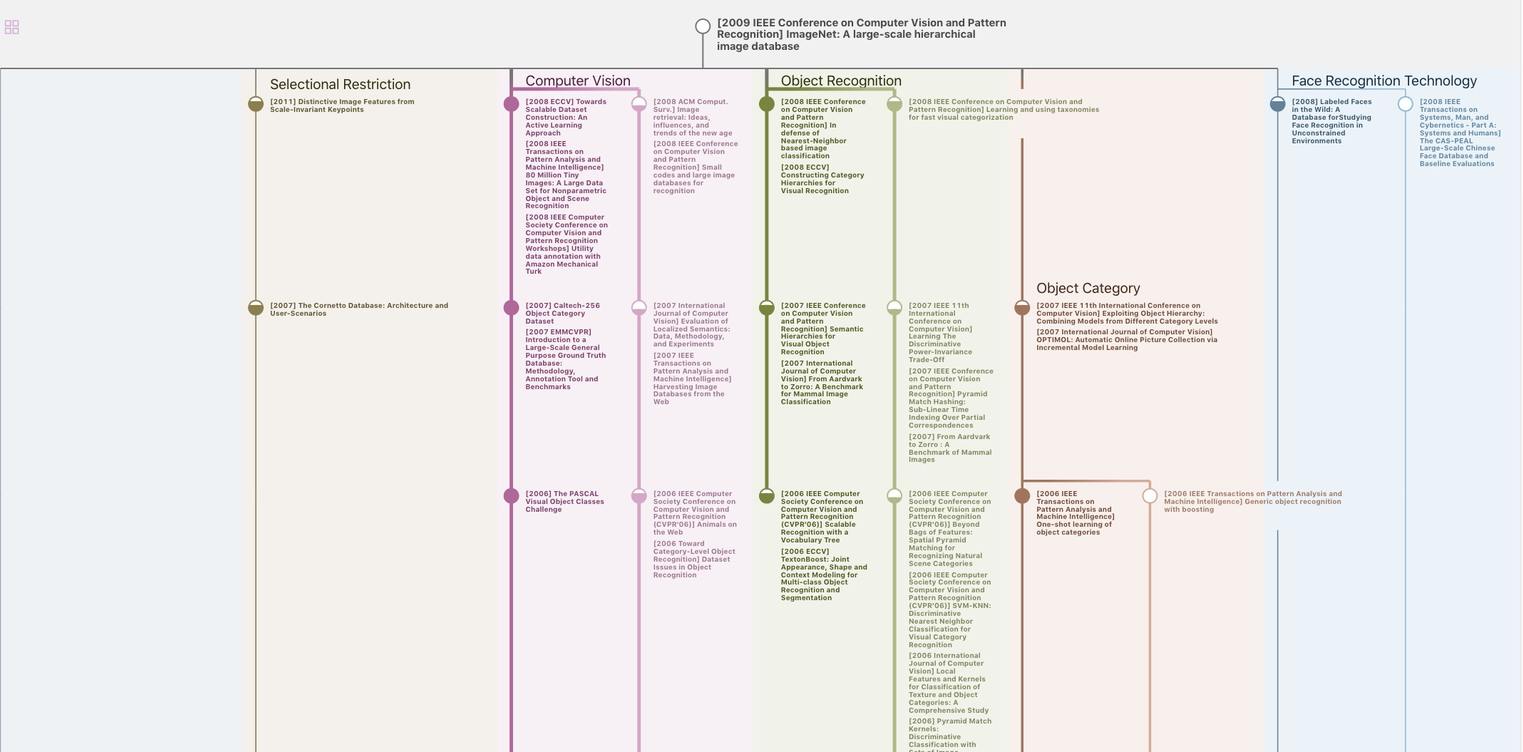
生成溯源树,研究论文发展脉络
Chat Paper
正在生成论文摘要