SMOF: Squeezing More Out of Filters Yields Hardware-Friendly CNN Pruning
arxiv(2021)
摘要
For many years, the family of convolutional neural networks (CNNs) has been a workhorse in deep learning. Recently, many novel CNN structures have been designed to address increasingly challenging tasks. To make them work efficiently on edge devices, researchers have proposed various structured network pruning strategies to reduce their memory and computational cost. However, most of them only focus on reducing the number of filter channels per layer without considering the redundancy within individual filter channels. In this work, we explore pruning from another dimension, the kernel size. We develop a CNN pruning framework called SMOF, which Squeezes More Out of Filters by reducing both kernel size and the number of filter channels. Notably, SMOF is friendly to standard hardware devices without any customized low-level implementations, and the pruning effort by kernel size reduction does not suffer from the fixed-size width constraint in SIMD units of general-purpose processors. The pruned networks can be deployed effortlessly with significant running time reduction. We also support these claims via extensive experiments on various CNN structures and general-purpose processors for mobile devices.
更多查看译文
关键词
CNN, Kernel size, Pruning
AI 理解论文
溯源树
样例
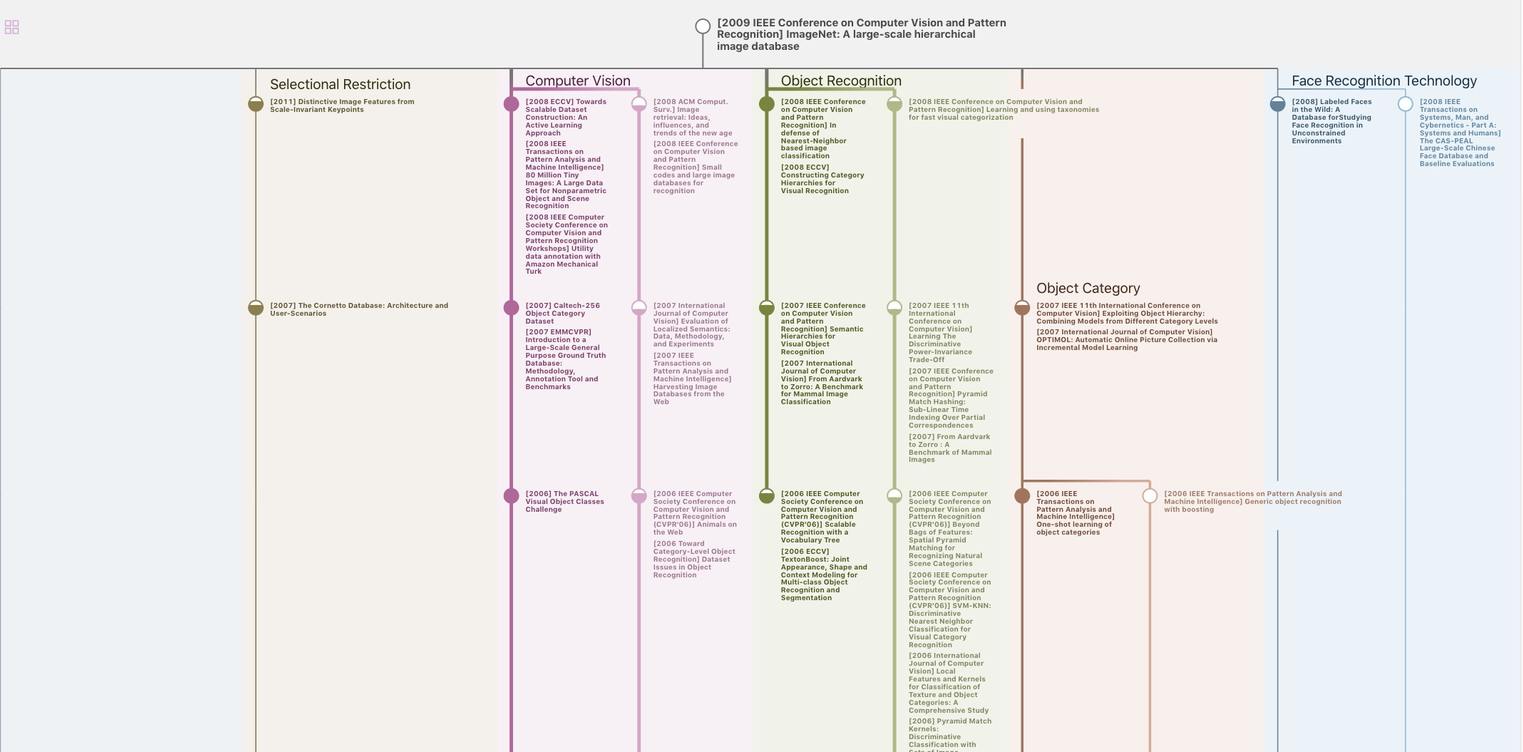
生成溯源树,研究论文发展脉络
Chat Paper
正在生成论文摘要