Deep Point Cloud Normal Estimation Via Triplet Learning
IEEE International Conference on Multimedia and Expo (ICME)(2022)
摘要
Normal estimation on 3D point clouds is a fundamental problem in 3D vision and graphics. Current methods often show limited accuracy in predicting normals at sharp features (e.g., edges and corners) and less robustness to noise. In this paper, we propose a novel normal estimation method for point clouds. It consists of two phases: (a) feature encoding which learns representations of local patches, and (b) normal estimation that takes the learned representation as input and regresses the normal vector. We are motivated that local patches on isotropic and anisotropic surfaces have similar or distinct normals, and that separable features or representations can be learned to facilitate normal estimation. To realise this, we first construct triplets of local patches on 3D point cloud data, and design a triplet network with a triplet loss for feature encoding. We then design a simple network with several MLPs and a loss function to regress the normal vector. Despite having a smaller network size compared to most other methods, experimental results show that our method preserves sharp features and achieves better normal estimation results on CAD-like shapes.
更多查看译文
关键词
3D Point Clouds,Normal Estimation
AI 理解论文
溯源树
样例
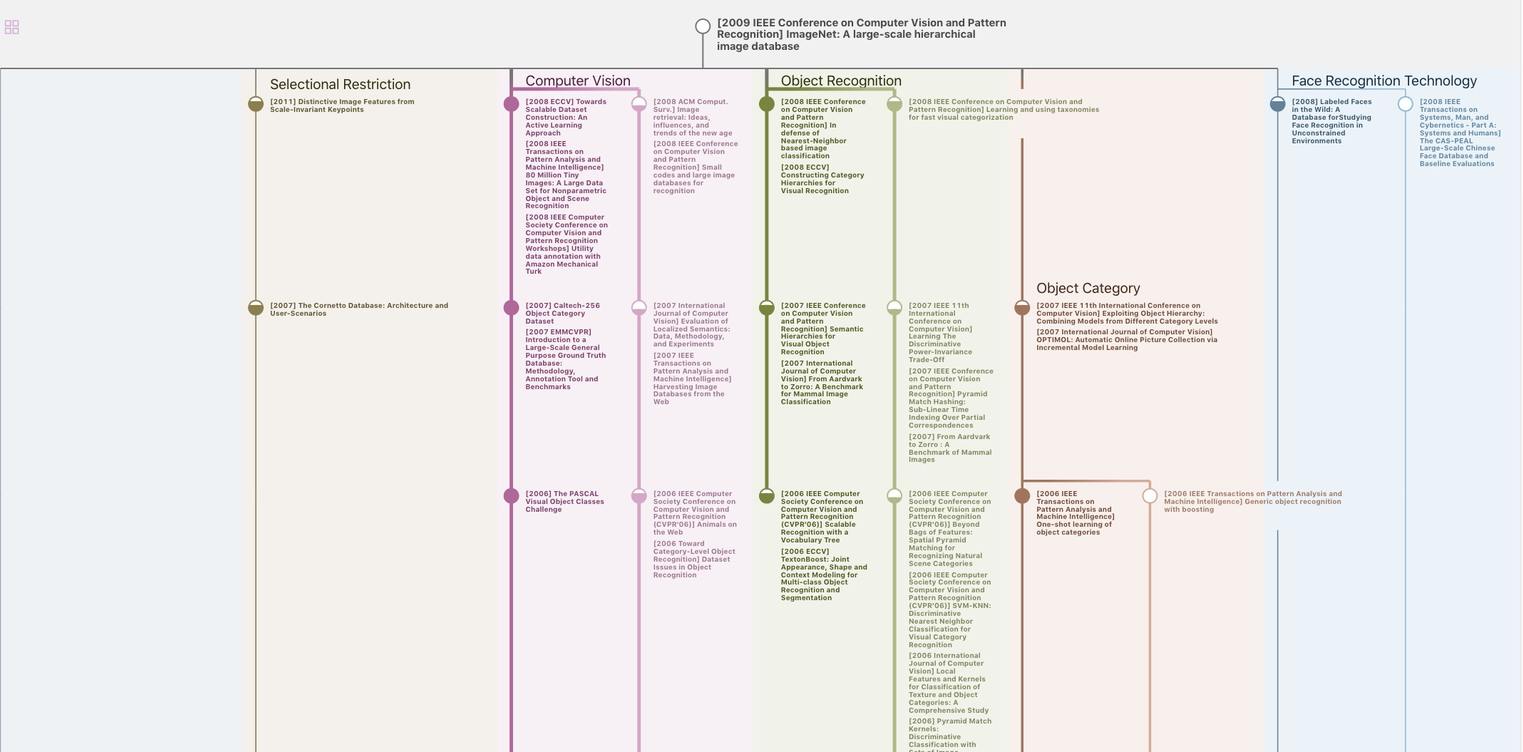
生成溯源树,研究论文发展脉络
Chat Paper
正在生成论文摘要