Addressing positivity violations in causal effect estimation using Gaussian process priors.
Statistics in medicine(2023)
摘要
In observational studies, causal inference relies on several key identifying assumptions. One identifiability condition is the positivity assumption, which requires the probability of treatment be bounded away from 0 and 1. That is, for every covariate combination, it should be possible to observe both treated and control subjects the covariate distributions should overlap between treatment arms. If the positivity assumption is violated, population-level causal inference necessarily involves some extrapolation. Ideally, a greater amount of uncertainty about the causal effect estimate should be reflected in such situations. With that goal in mind, we construct a Gaussian process model for estimating treatment effects in the presence of practical violations of positivity. Advantages of our method include minimal distributional assumptions, a cohesive model for estimating treatment effects, and more uncertainty associated with areas in the covariate space where there is less overlap. We assess the performance of our approach with respect to bias and efficiency using simulation studies. The method is then applied to a study of critically ill female patients to examine the effect of undergoing right heart catheterization.
更多查看译文
关键词
Bayesian nonparametrics,Metropolis within Gibbs,causal inference,extrapolation,overlap,population average treatment effect
AI 理解论文
溯源树
样例
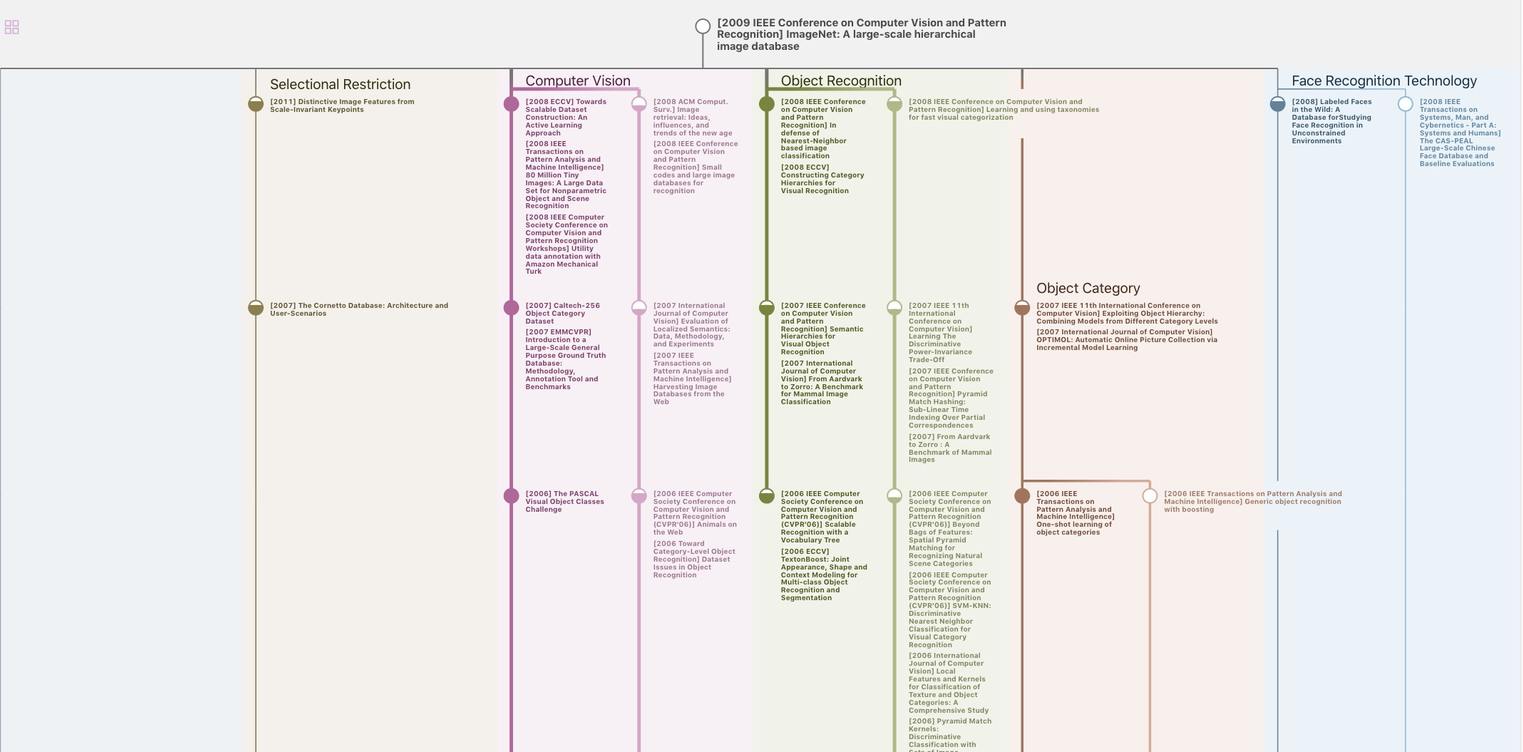
生成溯源树,研究论文发展脉络
Chat Paper
正在生成论文摘要