Forecasting Market Prices using DL with Data Augmentation and Meta-learning: ARIMA still wins!
arxiv(2021)
摘要
Deep-learning techniques have been successfully used for time-series forecasting and have often shown superior performance on many standard benchmark datasets as compared to traditional techniques. Here we present a comprehensive and comparative study of performance of deep-learning techniques for forecasting prices in financial markets. We benchmark state-of-the-art deep-learning baselines, such as NBeats, etc., on data from currency as well as stock markets. We also generate synthetic data using a fuzzy-logic based model of demand driven by technical rules such as moving averages, which are often used by traders. We benchmark the baseline techniques on this synthetic data as well as use it for data augmentation. We also apply gradient-based meta-learning to account for non-stationarity of financial time-series. Our extensive experiments notwithstanding, the surprising result is that the standard ARIMA models outperforms deep-learning even using data augmentation or meta-learning. We conclude by speculating as to why this might be the case.
更多查看译文
关键词
forecasting,arima,market,data augmentation,meta-learning
AI 理解论文
溯源树
样例
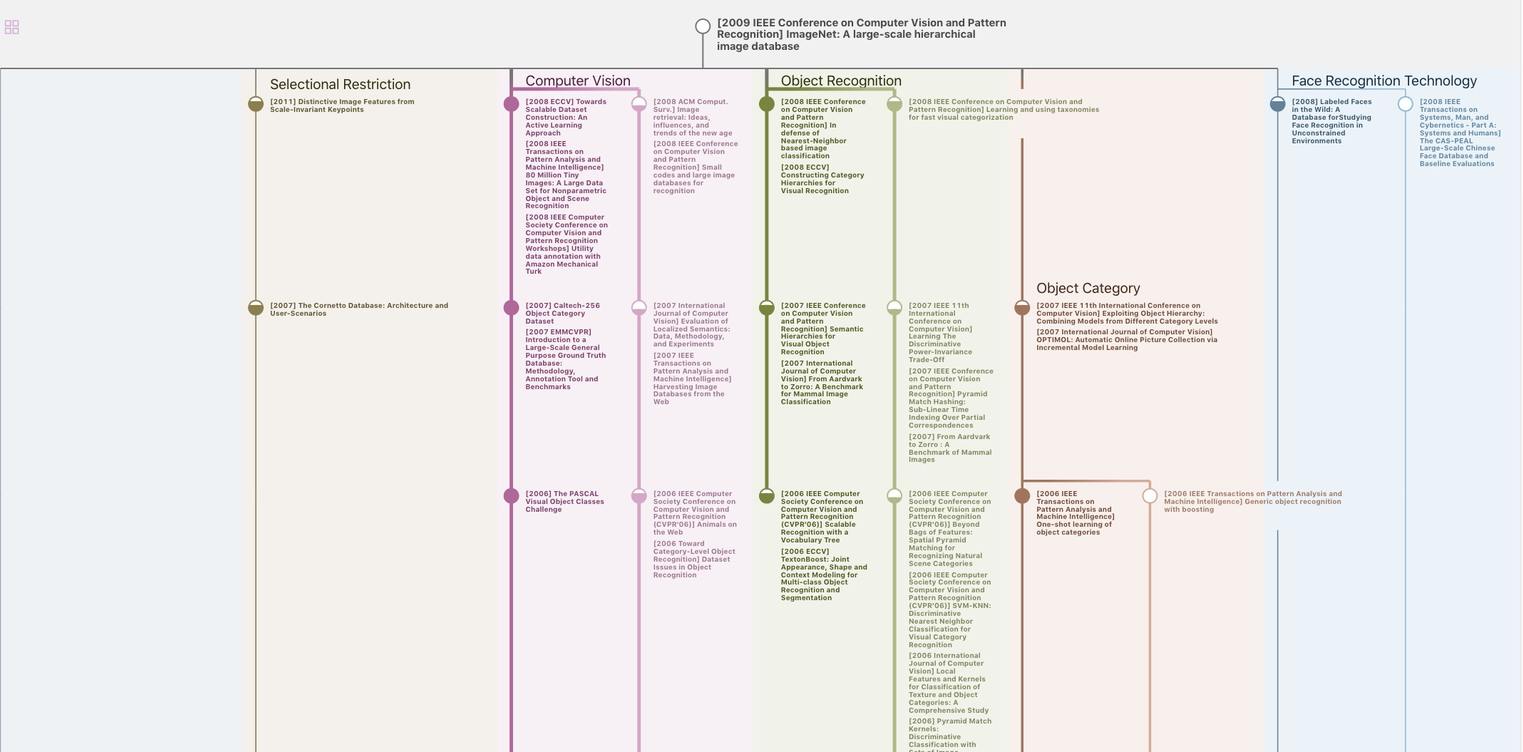
生成溯源树,研究论文发展脉络
Chat Paper
正在生成论文摘要