Weakly Supervised Solar Panel Mapping Using Residual Aggregated Network for Aerial Images.
IGARSS(2021)
摘要
With the rapid development of solar distribution, mapping the locations and sizes of solar panels is becoming increasingly valuable. Weakly supervised methods have been proposed to reduce the reliance on expensive pixel-wise annotations by adopting weak labels, but inevitably suffer from low mapping accuracy and poor boundary maintenance. To solve these problems, a novel weakly supervised residual aggregated network (WS-RAN) is presented in this paper. In the WS-RAN, pixel-wise labels are automatically generated from image-level labels by a classification network. Then, the produced pixel-wise labels are used to train the residual aggregated network (RAN) for target mapping, which is designed to cope the variations in size and shapes of individual solar panel layout. Particularly, for better boundary maintenance, the residual aggregated block is developed as the basic module in the bottom-up path of the RAN. Experiment results reveal that the proposed WS-RAN significantly outperforms four state-of-the-art weakly supervised methods with 76.0% F
1
score and 61.3% IoU score.
更多查看译文
关键词
Solar panel mapping,weakly supervised learning,feature pyramid network,residual aggregated block
AI 理解论文
溯源树
样例
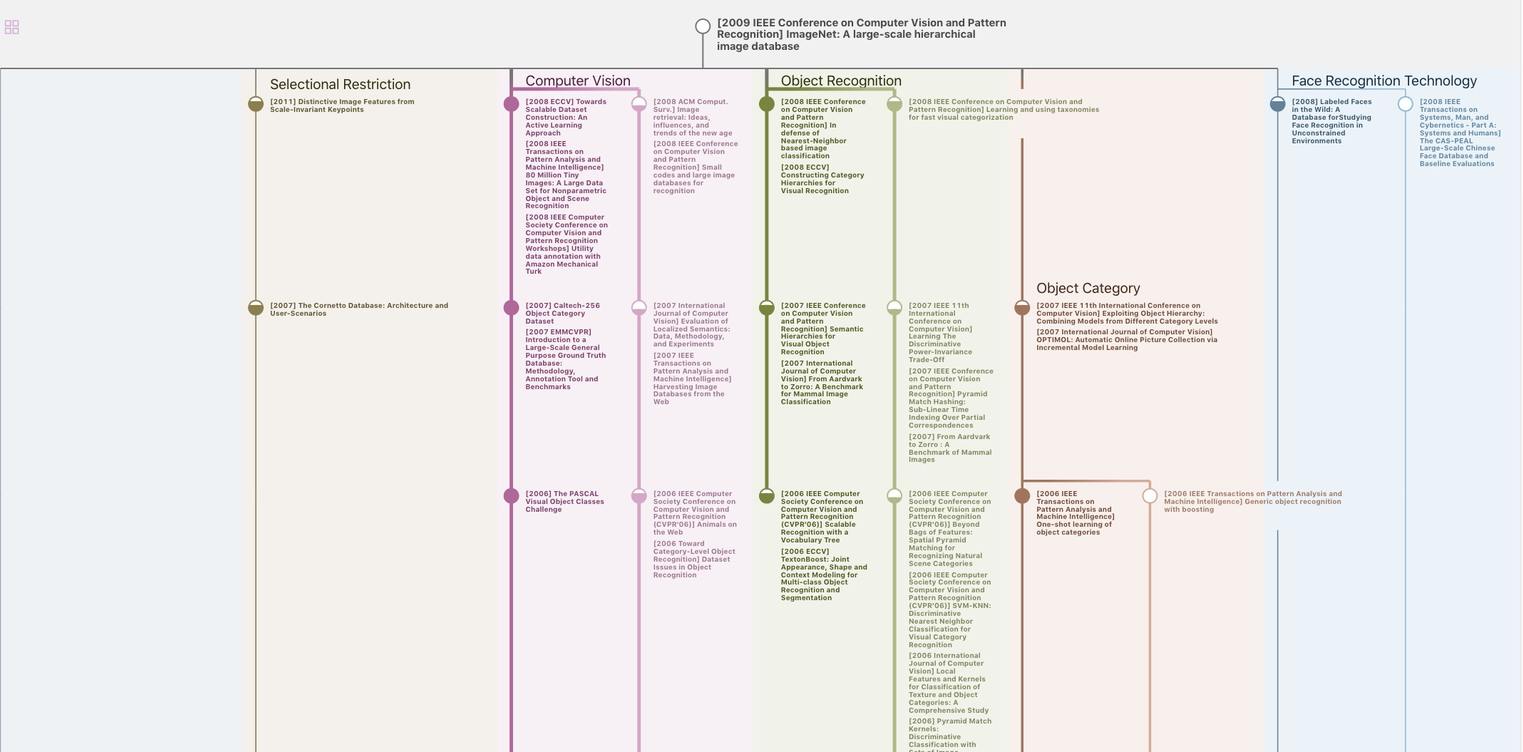
生成溯源树,研究论文发展脉络
Chat Paper
正在生成论文摘要