Spatial-Spectral Tensor Graph Convolutional Network for Hyperspectral Image Classification.
IGARSS(2021)
摘要
Graph convolutional networks (GCNs) have come necessarily into being with the increase of graph-structure data in real world, which recently, have been applied for feature extraction and classification of hyperspectral image (HSI). However, only spatial relationship of pixels being considered by GCN-based models, the relationship between spectral bands are underutilized. To solve above issue, benefit from introducing tensor theory to GCN, a novel spatial-spectral tensor graph convolutional network (SSTGCN) is proposed to learn tensor representation of spatial-spectral feature. Firstly, from the perspective of the spatial-spectral characteristic in HSI, spatial graph tensor and spectral graph tensor are constructed to model the spatial and spectral relationships, respectively. Then, two types of modules, i.e., the spatial tensor graph convolution (SATGC) module and spectral tensor graph convolution (SETGC) module, are designed to extract discriminative features. Experimental results on the Indian Pines data set demonstrate that the proposed method outperforms other HSI classification methods.
更多查看译文
关键词
Hyperspectral image classification,graph convolution networks,tensor theory,feature extraction,deep learning
AI 理解论文
溯源树
样例
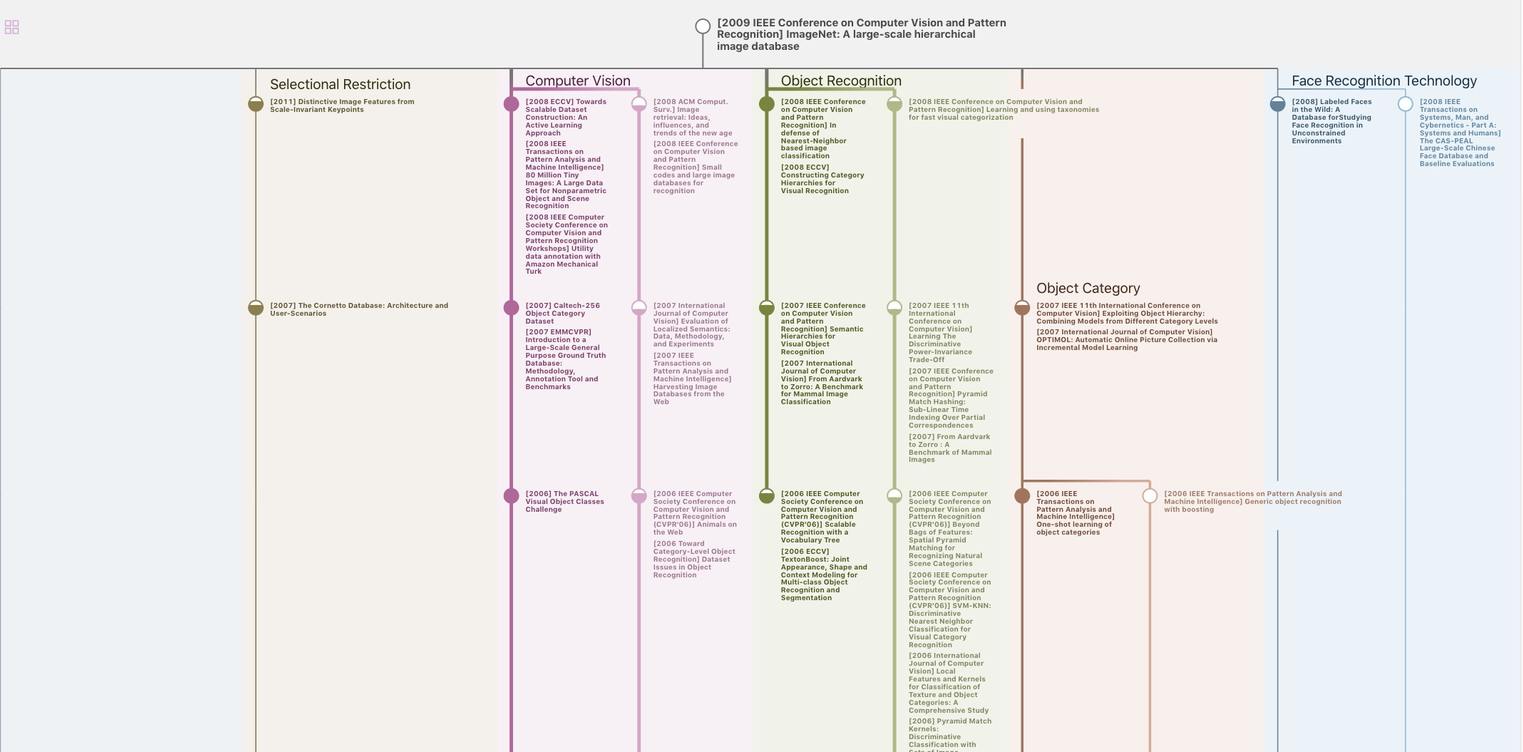
生成溯源树,研究论文发展脉络
Chat Paper
正在生成论文摘要