Bayesian Detection of Solid Subpixel Targets.
IGARSS(2021)
摘要
We implement and evaluate a Bayesian detector for opaque subpixel hyperspectral targets of unknown abundance. Using both simulated and real hyperspectral backgrounds, we compare this detector to the more conventional generalized likelihood ratio test (GLRT) approach, identifying theoretical differences and observing numerical similarities. Among the theoretical advantages provided by the Bayesian detector is admissibility, which means that no detector can be uniformly superior to it. Potential disadvantages include the need to choose a prior distribution, and the computation required to integrate that distribution. For solid subpixel targets, the uniform prior is a natural choice, and we find that adequately-accurate numerical integration can be achieved with only a few evaluations of the likelihood function. We show results for targets implanted in both simulated and real data.
更多查看译文
关键词
Algorithm,Hyperspectral imagery,Target detection,Likelihood ratio,Composite hypothesis testing,Bayes,GLRT,Multivariate $t$ distribution
AI 理解论文
溯源树
样例
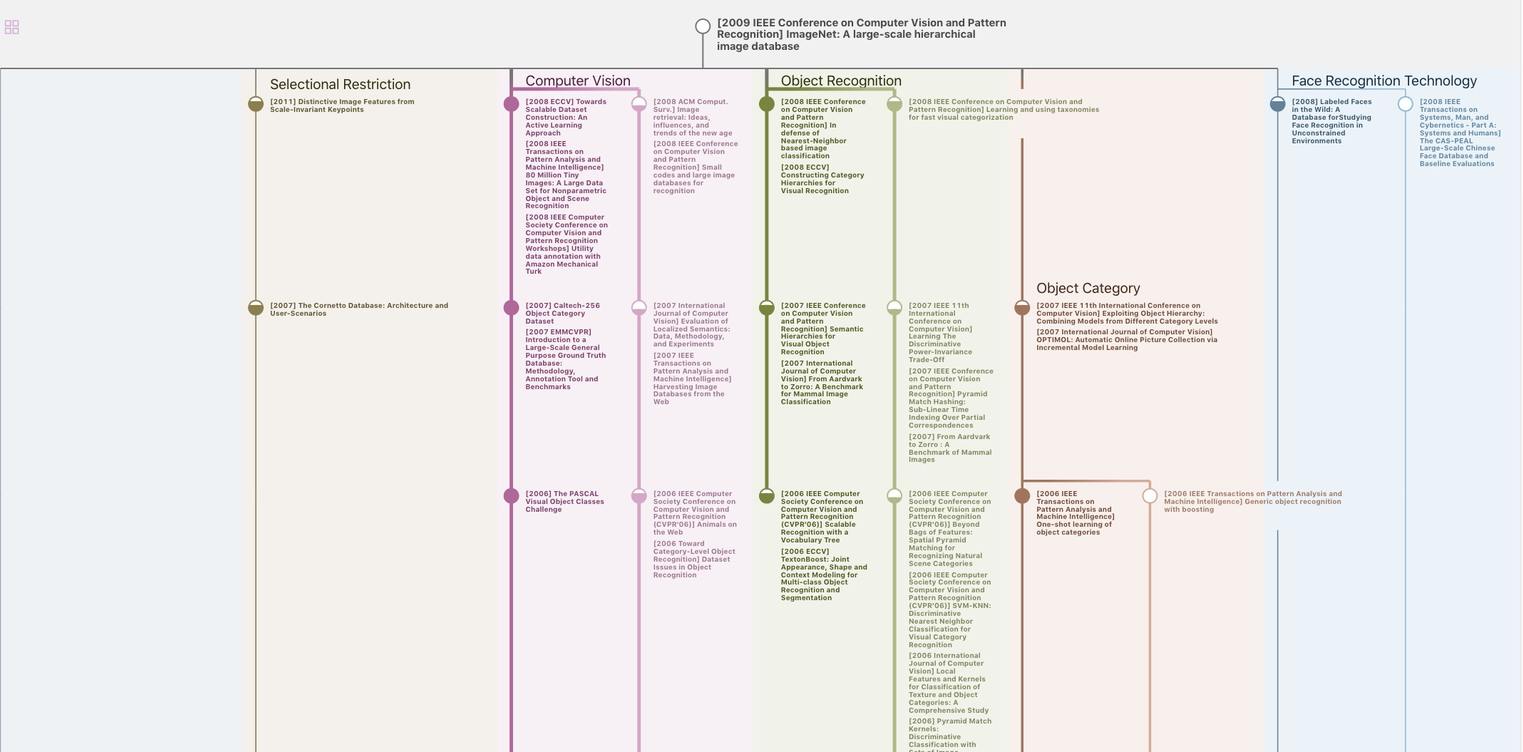
生成溯源树,研究论文发展脉络
Chat Paper
正在生成论文摘要