Himawari Thermal Anomaly Scrutiny with Deep Learning.
IGARSS(2021)
摘要
In the presented article, machine learning (ML) is employed on advanced Himawari imager (AHI) to examine real-time fire and map damaged zone over Yunnan, China. The main emphasis lies in employing machine learning as an alternative to primeval thresholding, extricating, and scrutinizing thermal anomaly using infrared (IR). Firstly, Himawari brightness temperature (BT), band ratio, albedo, and BT differences are utilized to scrutinize fire break out. Then, to ensure pixels are clear and free from clouds, the Himawari cloud product is implemented. Finally, machine learning models such as random forest (RF), artificial neural network (ANN), and time-series long short-term memory (LSTM), a deep learning model, are used to precisely classify the active fire pixels and achieved accuracies of 0.96%, 0.95%, 0.92% respectively. The results evaluated using another AHI wildfire product and inter-compared with multi-source fire products.
更多查看译文
关键词
Active fire,Brightness temperature,Burn scar mapping,Machine,Deep learning
AI 理解论文
溯源树
样例
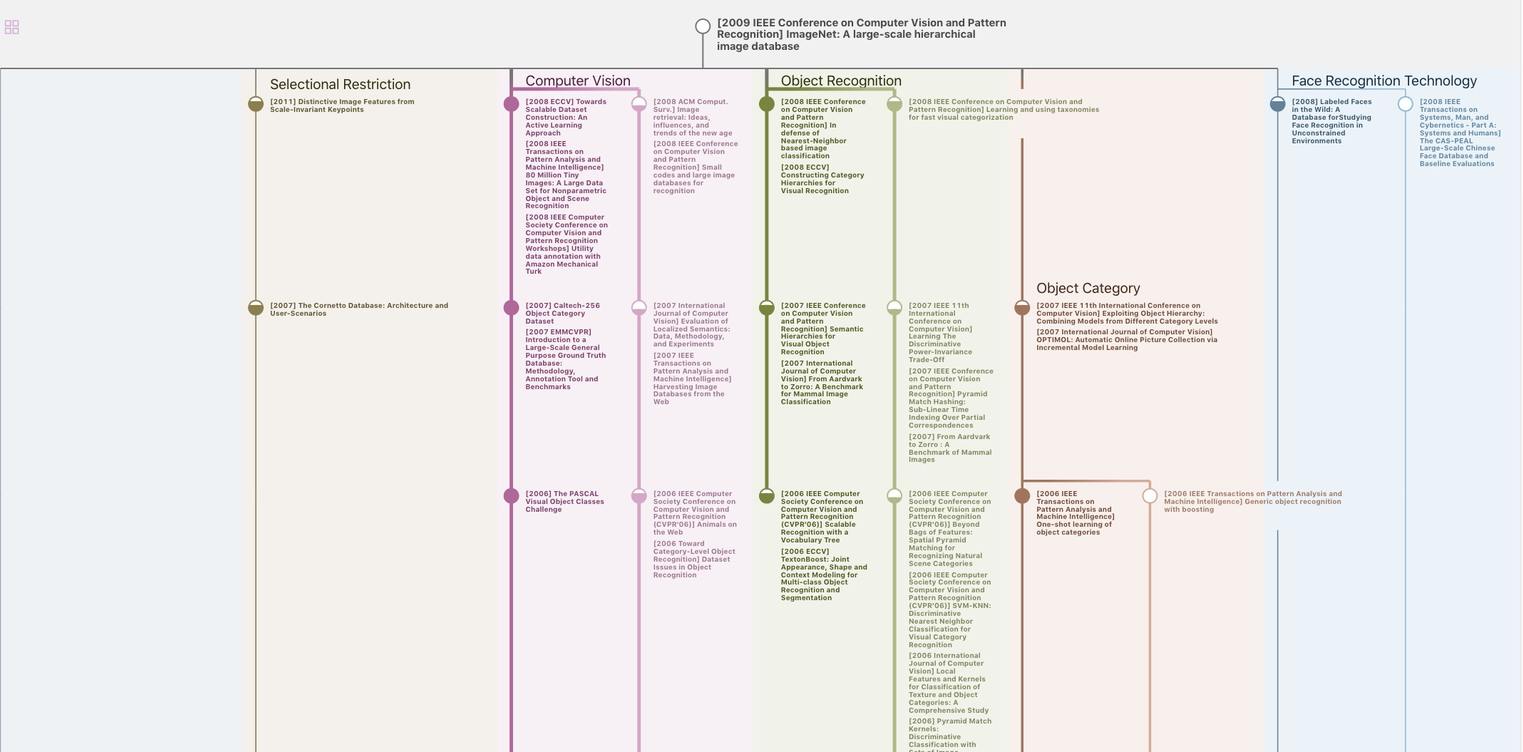
生成溯源树,研究论文发展脉络
Chat Paper
正在生成论文摘要