Exploring Temporal Context at Multiple Scales for Crop Mapping with Fully Convolutional Recurrent Nets and Fully Connected CRFS.
IGARSS(2021)
摘要
This paper introduces a novel hybrid N-to-N bidirectional ConvLSTM for multi-temporal crop recognition in areas characterized by highly complex crop dynamics as in tropical regions. The proposed method seeks to explore both spatial and temporal context of the data by adding a bidirectional ConvLSTM to every skip connection in the U-Net and applying the fully connected conditional random fields (CRF) methodology as a post-processing of the predictions. We evaluated our method on a publicly available tropical region dataset, from Sentinel-1 data, obtaining an average improvement of almost 4% in the Average F1 Score and 1.7% in Overall Accuracy, when comparing with a state-of-the art method.
更多查看译文
关键词
Convolutional Recurrent Networks,Fully Convolutional Networks,Recurrent Networks,Crop Recognition,Deep Learning,Remote Sensing
AI 理解论文
溯源树
样例
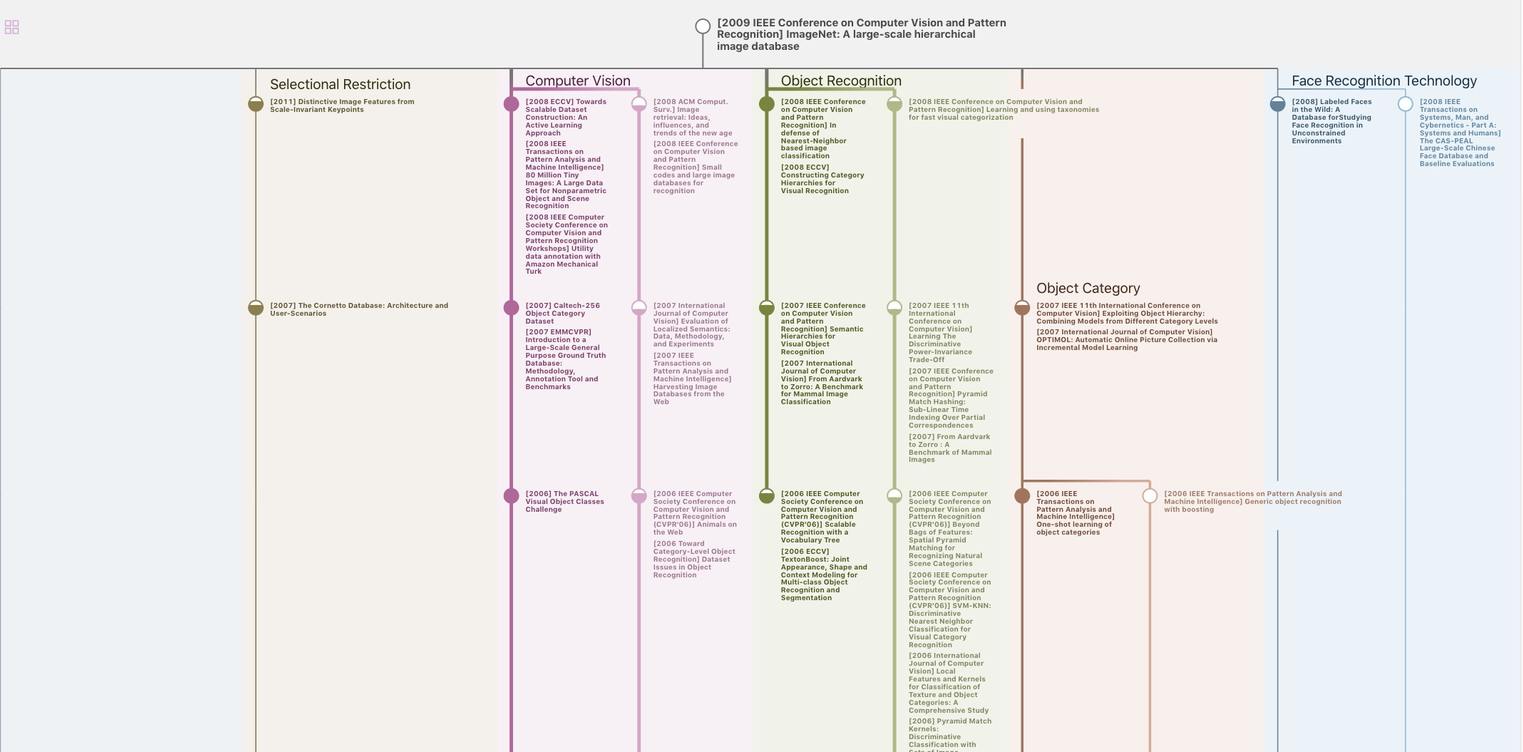
生成溯源树,研究论文发展脉络
Chat Paper
正在生成论文摘要