Influence of Sample Size in Land Cover Classification Accuracy Using Random Forest and Sentinel-2 Data in Portugal.
IGARSS(2021)
摘要
Classification accuracy of remote sensing images with supervised learning depends on the quality and characteristics of training samples. Size is a key aspect of a sample and its impact on classification depends on several factors, including the classifier employed, dimension on the feature space and land cover characteristics. Random Forest classifier is considered to be of low sensitivity to variations in sample size. However, further investigation is required when feature spaces are large and training is performed with spectral subclasses of the land cover classes to be mapped. This paper proposes to assess the impact of sample size in the classification accuracy of Random Forest using multitemporal Sentinel-2 data and a detailed set of training subclasses to produce a map with general land cover classes. The results revealed similar classification accuracies after major reductions in sample size.
更多查看译文
关键词
Random Forest,Sentinel-2,training sample,sample size
AI 理解论文
溯源树
样例
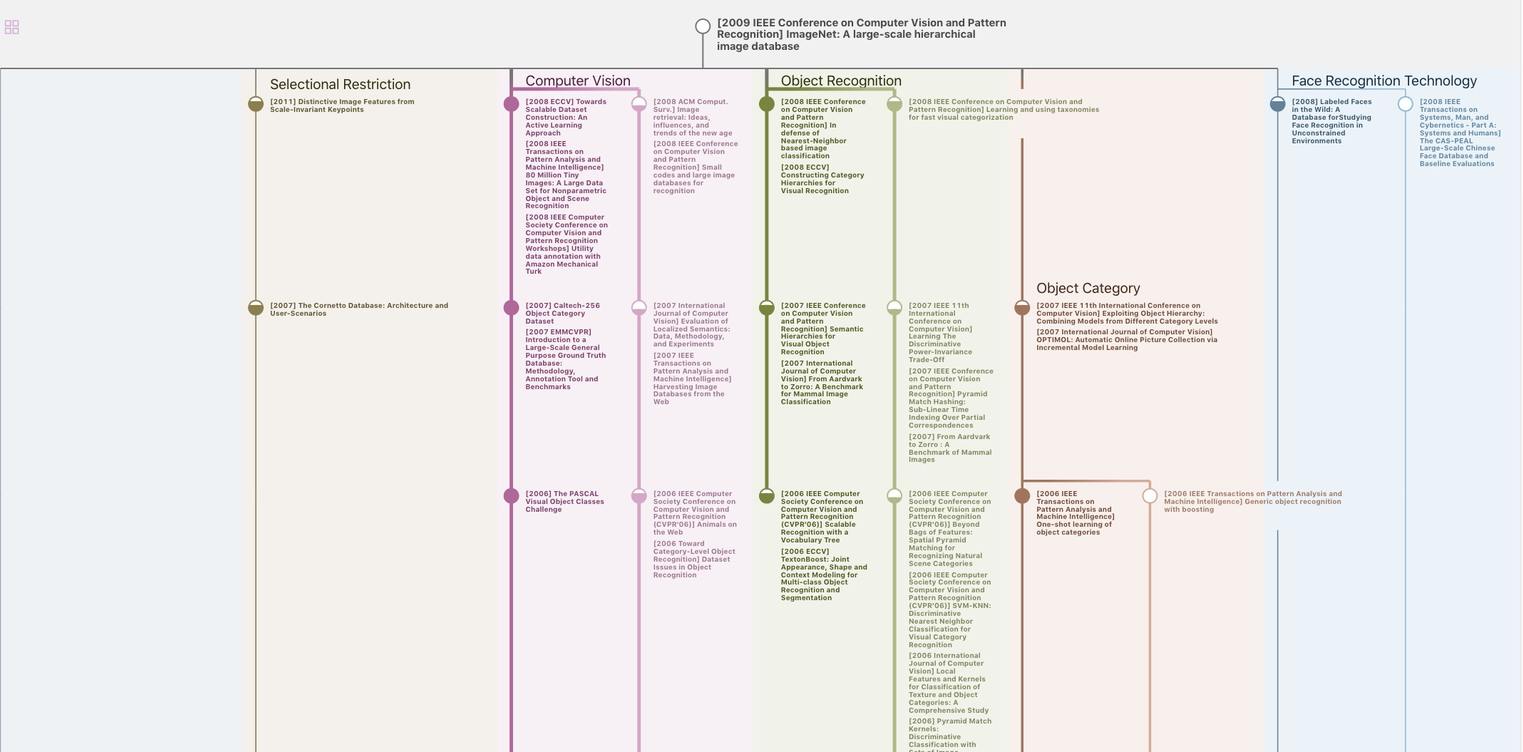
生成溯源树,研究论文发展脉络
Chat Paper
正在生成论文摘要