Segmentation of Tree Canopies in Urban Environments Using Dilated Convolutional Neural Network
IGARSS(2021)
摘要
Object detection and image segmentation are essential for environmental monitoring. This task can be performed and automated using machines with the processing capabilities of convolutional neural networks (CNN), achieving outstanding performance thanks to the current computing capacity and data available. Still, there are advances to perceive and questions on how to get the optimal performance and improve the results. With this aim, we assessed a state-of-the-art CNN, the Dynamic Dilated Convolution Neural Network (DDCN), to segment trees inside an urban environment. We chose DDCN because it exploits the paradigm of multi-context without increasing the number of trainable parameters of the network and defines, while training, the best patch size that should be used by the network in the test phase, helping to tune the CNN. With this technique, we achieved: pixel accuracy of 95.86%; average accuracy of 90.63%; F1-score of 90.93%; Kappa index of 81.87% and IoU of 72.78%. We are showing the capabilities of this CNN to segment complex images.
更多查看译文
关键词
Semantic Segmentation,Deep Learning,Remote sensing,Urban environment,Convolutional Neural Network
AI 理解论文
溯源树
样例
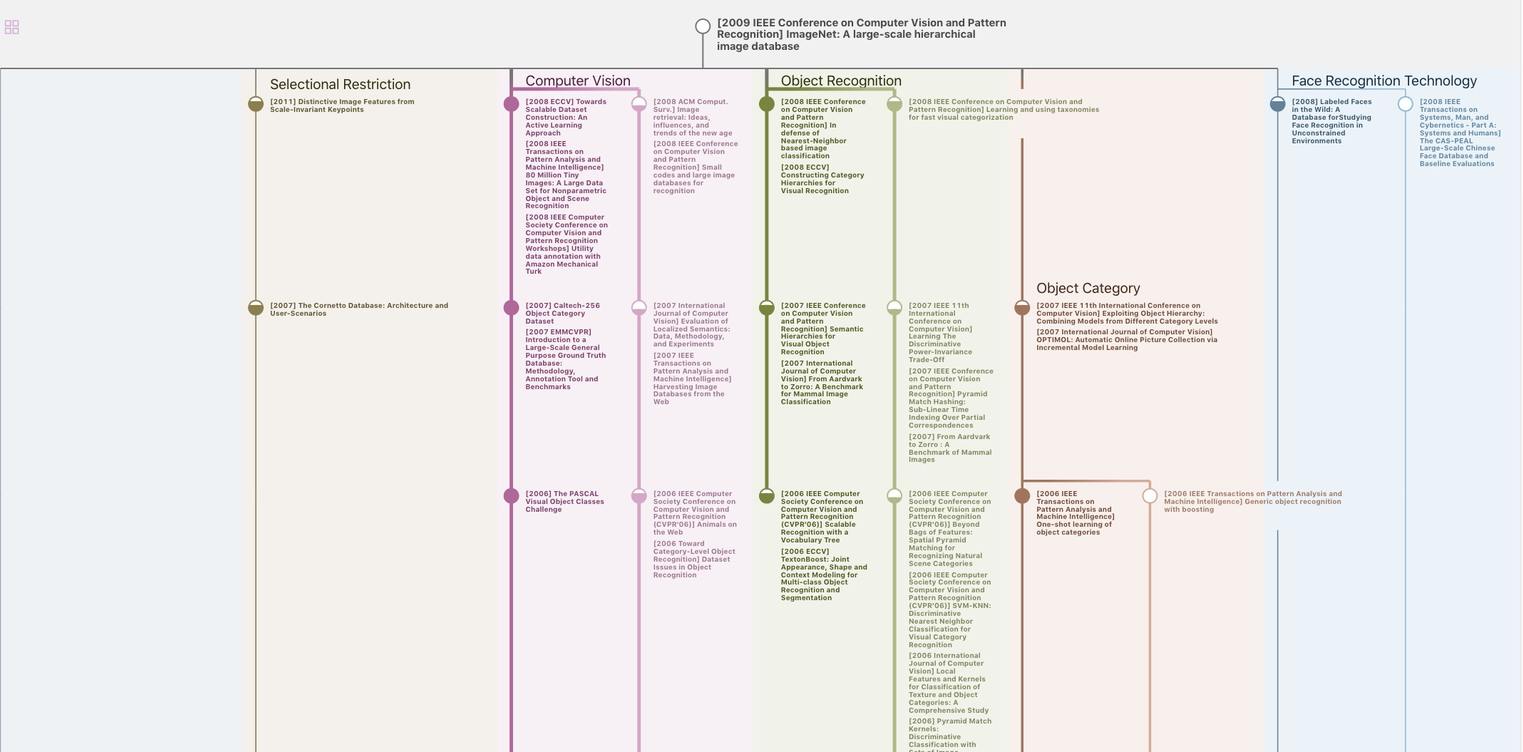
生成溯源树,研究论文发展脉络
Chat Paper
正在生成论文摘要