Adapting Kernels for Hyperspectral Image Classification.
IGARSS(2021)
摘要
Despite its great potential in a wide range of human activities, hyperspectral remote sensing imaging (HSI) exhibits several challenges that prevent full exploitation of its data. In particular, land-cover classification based on HSI data suffers significant degradation due to problematic data variability. Convolutional Neural Networks (CNNs) ability to extract spectral-spatial features has enabled the development of powerful classifiers, which achieve not yet seen accuracy results. To enhance the feature extraction procedure, this paper presents a novel HSI-CNN model (DKDCNet) which combines adaptive deforming kernels (DK) and convolutions (DC) with the aim of pinpointing the effective receptive field (ERF) on the challenging input data. Experimental results on the University of Houston benchmark show that DKDCNet is able to obtain a more accurate classification than traditional strategies with similar computational cost for HSI classification. Source code: https://github.com/mhaut/DKDCNet.
更多查看译文
关键词
Hyperspectral imaging (HSI),Classification,Convolutional Neural Network (CNN),Effective Receptive Field (ERF)
AI 理解论文
溯源树
样例
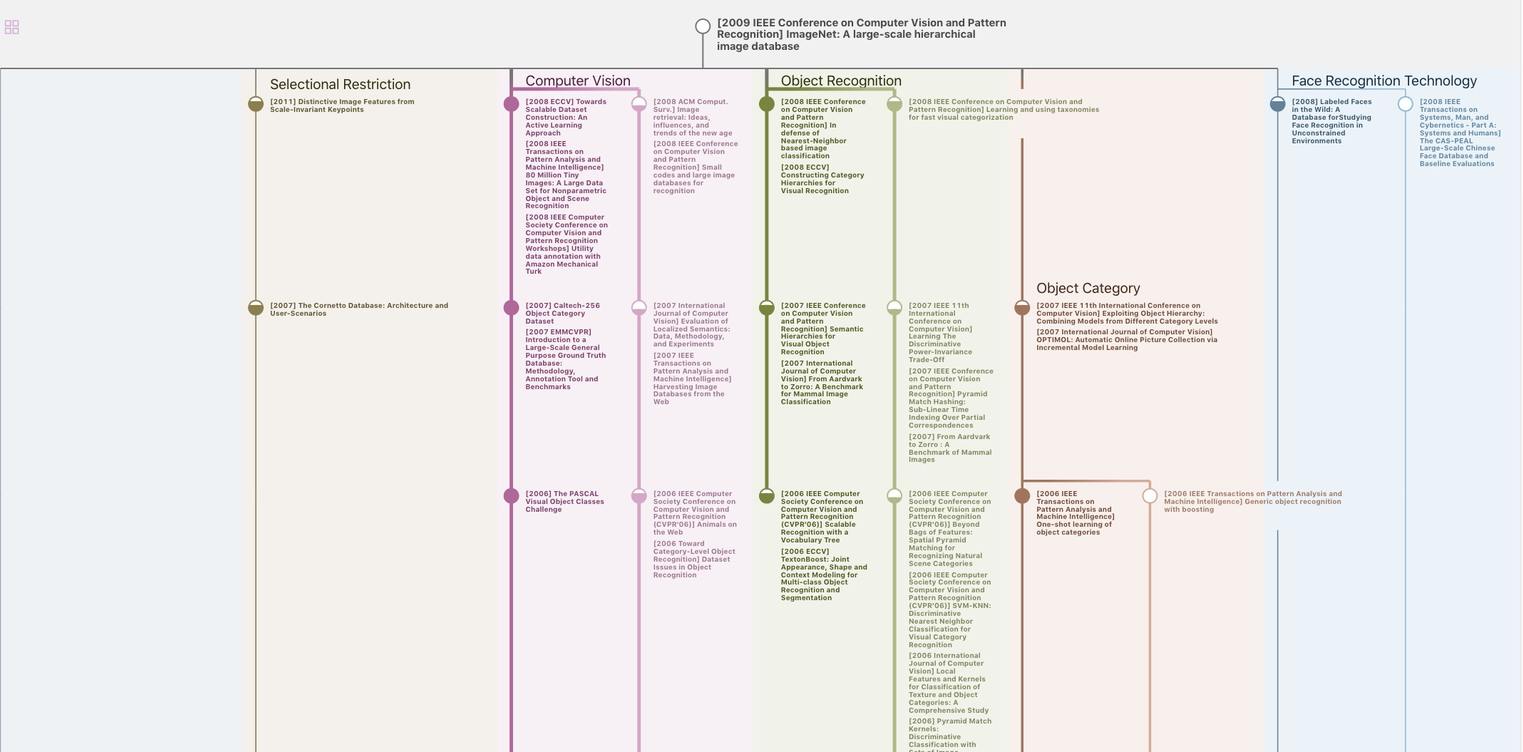
生成溯源树,研究论文发展脉络
Chat Paper
正在生成论文摘要