A Machine Learning Framework for Mapping Soil Nutrients with Multi-Source Data Fusion.
IGARSS(2021)
摘要
One of the major considerations in precision agriculture is optimizing fertilization to ensure maximum crop productivity. The principle behind this precision fertilization is to adjust the fertilizer inputs according to the properties of soils and crop stage such that crop yield is maximized and fertilizer loss is minimized. For this, one needs to understand the default soil nutrient status. This paper presents a feasible approach for developing high resolution spatial map (30m) of soil nutrients based on machine learning model with multiple sources covariates from climate, terrain, remote satellite etc. The covariate variables in different scales were chosen based on their association with soil nutrients and converted to construct field scale soil nutrients (pH, OM, EC, N, P & K) mapping models. The nutrients distribution is able to capture the spatial variability with moderate to high accuracy. This research is a methodological contribution to precision agriculture and lays the ground for precise application of fertilizers.
更多查看译文
关键词
Geo-spatial data,Remote sensing,Machine learning,Climate,Soil properties
AI 理解论文
溯源树
样例
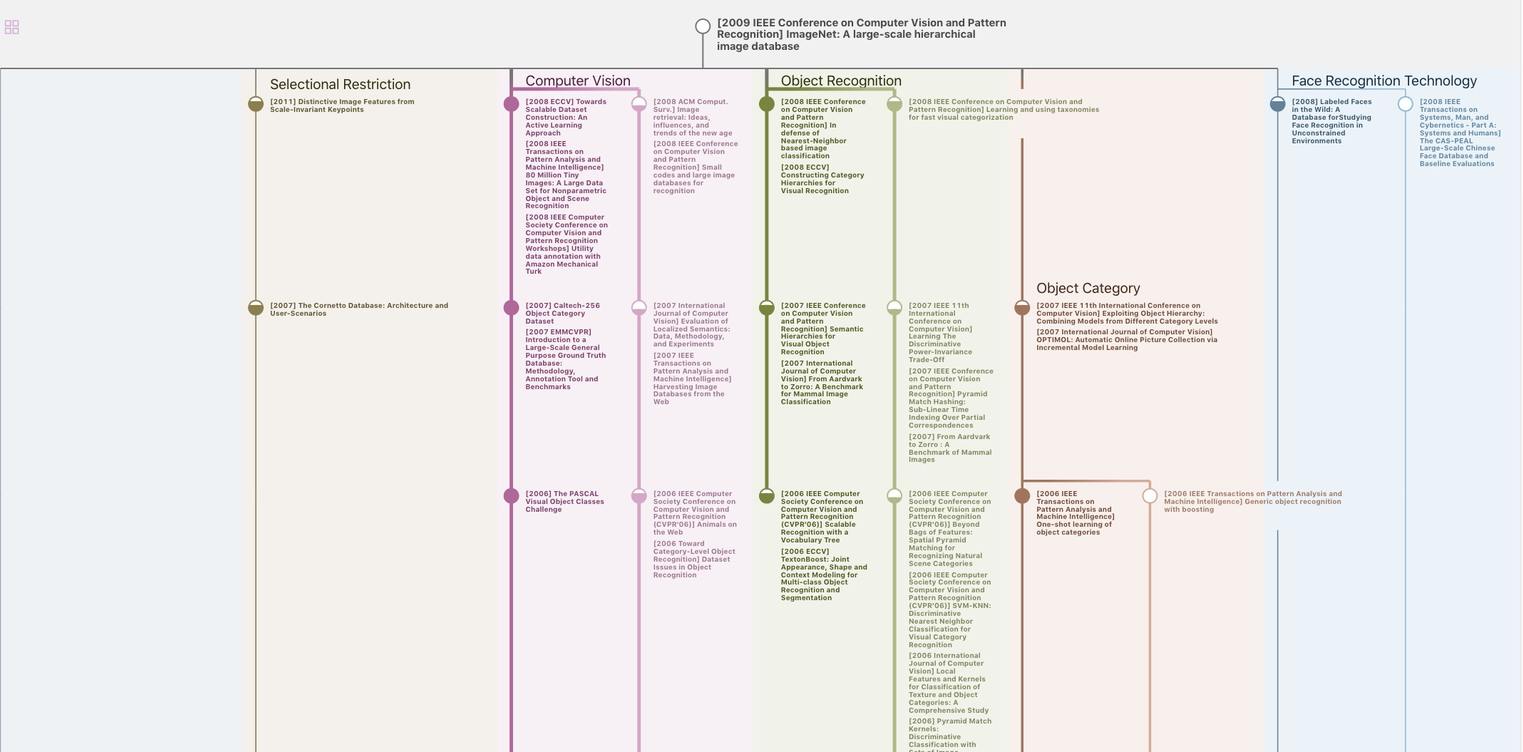
生成溯源树,研究论文发展脉络
Chat Paper
正在生成论文摘要