Testing Deep Learning-based Visual Perception for Automated Driving
ACM Transactions on Cyber-Physical Systems(2021)
摘要
AbstractDue to the impressive performance of deep neural networks (DNNs) for visual perception, there is an increased demand for their use in automated systems. However, to use deep neural networks in practice, novel approaches are needed, e.g., for testing. In this work, we focus on the question of how to test deep learning-based visual perception functions for automated driving. Classical approaches for testing are not sufficient: A purely statistical approach based on a dataset split is not enough, as testing needs to address various purposes and not only average case performance. Additionally, a complete specification is elusive due to the complexity of the perception task in the open context of automated driving. In this article, we review and discuss existing work on testing DNNs for visual perception with a special focus on automated driving for test input and test oracle generation as well as test adequacy. We conclude that testing of DNNs in this domain requires several diverse test sets. We show how such tests sets can be constructed based on the presented approaches addressing different purposes based on the presented methods and identify open research questions.
更多查看译文
关键词
Software testing, deep learning, perception, computer vision, automated driving, autonomous driving
AI 理解论文
溯源树
样例
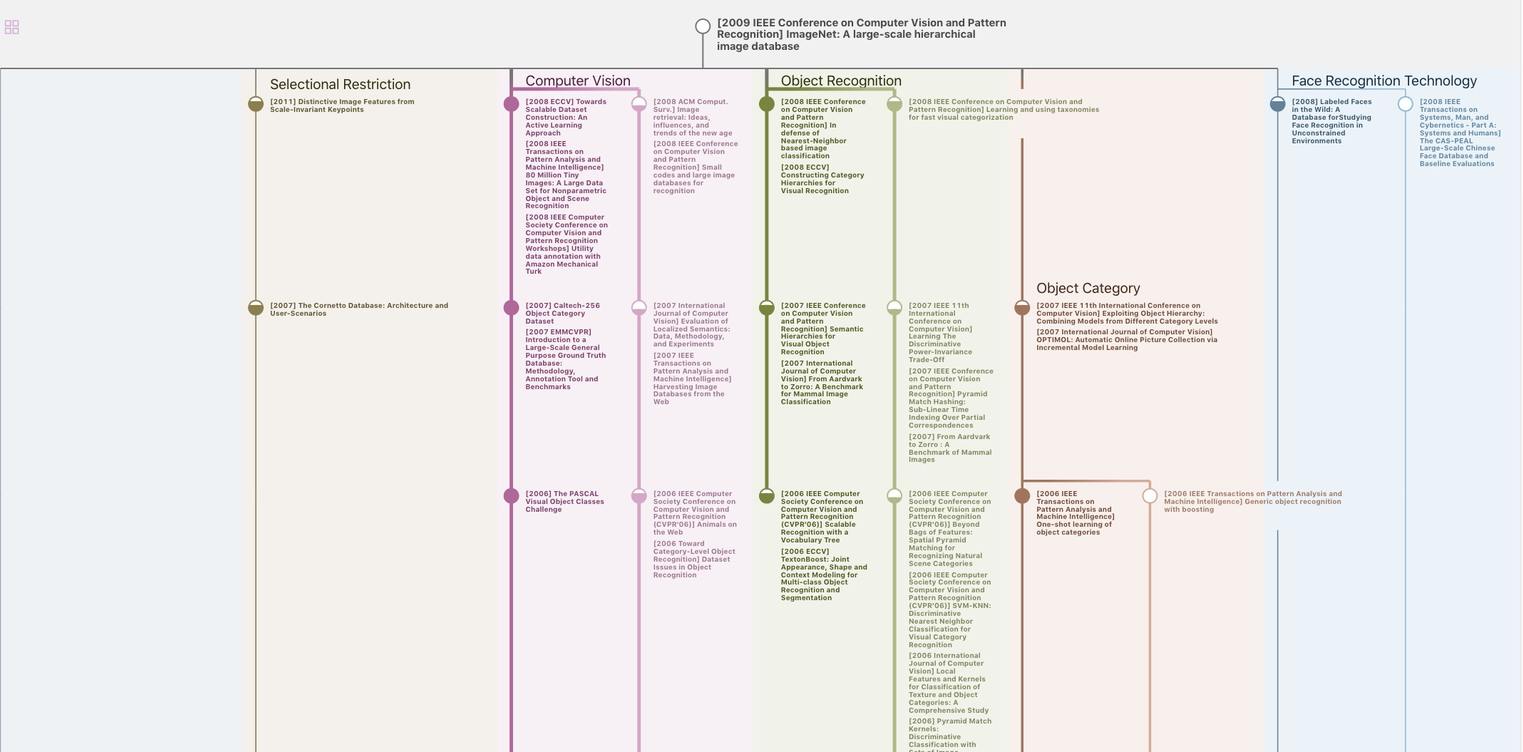
生成溯源树,研究论文发展脉络
Chat Paper
正在生成论文摘要