Smoothness-Driven Consensus Based on Compact Representation for Robust Feature Matching
IEEE transactions on neural networks and learning systems(2023)
摘要
For robust feature matching, a popular and particularly effective method is to recover smooth functions from the data to differentiate the true correspondences (inliers) from false correspondences (outliers). In the existing works, the well-established regularization theory has been extensively studied and exploited to estimate the functions while controlling its complexity to enforce the smoothness constraint, which has shown prominent advantages in this task. However, despite the theoretical optimality properties, the high complexities in both time and space are induced and become the main obstacle of their application. In this article, we propose a novel method for multivariate regression and point matching, which exploits the sparsity structure of smooth functions. Specifically, we use compact Fourier bases for constructing the function, which inherently allows a coarse-to-fine representation. The smoothness constraint can be explicitly imposed by adopting a few low-frequency bases for representation, resulting in reduced computational complexities of the induced multivariate regression algorithm. To cope with potential gross outliers, we formulate the learning problem into a Bayesian framework with latent variables indicating the inliers and outliers and a mixture model accounting for the distribution of data, where a fast expectation-maximization solution can be derived. Extensive experiments are conducted on synthetic data and real-world image matching, and point set registration datasets, which demonstrates the advantages of our method against the current state-of-the-art methods in terms of both scalability and robustness.
更多查看译文
关键词
Task analysis,Data models,Computational modeling,Multivariate regression,Strain,Robustness,Optimization,Compact representation,feature matching,mismatch removal,outlier,regularization
AI 理解论文
溯源树
样例
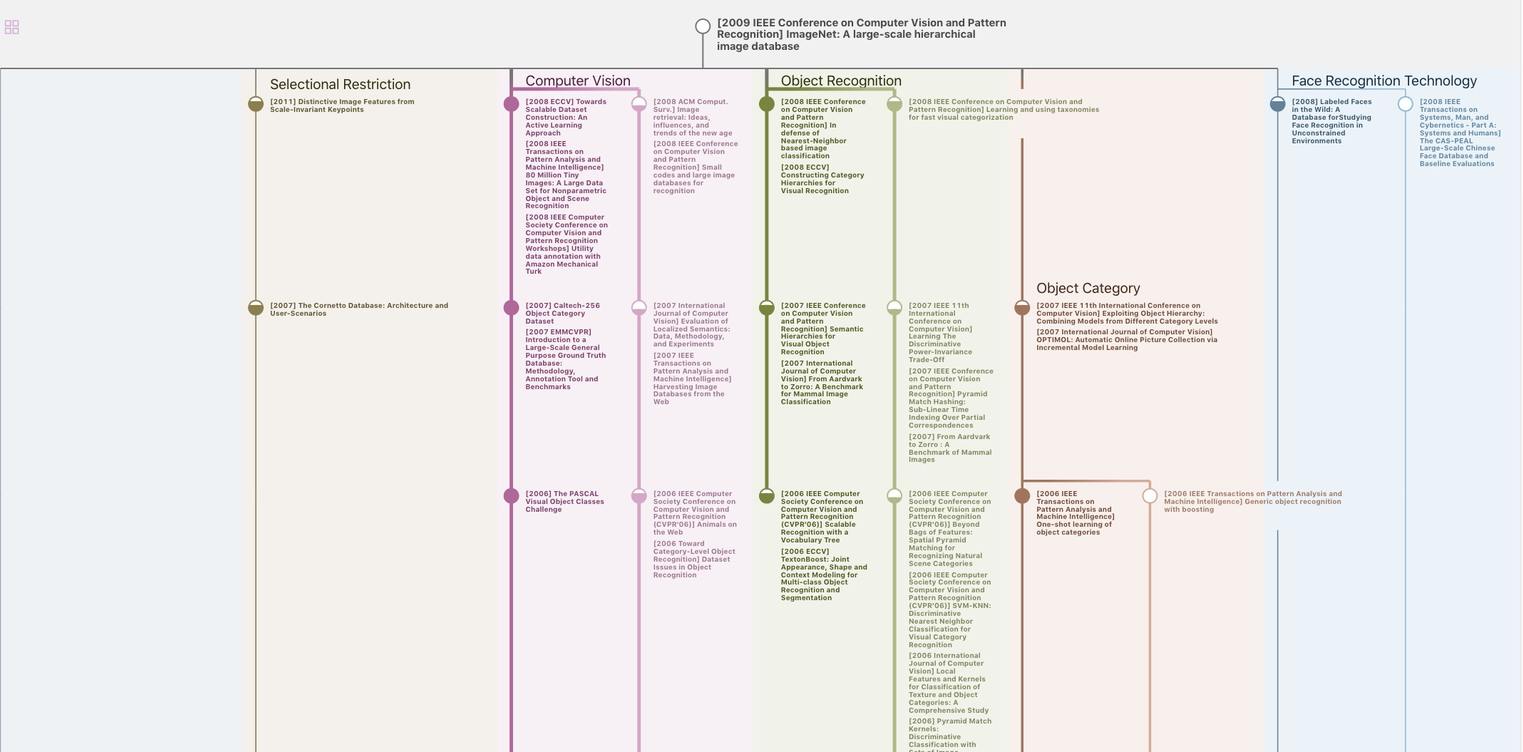
生成溯源树,研究论文发展脉络
Chat Paper
正在生成论文摘要