Activation Landscapes as a Topological Summary of Neural Network Performance.
IEEE BigData(2021)
摘要
We use topological data analysis (TDA) to study how data transforms as it passes through successive layers of a deep neural network (DNN). We compute the persistent homology of the activation data for each layer of the network and summarize this information using persistence landscapes. The resulting feature map provides both an informative visual- ization of the network and a kernel for statistical analysis and machine learning. We observe that the topological complexity often increases with training and that the topological complexity does not decrease with each layer.
更多查看译文
关键词
Topological data analysis,persistent homology,persistence landscapes,deep neural networks,topological complexity,local homology
AI 理解论文
溯源树
样例
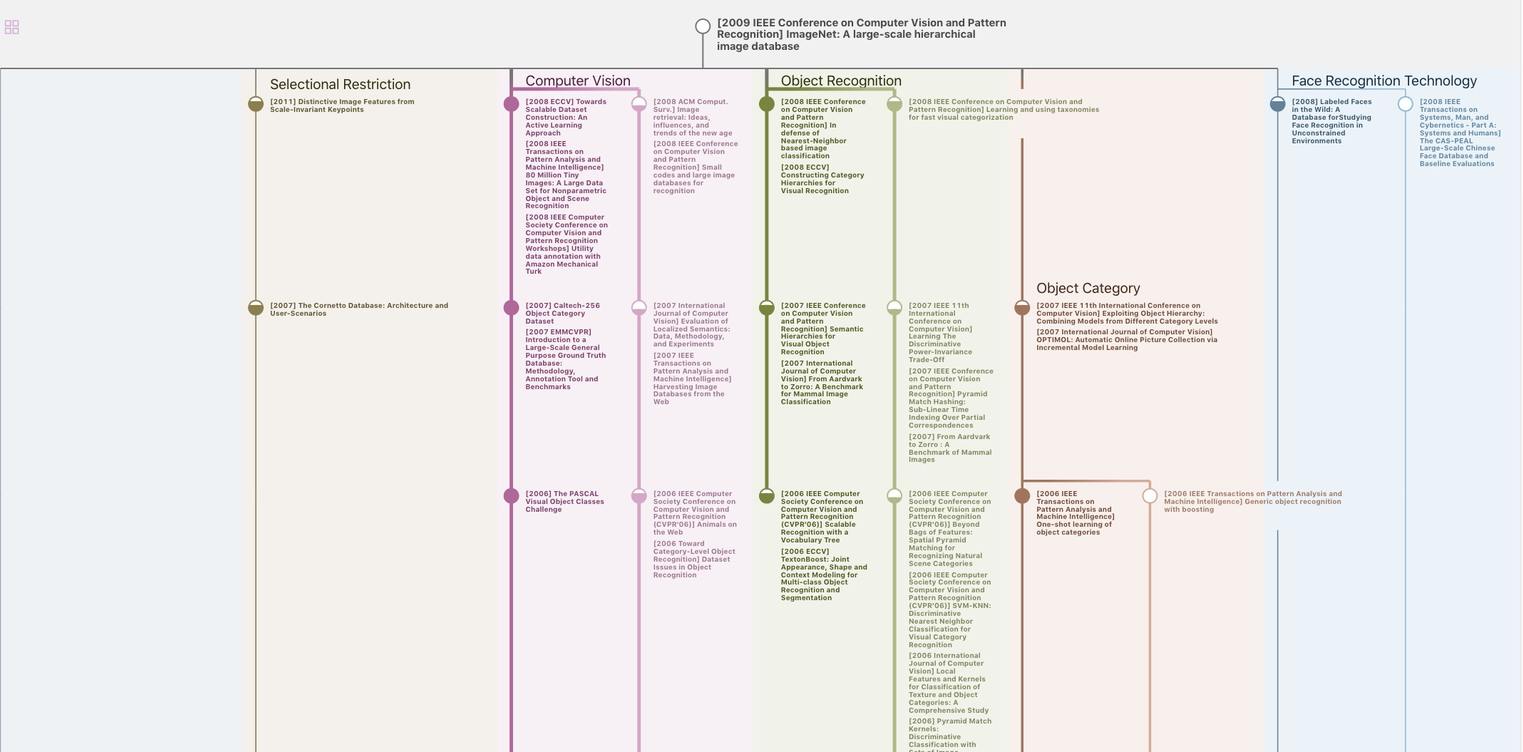
生成溯源树,研究论文发展脉络
Chat Paper
正在生成论文摘要