Robust Event Classification Using Imperfect Real-World PMU Data
arxiv(2023)
摘要
This article studies robust event classification using imperfect real-world phasor measurement unit (PMU) data. By analyzing the real-world PMU data, we find that it is challenging to directly use this data set for event classifiers due to the low data quality observed in PMU measurements and event logs. To address these challenges, we develop a novel machine learning framework for training robust event classifiers, which consists of three main steps: 1) data preprocessing; 2) fine-grained event data extraction; and 3) feature engineering. Specifically, the data preprocessing step addresses the data quality issues of PMU measurements (e.g., bad data and missing data); in the fine-grained event data extraction step, a model-free event detection method is developed to accurately localize the events from the inaccurate event timestamps in the event logs; and the feature engineering step constructs the event features based on the patterns of different event types, in order to improve the performance and the interpretability of the event classifiers. Based on the proposed framework, we develop a workflow for event classification using the real-world PMU data streaming into the system in real time. Using the proposed framework, robust event classifiers can be efficiently trained based on many off-the-shelf lightweight machine learning models. Numerical experiments using the real-world data set from the Western Interconnection of the U.S. power transmission grid show that the event classifiers trained under the proposed framework can achieve high classification accuracy while being robust against low-quality data.
更多查看译文
关键词
Phasor measurement units,Machine learning,Feature extraction,Neural networks,Training,Data models,Frequency measurement,Event classification,event detection,feature engineering,phasor measurement units (PMUs)
AI 理解论文
溯源树
样例
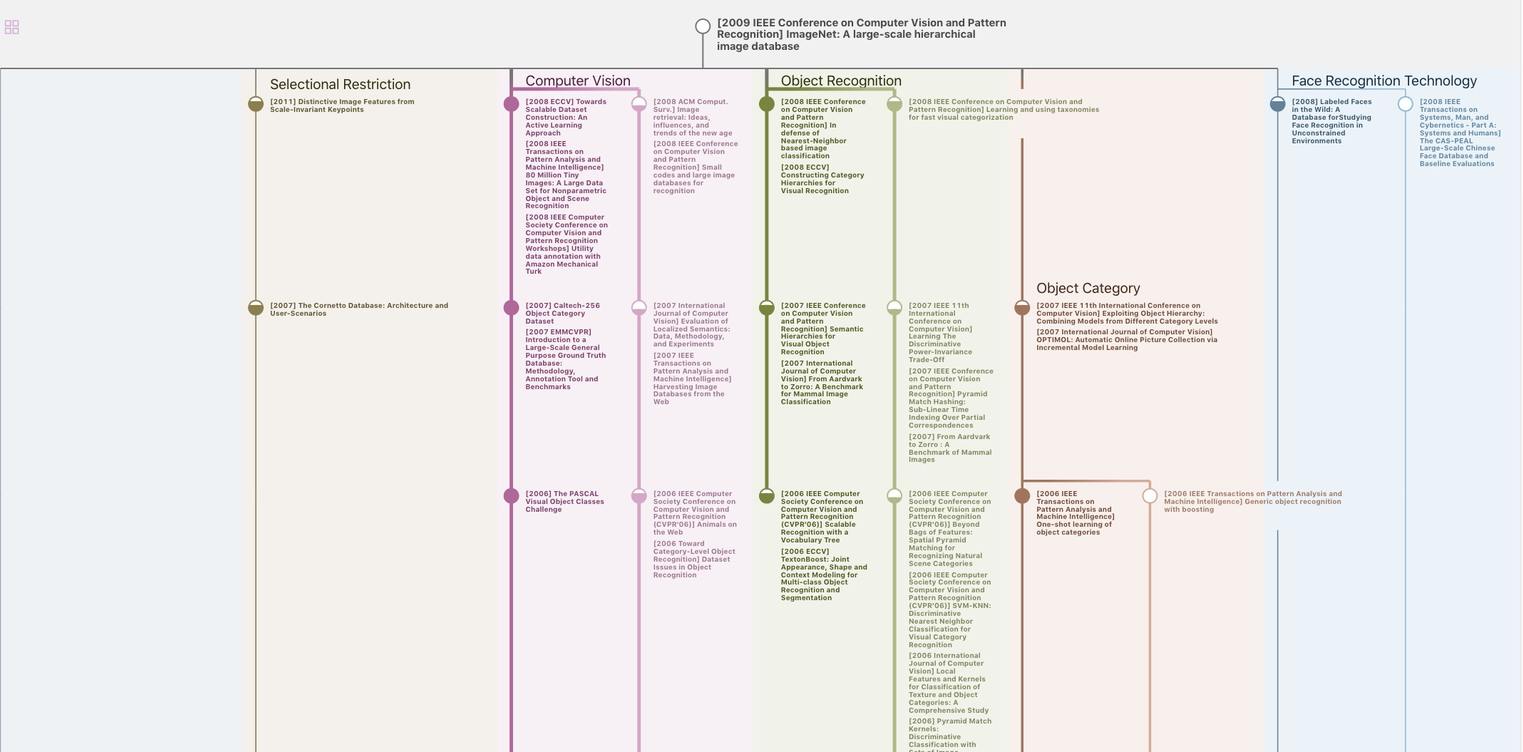
生成溯源树,研究论文发展脉络
Chat Paper
正在生成论文摘要