Inductive Biases and Variable Creation in Self-Attention Mechanisms.
International Conference on Machine Learning(2022)
摘要
Self-attention, an architectural motif designed to model long-range interactions in sequential data, has driven numerous recent breakthroughs in natural language processing and beyond. This work provides a theoretical analysis of the inductive biases of self-attention modules. Our focus is to rigorously establish which functions and long-range dependencies self-attention blocks prefer to represent. Our main result shows that bounded-norm Transformer networks "create sparse variables": a single self-attention head can represent a sparse function of the input sequence, with sample complexity scaling only logarithmically with the context length. To support our analysis, we present synthetic experiments to probe the sample complexity of learning sparse Boolean functions with Transformers.
更多查看译文
关键词
mechanisms,self-attention
AI 理解论文
溯源树
样例
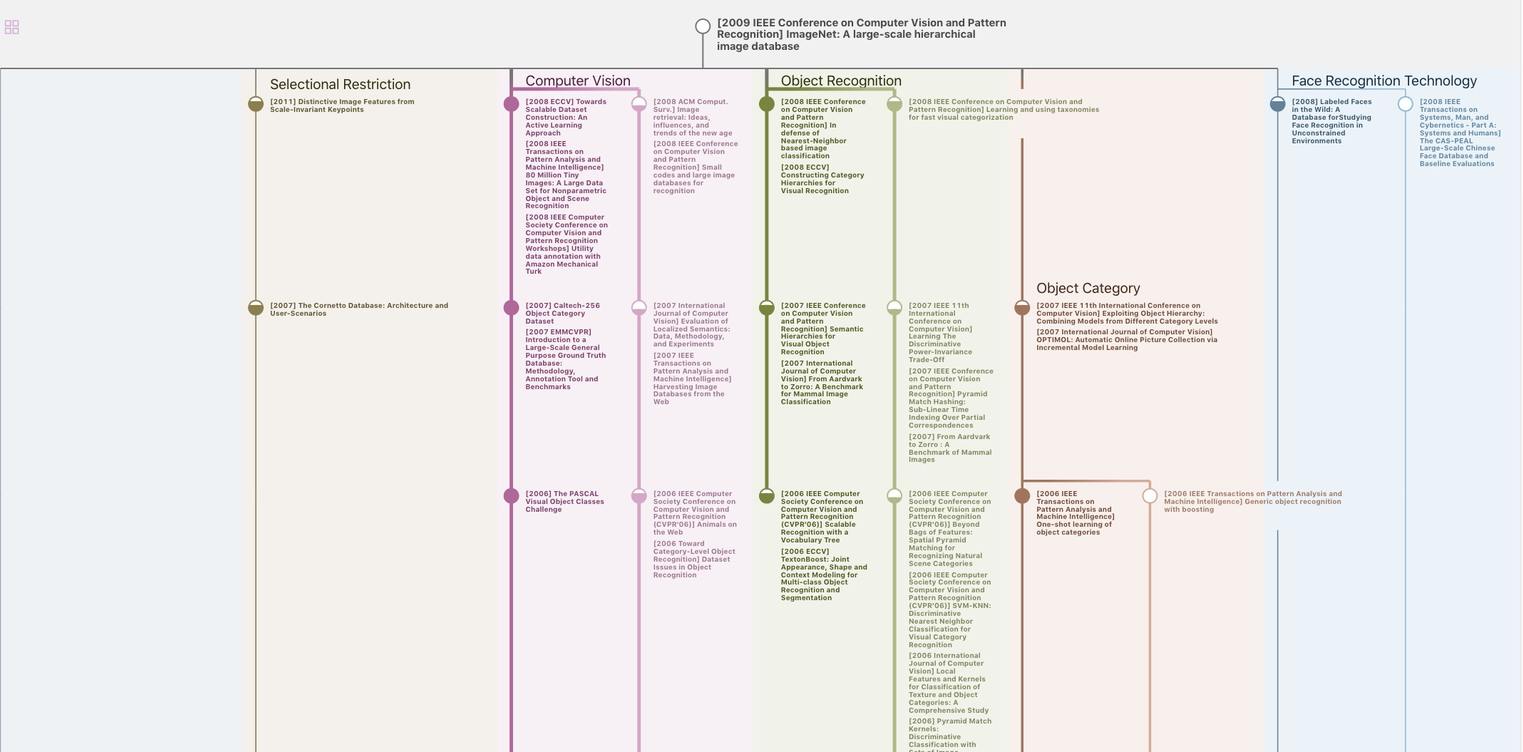
生成溯源树,研究论文发展脉络
Chat Paper
正在生成论文摘要