Epanechnikov Kernel For Pdf Estimation Applied To Equalization And Blind Source Separation
SIGNAL PROCESSING(2021)
摘要
Information Theoretic Learning (ITL) methods have been applied in a variety of applications as dynamic modeling, equalization and blind source separation. Usually, such methods involve the estimation of the probability density function (pdf) of the signals, what can be achieved through the use of kernel density estimators (KDE). In literature, the Gaussian kernel is the most largely used function in the estimator. However, using a second-order approximation, it is possible to show that the Epanechnikov kernel leads to an improved pdf estimation when compared to that using the Gaussian kernel. Hence, in this work, we explore the use of the Epanechnikov kernel in KDE applied to equalization and blind source separation problems. Following an ITL perspective, we develop methods based on the Epanechnikov kernel for both problems, showing how the proposed approach may improve performance when compared to methods in the literature which use the Gaussian kernel. (c) 2021 Elsevier B.V. All rights reserved.
更多查看译文
关键词
Information theoretic learning, Kernel density estimation, Epanechnikov kernel, Unsupervised equalization, Blind source separation
AI 理解论文
溯源树
样例
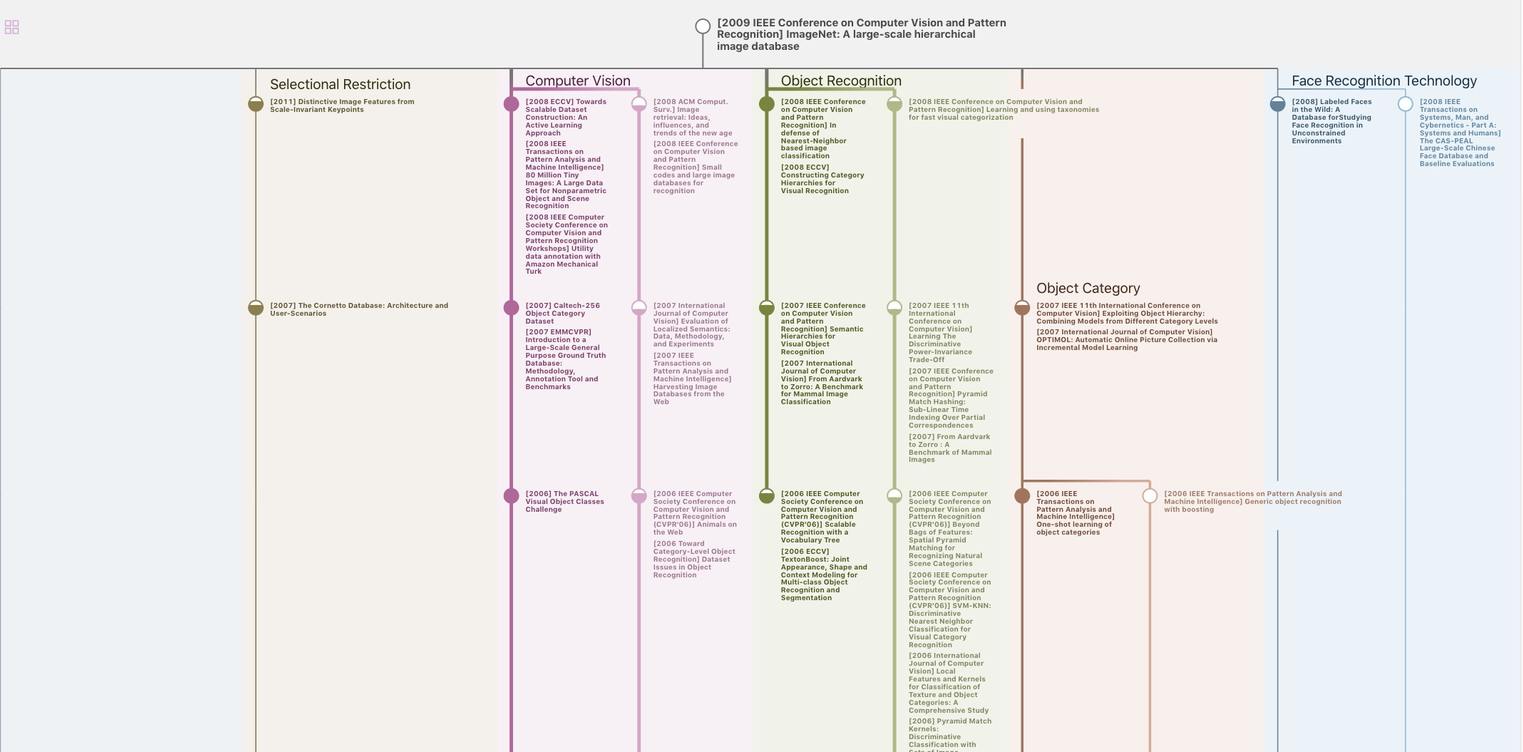
生成溯源树,研究论文发展脉络
Chat Paper
正在生成论文摘要