Mesh Convolutional Autoencoder for Semi-Regular Meshes of Different Sizes
2022 IEEE WINTER CONFERENCE ON APPLICATIONS OF COMPUTER VISION (WACV 2022)(2022)
摘要
The analysis of deforming 3D surface meshes is accelerated by autoencoders since the low-dimensional embeddings can be used to visualize underlying dynamics. But, state-of-the-art mesh convolutional autoencoders require a fixed connectivity of all input meshes handled by the autoencoder. This is due to either the use of spectral convolutional layers or mesh dependent pooling operations. Therefore, the types of datasets that one can study are limited and the learned knowledge cannot be transferred to other datasets that exhibit similar behavior. To address this, we transform the discretization of the surfaces to semi-regular meshes that have a locally regular connectivity and whose meshing is hierarchical. This allows us to apply the same spatial convolutional filters to the local neighborhoods and to define a pooling operator that can be applied to every semi-regular mesh. We apply the same mesh autoencoder to different datasets and our reconstruction error is more than 50% lower than the error from state-of-the-art models, which have to be trained for every mesh separately. Additionally, we visualize the underlying dynamics of unseen mesh sequences with an autoencoder trained on different classes of meshes.
更多查看译文
关键词
mesh convolutional autoencoder,sizes,semi-regular
AI 理解论文
溯源树
样例
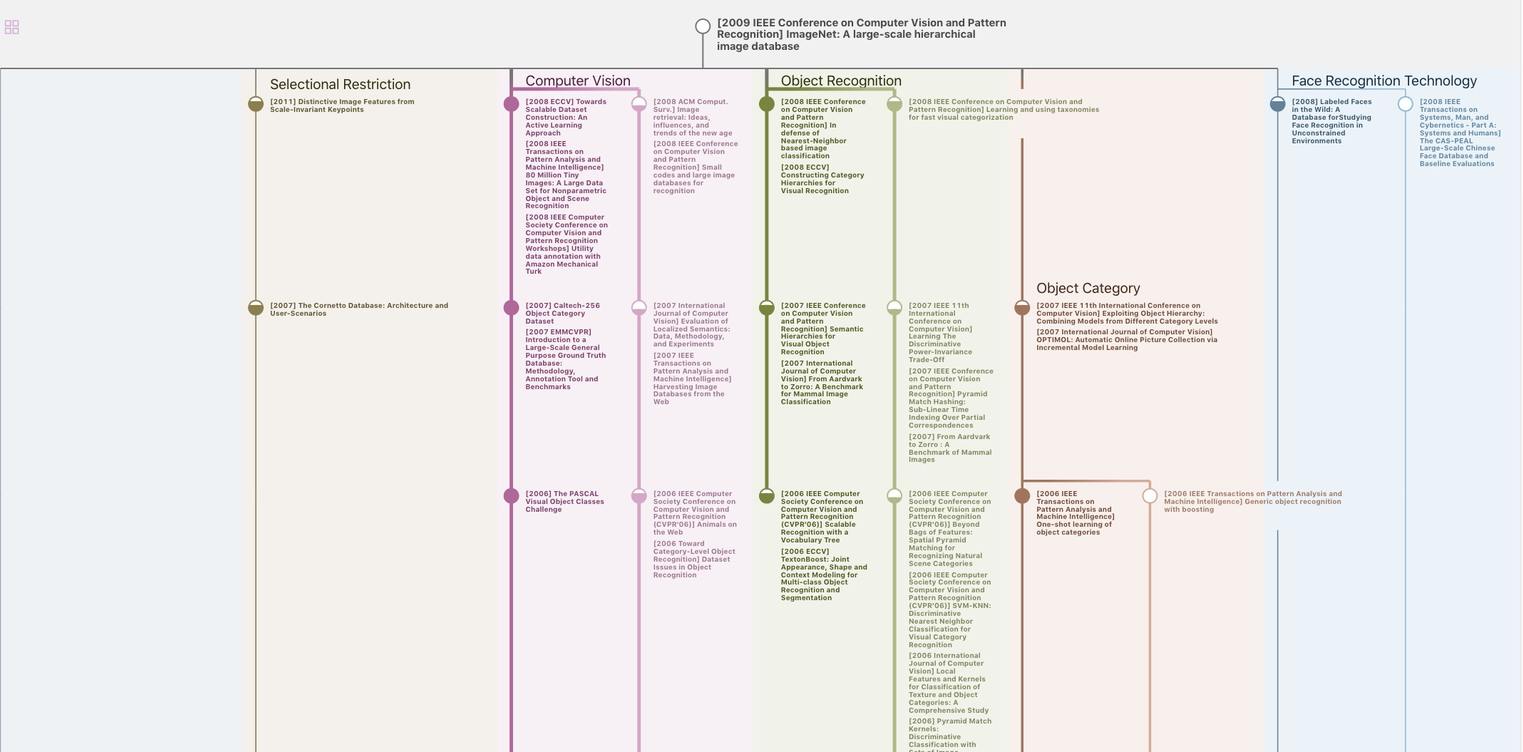
生成溯源树,研究论文发展脉络
Chat Paper
正在生成论文摘要