Real Additive Margin Softmax for Speaker Verification
IEEE International Conference on Acoustics, Speech, and Signal Processing (ICASSP)(2022)
摘要
The additive margin softmax (AM-Softmax) loss has delivered remarkable performance in speaker verification. A supposed behavior of AM-Softmax is that it can shrink within-class variation by putting emphasis on target logits, which in turn improves margin between target and non-target classes. In this paper, we conduct a careful analysis on the behavior of AM-Softmax loss, and show that this loss does not implement real max-margin training. Based on this observation, we present a Real AM-Softmax loss which involves a true margin function in the softmax training. Experiments conducted on VoxCeleb1, SITW and CNCeleb demonstrated that the corrected AM-Softmax loss consistently outperforms the original one. The code has been released at https://gitlab.com/csltstu/sunine.
更多查看译文
关键词
speaker verification,additive margin softmax,max-margin metric learning
AI 理解论文
溯源树
样例
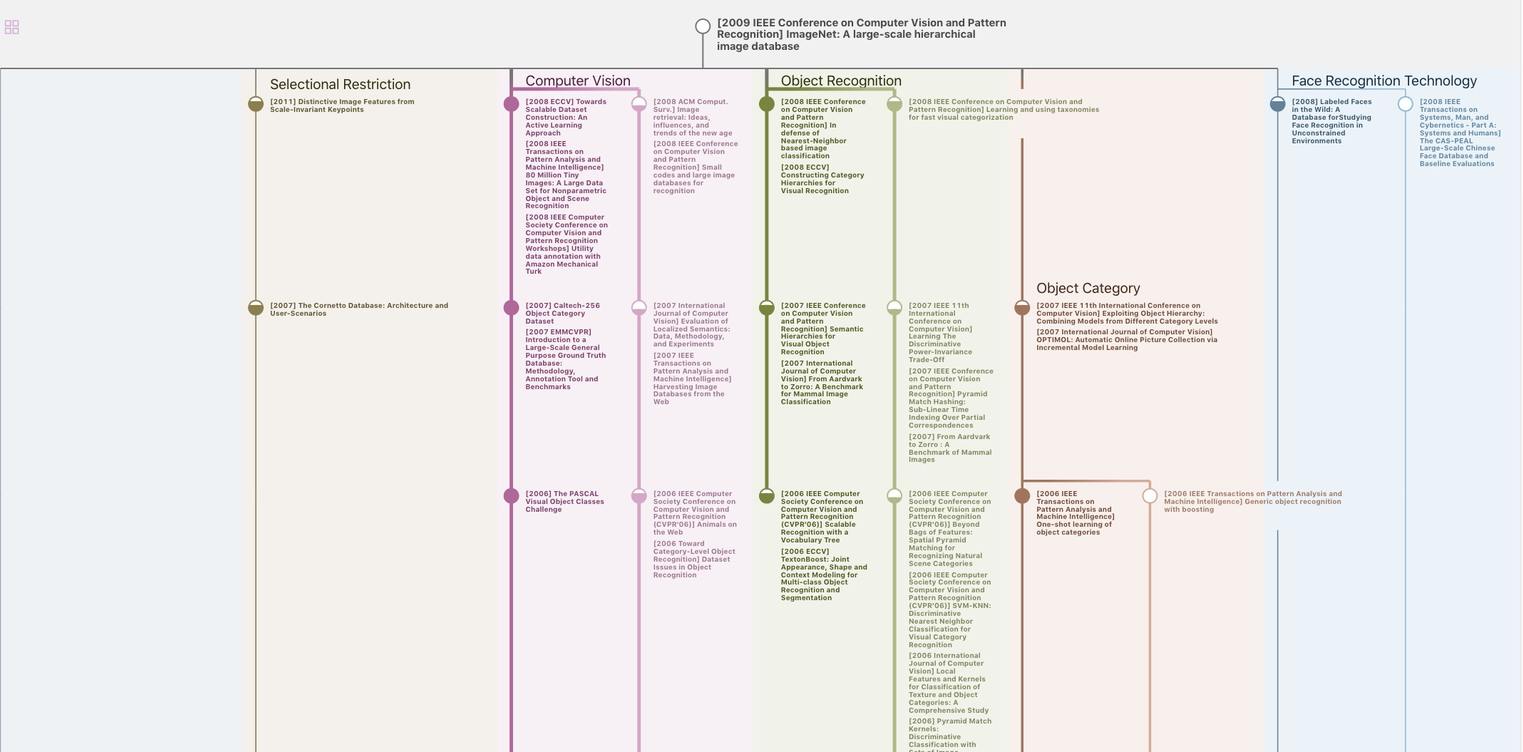
生成溯源树,研究论文发展脉络
Chat Paper
正在生成论文摘要